Utilizing Alignment Loss to Advance Eye Center Detection and Face Recognition in the LWIR Band
IEEE Transactions on Biometrics, Behavior, and Identity Science(2023)
摘要
Geometric normalization is an integral part of most of the face recognition (FR) systems. To geometrically normalize a face, it is essential to detect the eye centers, since one way to align the face images is to make the line joining the eye centers horizontal. This paper proposes a novel approach to detect eye centers in the challenging Long-Wave Infrared (LWIR) spectrum (8-
$14 ~\mu \text{m}$
). While using thermal band images for face recognition is a feasible approach in low-light and nighttime conditions, where visible face images cannot be used, there are not many thermal or dual band (visible and thermal) face datasets available to train and test new eye center detection models. This work takes advantage of the available deep learning based eye center detection algorithms in the visible band to detect the eye centers in thermal face images through image synthesis. While we empirically evaluate different image synthesis models, we determine that StarGAN2 yields the highest eye center detection accuracy, when compared to the other state-of-the-art models. We incorporate alignment loss that captures the normalized error between the detected and actual eye centers as an additional loss term during training (using the generated images during training, ground truth annotations, and an eye center detection model), so that the model learns to align the images to minimize this error. During test phase, visible images are generated from the thermal images using the trained model. Then, the available landmark detection algorithms in the visible band, namely, MT-CNN and HR-Net are used to detect the eye centers. Next, these eye centers are used to geometrically normalize the source thermal face images before performing same-spectral (thermal-to-thermal) face recognition. The proposed method improved the eye center detection accuracy by 60% over the baseline model, and by 14% over training only the StarGAN2 model without the alignment loss. The proposed approach also reports the highest improvement in the face recognition accuracy by 36% and 3% over the baseline and original StarGAN2 models, respectively, when using deep learning based face recognition models, namely, Facenet, ArcFace, and VGG-Face. We also perform experiments by augmenting the train and test datasets with images rotated in-plane to further demonstrate the efficiency of the proposed approach. When CycleGAN (another unpaired image translation network) is used to generate images before incorporating the alignment loss, it failed to preserve the alignment at the slightest, therefore the eye center detection accuracy was extremely low. With the alignment loss, the accuracy increased by 20%, 50%, and 80% when the normalized error (e)
$\le0.05$
, 0.10 and 0.25 respectively.
更多查看译文
关键词
Biometrics,face recognition,thermal imaging,long wave infrared,geometric normalization,image synthesis,alignment loss,eye center detection,StarGAN2,MT-CNN,HR-Net
AI 理解论文
溯源树
样例
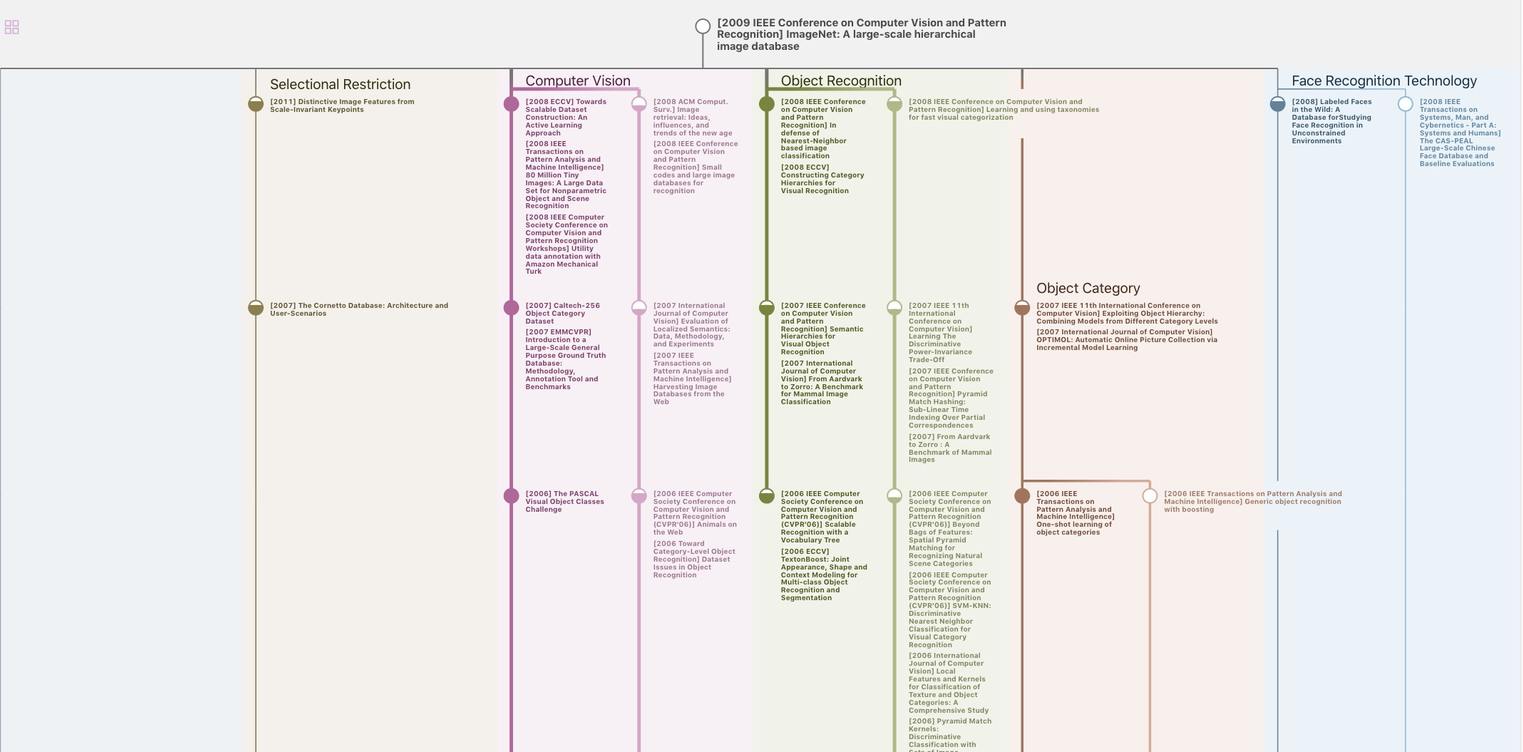
生成溯源树,研究论文发展脉络
Chat Paper
正在生成论文摘要