Thompson Sampling for Parameterized Markov Decision Processes with Uninformative Actions
CoRR(2023)
摘要
We study parameterized MDPs (PMDPs) in which the key parameters of interest are unknown and must be learned using Bayesian inference. One key defining feature of such models is the presence of "uninformative" actions that provide no information about the unknown parameters. We contribute a set of assumptions for PMDPs under which Thompson sampling guarantees an asymptotically optimal expected regret bound of $O(T^{-1})$, which are easily verified for many classes of problems such as queuing, inventory control, and dynamic pricing.
更多查看译文
关键词
parameterized markov decision processes,uninformative actions
AI 理解论文
溯源树
样例
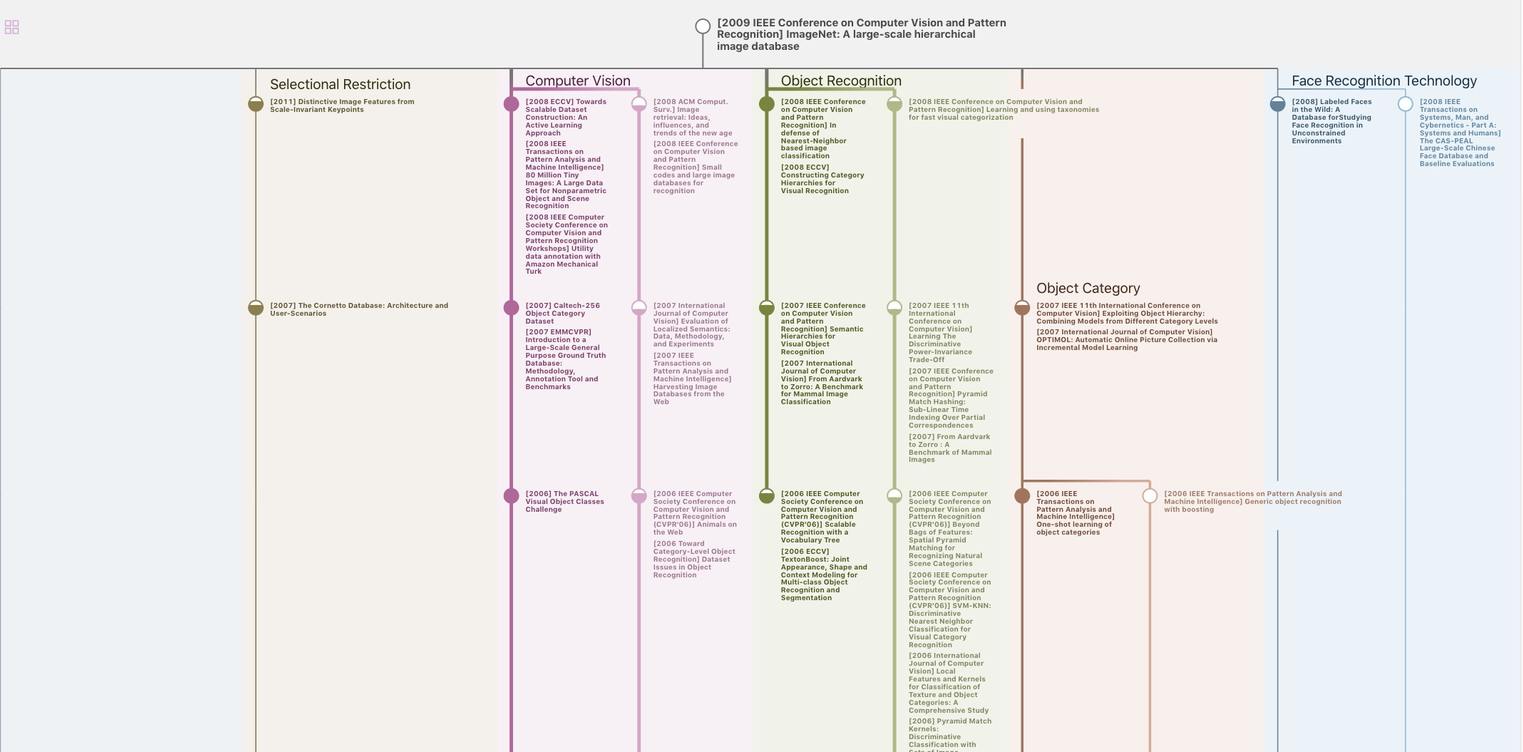
生成溯源树,研究论文发展脉络
Chat Paper
正在生成论文摘要