Fast Submodular Function Maximization
CoRR(2023)
摘要
Submodular functions have many real-world applications, such as document summarization, sensor placement, and image segmentation. For all these applications, the key building block is how to compute the maximum value of a submodular function efficiently. We consider both the online and offline versions of the problem: in each iteration, the data set changes incrementally or is not changed, and a user can issue a query to maximize the function on a given subset of the data. The user can be malicious, issuing queries based on previous query results to break the competitive ratio for the online algorithm. Today, the best-known algorithm for online submodular function maximization has a running time of $O(n k d^2)$ where $n$ is the total number of elements, $d$ is the feature dimension and $k$ is the number of elements to be selected. We propose a new method based on a novel search tree data structure. Our algorithm only takes $\widetilde{O}(nk + kd^2 + nd)$ time.
更多查看译文
关键词
function
AI 理解论文
溯源树
样例
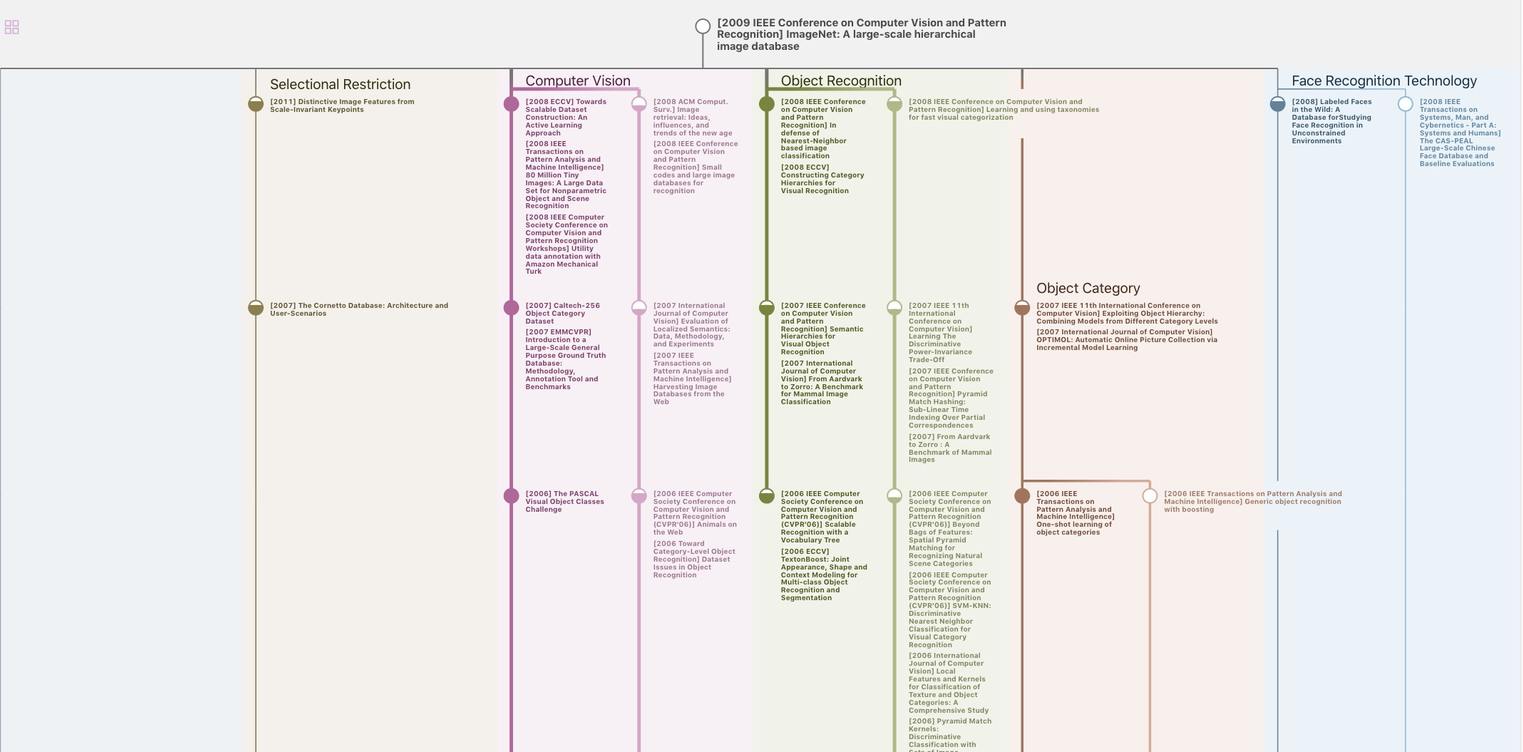
生成溯源树,研究论文发展脉络
Chat Paper
正在生成论文摘要