Improving the accuracy of genomic prediction for meat quality traits using whole genome sequence data in pigs
Journal of Animal Science and Biotechnology(2023)
摘要
Background Pork quality can directly affect customer purchase tendency and meat quality traits have become valuable in modern pork production. However, genetic improvement has been slow due to high phenotyping costs. In this study, whole genome sequence (WGS) data was used to evaluate the prediction accuracy of genomic best linear unbiased prediction (GBLUP) for meat quality in large-scale crossbred commercial pigs. Results We produced WGS data (18,695,907 SNPs and 2,106,902 INDELs exceed quality control) from 1,469 sequenced Duroc × (Landrace × Yorkshire) pigs and developed a reference panel for meat quality including meat color score, marbling score, L* (lightness), a* (redness), and b* (yellowness) of genomic prediction. The prediction accuracy was defined as the Pearson correlation coefficient between adjusted phenotypes and genomic estimated breeding values in the validation population. Using different marker density panels derived from WGS data, accuracy differed substantially among meat quality traits, varied from 0.08 to 0.47. Results showed that MultiBLUP outperform GBLUP and yielded accuracy increases ranging from 17.39% to 75%. We optimized the marker density and found medium- and high-density marker panels are beneficial for the estimation of heritability for meat quality. Moreover, we conducted genotype imputation from 50K chip to WGS level in the same population and found average concordance rate to exceed 95% and r 2 = 0.81. Conclusions Overall, estimation of heritability for meat quality traits can benefit from the use of WGS data. This study showed the superiority of using WGS data to genetically improve pork quality in genomic prediction.
更多查看译文
关键词
Genomic prediction,Meat quality,Pigs,Whole genome sequence
AI 理解论文
溯源树
样例
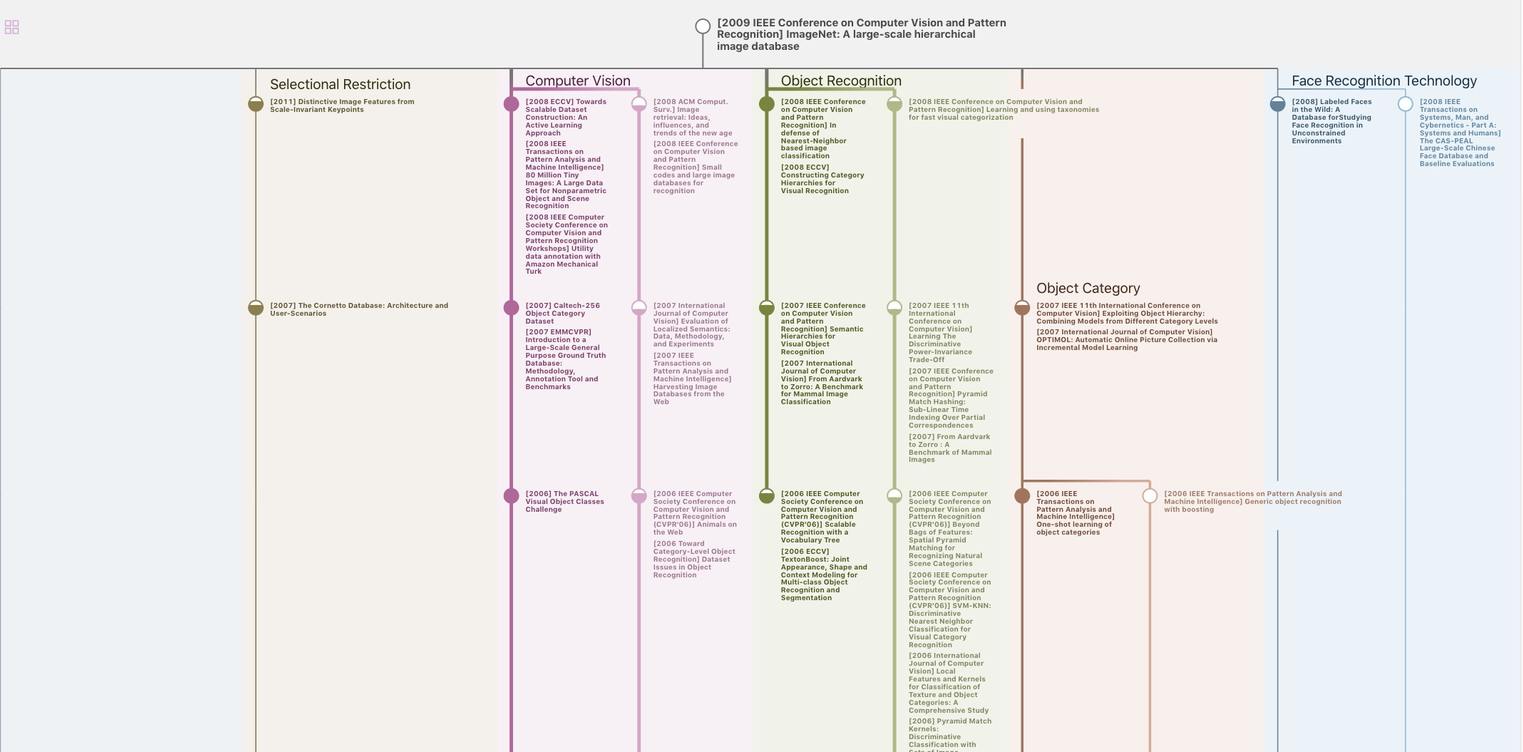
生成溯源树,研究论文发展脉络
Chat Paper
正在生成论文摘要