Small-data Reduced Order Modeling of Chaotic Dynamics through SyCo-AE: Synthetically Constrained Autoencoders
CoRR(2023)
摘要
Data-driven reduced order modeling of chaotic dynamics can result in systems that either dissipate or diverge catastrophically. Leveraging non-linear dimensionality reduction of autoencoders and the freedom of non-linear operator inference with neural-networks, we aim to solve this problem by imposing a synthetic constraint in the reduced order space. The synthetic constraint allows our reduced order model both the freedom to remain fully non-linear and highly unstable while preventing divergence. We illustrate the methodology with the classical 40-variable Lorenz '96 equations, showing that our methodology is capable of producing medium-to-long range forecasts with lower error using less data.
更多查看译文
关键词
chaotic dynamics,small-data
AI 理解论文
溯源树
样例
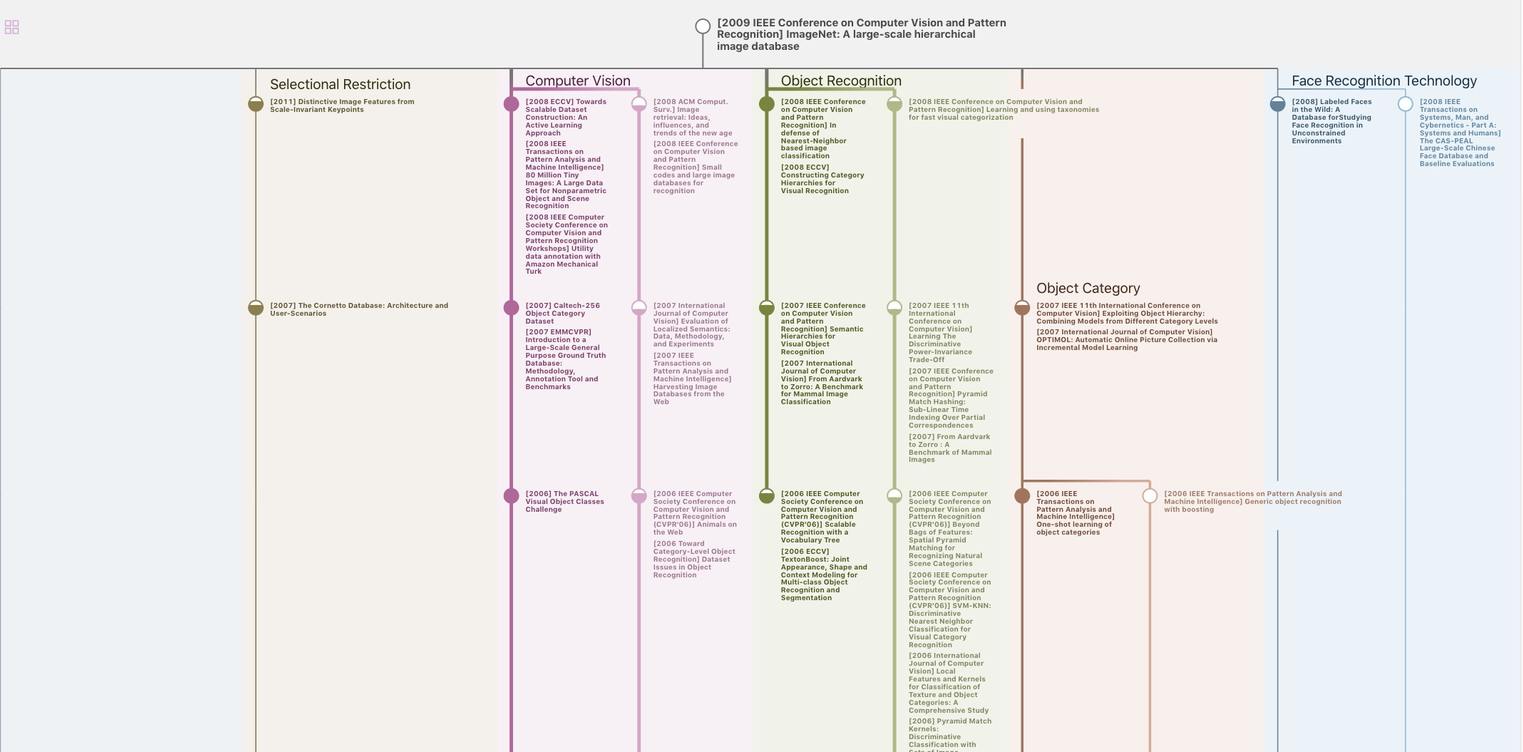
生成溯源树,研究论文发展脉络
Chat Paper
正在生成论文摘要