Weighted scoringRules: Emphasising Particular Outcomes when Evaluating Probabilistic Forecasts
arXiv (Cornell University)(2023)
摘要
When predicting future events, it is common to issue forecasts that are probabilistic, in the form of probability distributions over the range of possible outcomes. Such forecasts can be evaluated using proper scoring rules. Proper scoring rules condense forecast performance into a single numerical value, allowing competing forecasters to be ranked and compared. To facilitate the use of scoring rules in practical applications, the scoringRules package in R provides popular scoring rules for a wide range of forecast distributions. This paper discusses an extension to the scoringRules package that additionally permits the implementation of popular weighted scoring rules. Weighted scoring rules allow particular outcomes to be targeted during forecast evaluation, recognising that certain outcomes are often of more interest than others when assessing forecast quality. This introduces the potential for very flexible, user-oriented evaluation of probabilistic forecasts. We discuss the theory underlying weighted scoring rules, and describe how they can readily be implemented in practice using scoringRules. Functionality is available for weighted versions of several popular scoring rules, including the logarithmic score, the continuous ranked probability score (CRPS), and the energy score. Two case studies are presented to demonstrate this, whereby weighted scoring rules are applied to univariate and multivariate probabilistic forecasts in the fields of meteorology and economics.
更多查看译文
关键词
weighted scoringrules,particular outcomes,evaluating
AI 理解论文
溯源树
样例
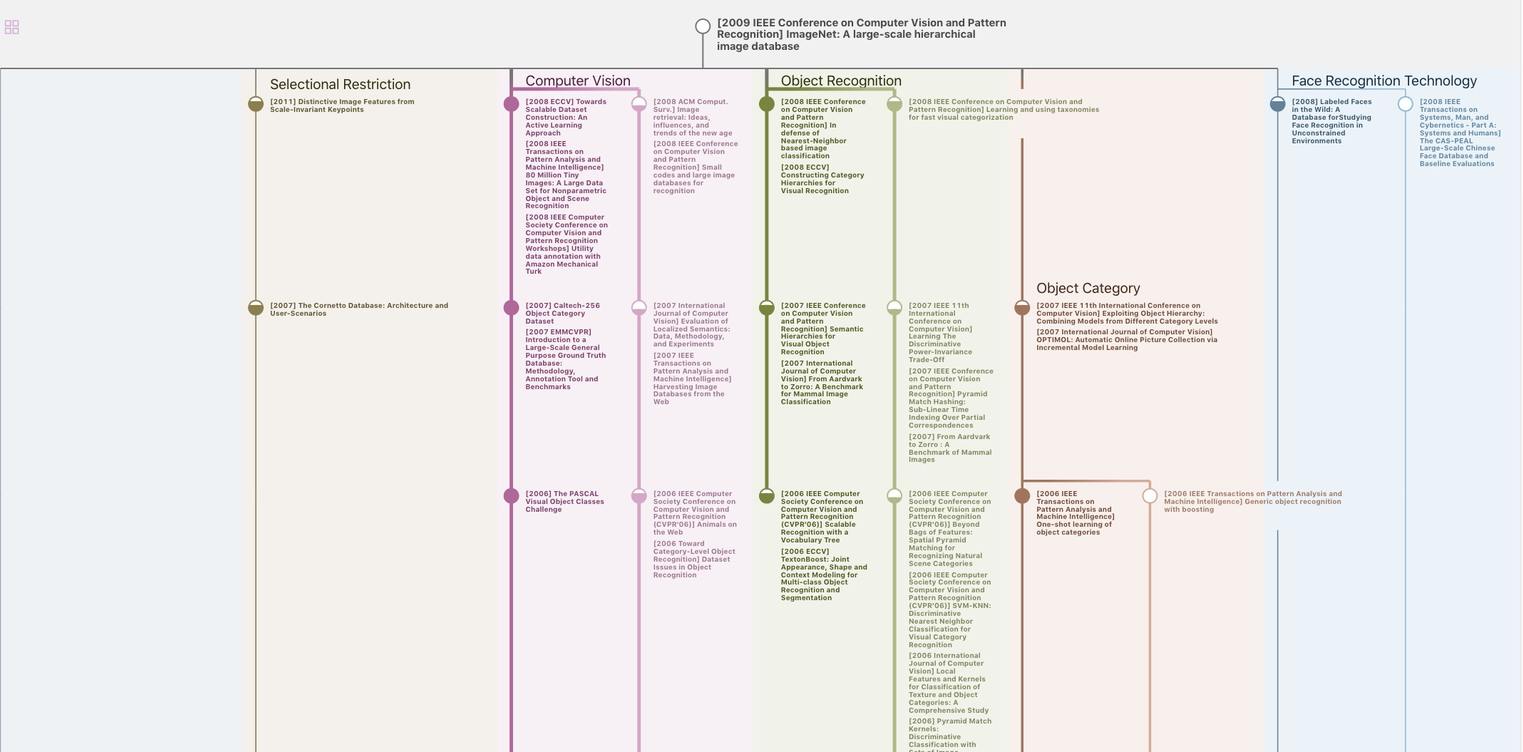
生成溯源树,研究论文发展脉络
Chat Paper
正在生成论文摘要