Learning to Code on Graphs for Topological Interference Management
CoRR(2023)
摘要
The state-of-the-art coding schemes for topological interference management (TIM) problems are usually handcrafted for specific families of network topologies, relying critically on experts' domain knowledge. This inevitably restricts the potential wider applications to wireless communication systems, due to the limited generalizability. This work makes the first attempt to advocate a novel intelligent coding approach to mimic topological interference alignment (IA) via local graph coloring algorithms, leveraging the new advances of graph neural networks (GNNs) and reinforcement learning (RL). The proposed LCG framework is then generalized to discover new IA coding schemes, including one-to-one vector IA and subspace IA. The extensive experiments demonstrate the excellent generalizability and transferability of the proposed approach, where the parameterized GNNs trained by small size TIM instances are able to work well on new unseen network topologies with larger size.
更多查看译文
关键词
excellent generalizability,experts,local graph coloring algorithms,novel intelligent coding approach,potential wider applications,reinforcement learning,size TIM instances,specific families,state-of-the-art coding schemes,topological interference alignment,topological interference management problems,unseen network topologies,wireless communication systems
AI 理解论文
溯源树
样例
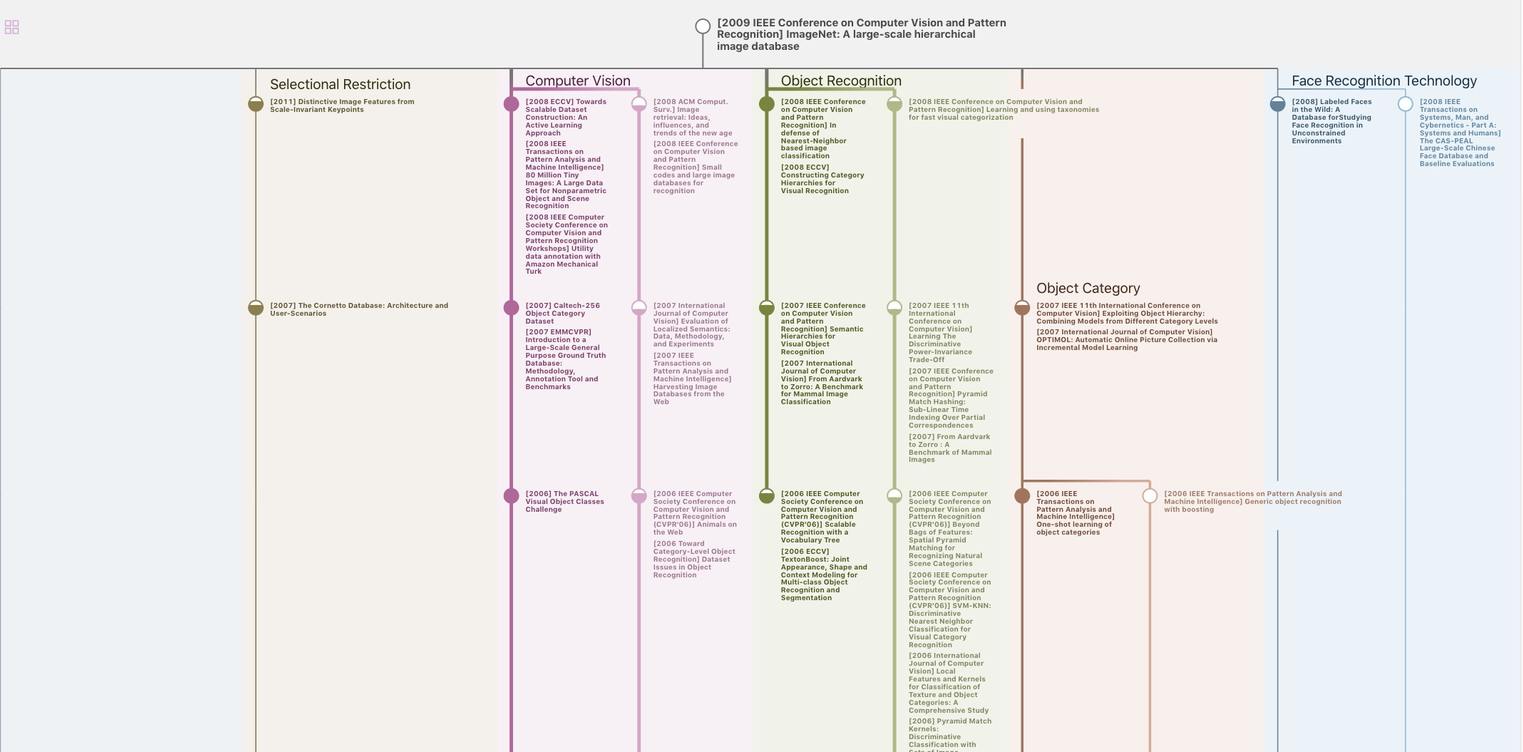
生成溯源树,研究论文发展脉络
Chat Paper
正在生成论文摘要