Measuring Progress in Fine-grained Vision-and-Language Understanding
conf_acl(2023)
摘要
While pretraining on large-scale image-text data from the Web has facilitated rapid progress on many vision-and-language (V&L) tasks, recent work has demonstrated that pretrained models lack "fine-grained" understanding, such as the ability to recognise relationships, verbs, and numbers in images. This has resulted in an increased interest in the community to either develop new benchmarks or models for such capabilities. To better understand and quantify progress in this direction, we investigate four competitive V&L models on four fine-grained benchmarks. Through our analysis, we find that X-VLM (Zeng et al., 2022) consistently outperforms other baselines, and that modelling innovations can impact performance more than scaling Web data, which even degrades performance sometimes. Through a deeper investigation of X-VLM, we highlight the importance of both novel losses and rich data sources for learning fine-grained skills. Finally, we inspect training dynamics, and discover that for some tasks, performance peaks early in training or significantly fluctuates, never converging.
更多查看译文
关键词
progress,understanding,fine-grained,vision-and-language
AI 理解论文
溯源树
样例
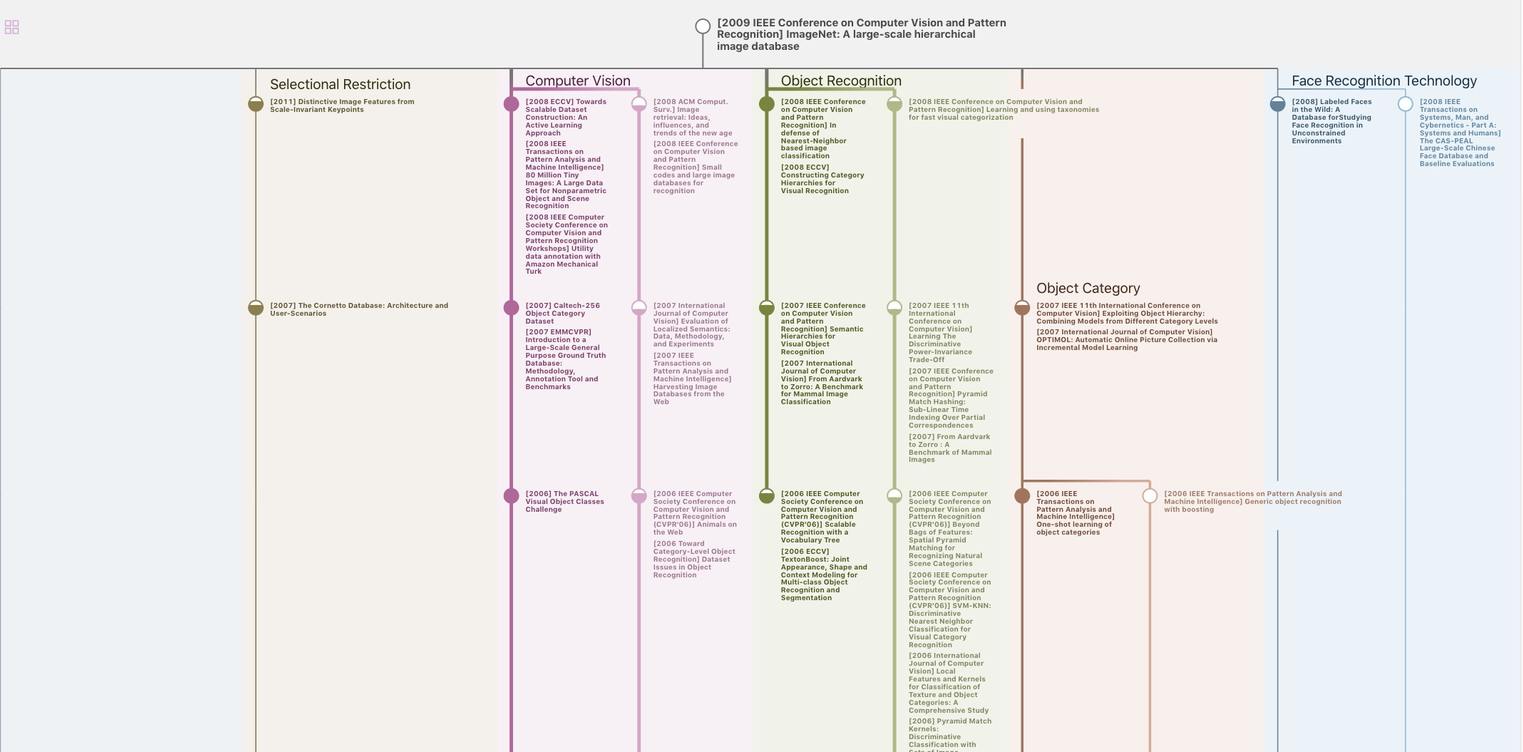
生成溯源树,研究论文发展脉络
Chat Paper
正在生成论文摘要