ZARA: Improving Few-Shot Self-Rationalization for Small Language Models.
CoRR(2023)
摘要
Language models (LMs) that jointly generate end-task answers as well as free-text rationales are known as self-rationalization models. Recent works demonstrate great performance gain for self-rationalization by few-shot prompting LMs with rationale-augmented exemplars. However, the ability to benefit from explanations only emerges with large-scale LMs, which have poor accessibility. In this work, we explore the less-studied setting of leveraging explanations for small LMs to improve few-shot self-rationalization. We first revisit the relationship between rationales and answers. Inspired by the implicit mental process of how human beings assess explanations, we present a novel approach, Zero-shot Augmentation of Rationale-Answer pairs (ZARA), to automatically construct pseudo-parallel data for self-training by reducing the problem of plausibility judgement to natural language inference. Experimental results show ZARA achieves SOTA performance on the FEB benchmark, for both the task accuracy and the explanation metric. In addition, we conduct human and quantitative evaluation validating ZARA's ability to automatically identify plausible and accurate rationale-answer pairs.
更多查看译文
关键词
small language models,few-shot,self-rationalization
AI 理解论文
溯源树
样例
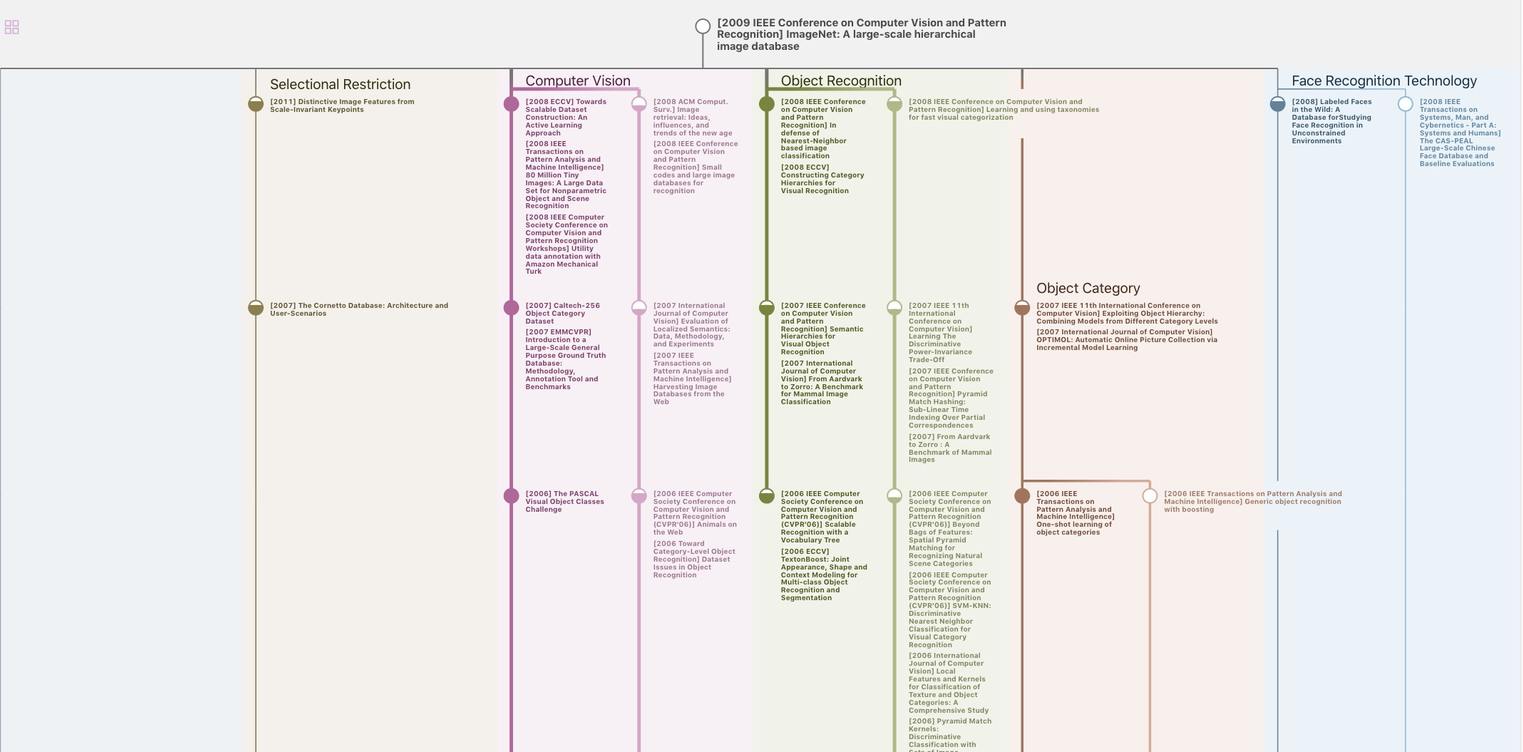
生成溯源树,研究论文发展脉络
Chat Paper
正在生成论文摘要