Representation of Semantic Word Embeddings Based on SLDA and Word2vec Model
Chinese Journal of Electronics(2023)
摘要
To solve the problem of semantic loss in text representation, this paper proposes a new embedding method of word representation in semantic space called wt2svec based on supervised latent Dirichlet allocation (SLDA) and Word2vec. It generates the global topic embedding word vector utilizing SLDA which can discover the global semantic information through the latent topics on the whole document set. It gets the local semantic embedding word vector based on the Word2vec. The new semantic word vector is obtained by combining the global semantic information with the local semantic information. Additionally, the document semantic vector named doc2svec is generated. The experimental results on different datasets show that wt2svec model can obviously promote the accuracy of the semantic similarity of words, and improve the performance of text categorization compared with Word2vec.
更多查看译文
关键词
Supervised latent Dirichlet allocation,Semantic word vector,Word2vec,Word embedding,Semantic similarity,Text categorization
AI 理解论文
溯源树
样例
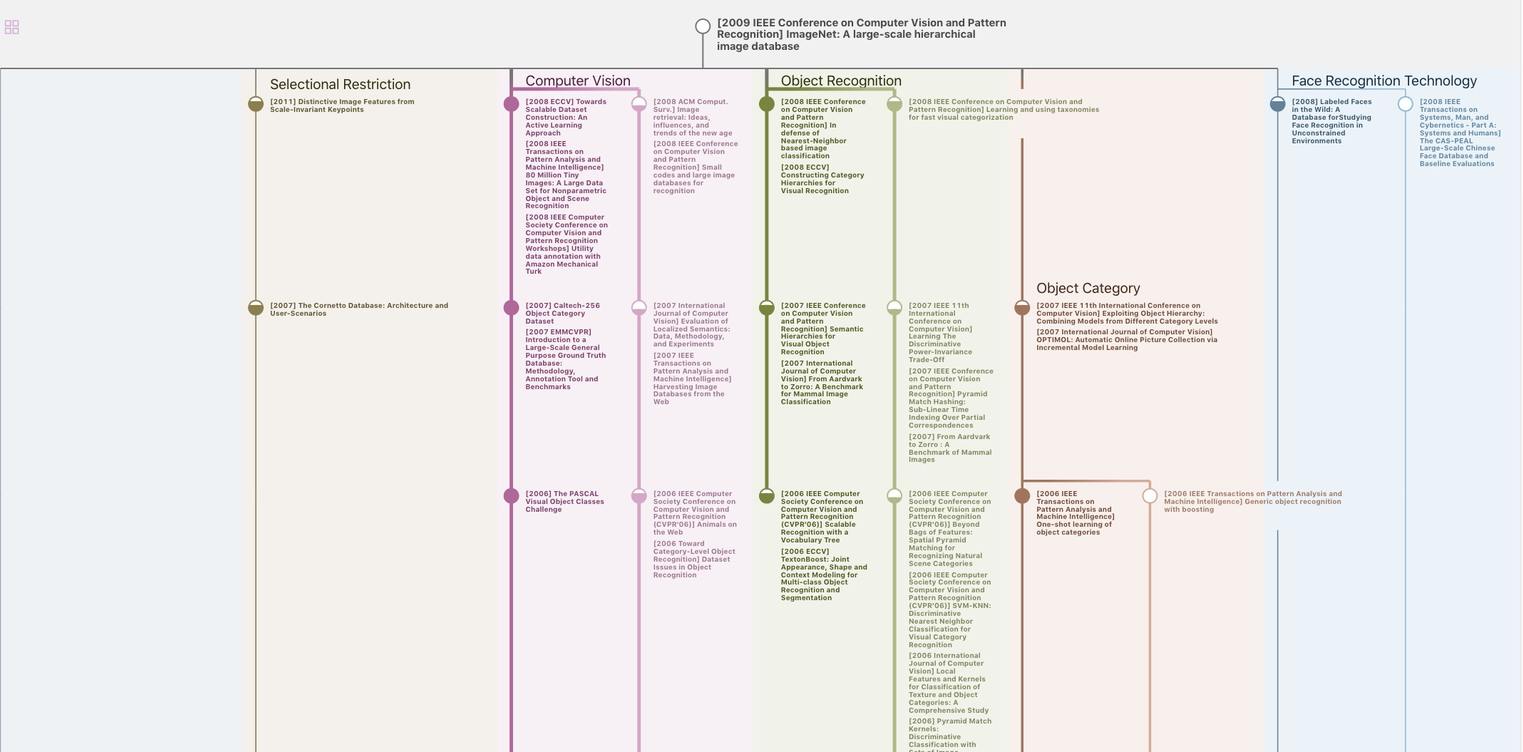
生成溯源树,研究论文发展脉络
Chat Paper
正在生成论文摘要