TPN: Triple parts network for few-shot instance segmentation
Multimedia Tools and Applications(2023)
摘要
Few-shot instance segmentation aims to segment unseen objects in a so-called query image, given only one close-up illustration named support image. Recently, many few-shot instance segmentation methods are based on Mask R-CNN, and do not take advantage of the information embedding in support images. In this work, we propose Triple Parts Network (TPN) based on Mask R-CNN for few-shot instance segmentation consisting of three key modules, i.e., Attention Fusion Module (AFM), Cosine Similarity based Classifier with Circle Loss (CSC), and Cross-Local Module (CLM). AFM generates proposals targeting at the object in the support image. CSC uses cosine similarity with circle loss to classify objects. CLM is applied to the mask branch to establish a full relation between support feature maps and query feature maps. The experiments are conducted on the Microsoft COCO and PASCAL VOC benchmarks, and the results show that our TPN has better performance than other state-of-the-art methods.
更多查看译文
关键词
Few-shot learning, Object detection, Instance segmentation
AI 理解论文
溯源树
样例
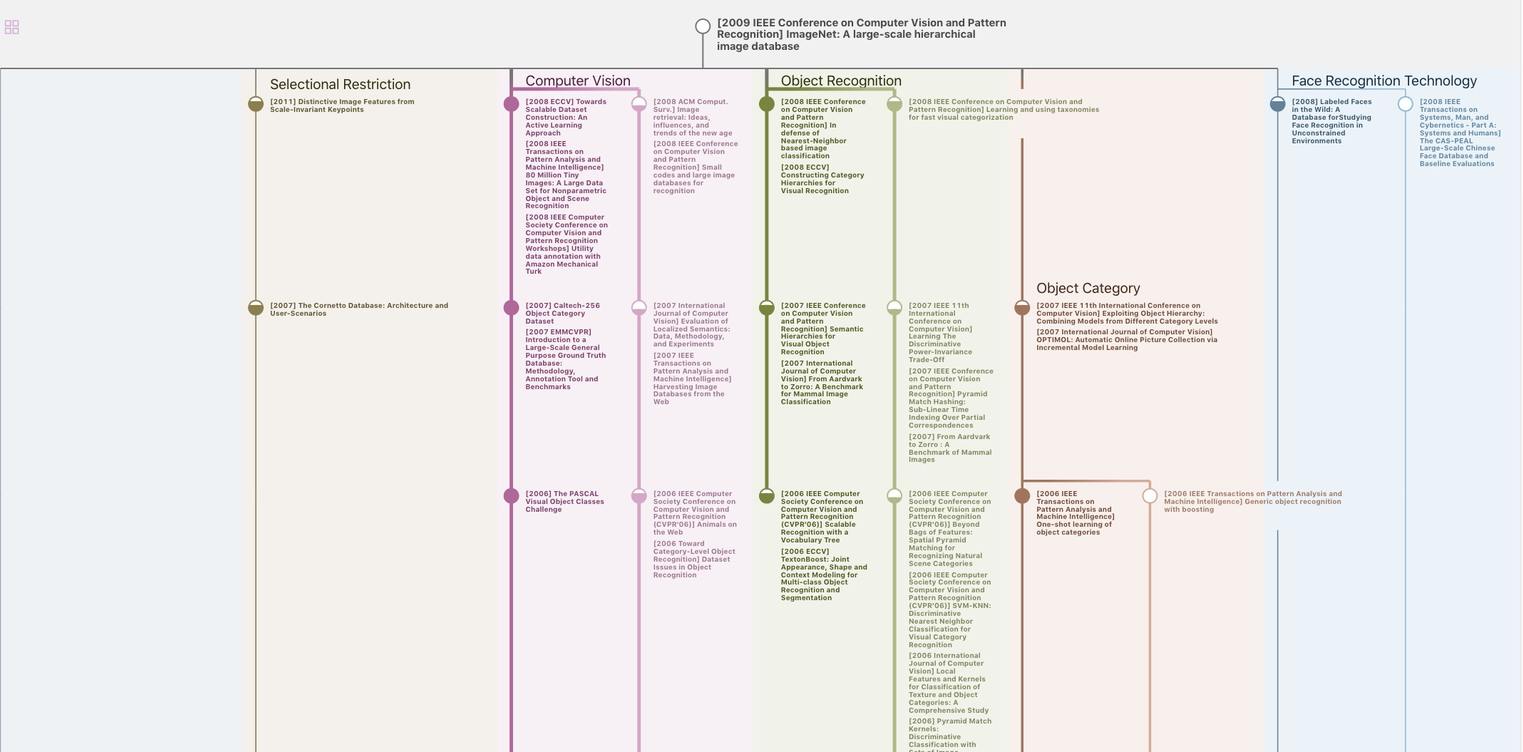
生成溯源树,研究论文发展脉络
Chat Paper
正在生成论文摘要