A Surprisingly Simple Continuous-Action POMDP Solver: Lazy Cross-Entropy Search Over Policy Trees
AAAI 2024(2024)
摘要
The Partially Observable Markov Decision Process (POMDP) provides a principled framework for decision making in stochastic partially observable environments. However, computing good solutions for problems with continuous action spaces remains challenging. To ease this challenge, we propose a simple online POMDP solver, called Lazy Cross-Entropy Search Over Policy Trees (LCEOPT). At each planning step, our method uses a novel lazy Cross-Entropy method to search the space of policy trees, which provide a simple policy representation. Specifically, we maintain a distribution on promising finite-horizon policy trees. The distribution is iteratively updated by sampling policies, evaluating them via Monte Carlo simulation, and refitting them to the top-performing ones. Our method is lazy in the sense that it exploits the policy tree representation to avoid redundant computations in policy sampling, evaluation, and distribution update. This leads to computational savings of up to two orders of magnitude. Our LCEOPT is surprisingly simple as compared to existing state-of-the-art methods, yet empirically outperforms them on several continuous-action POMDP problems, particularly for problems with higher-dimensional action spaces.
更多查看译文
关键词
PRS: Planning with Markov Models (MDPs, POMDPs),RU: Sequential Decision Making,RU: Stochastic Optimization
AI 理解论文
溯源树
样例
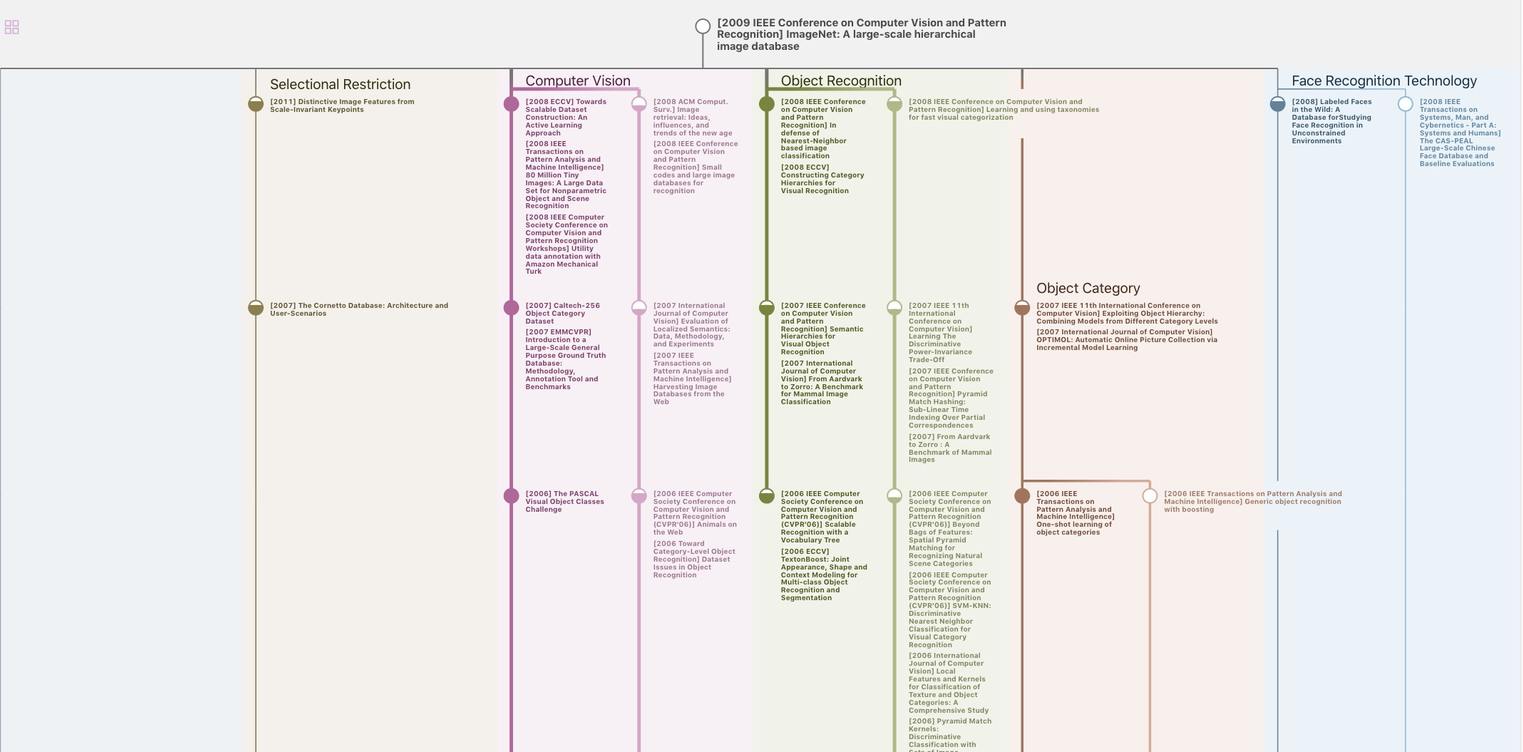
生成溯源树,研究论文发展脉络
Chat Paper
正在生成论文摘要