MNL: A Highly-Efficient Model for Large-scale Dynamic Weighted Directed Network Representation
IEEE Transactions on Big Data(2023)
摘要
A Non-negative Latent-factorization-of-tensors model relying on a
N
onnegative and
M
ultiplicative
U
pdate on
I
ncomplete
T
ensors (NMU-IT) algorithm facilitates efficient representation learning to a
D
ynamic
W
eighted
D
irected
N
etwork (DWDN). However, a NMU-IT algorithm leads to slow model convergence and inefficient selection of hyper-parameters. Aiming to address these challenging issues, this work proposes a Momentum-incorporated Biased Non-negative and Adaptive Latent-factorization-of-tensors (MNL) model. It adopts two-fold ideas: 1) incorporating a generalized momentum method into the NMU-IT algorithm to enable fast model convergence; 2) facilitating hyper-parameter slef-adaptation via Particle Swarm Optimization. Empirical studies on four real DWDNs indicate that the proposed MNL is superior to state-of-the-art models in performing efficient representation learning to a DWDN, which is definitely supported by its high computational efficiency and prediction accuracy for missing links of a DWDN. Moreover, its hyper-parameter-free training enables its high practicability in real scenes.
更多查看译文
关键词
Dynamic weighted directed network,high-dimensional and incomplete tensor,non-negative latent-factorization-of-tensors,linear bias,high dimensional and incomplete,momentum method,particle swarm optimization,adaptive model
AI 理解论文
溯源树
样例
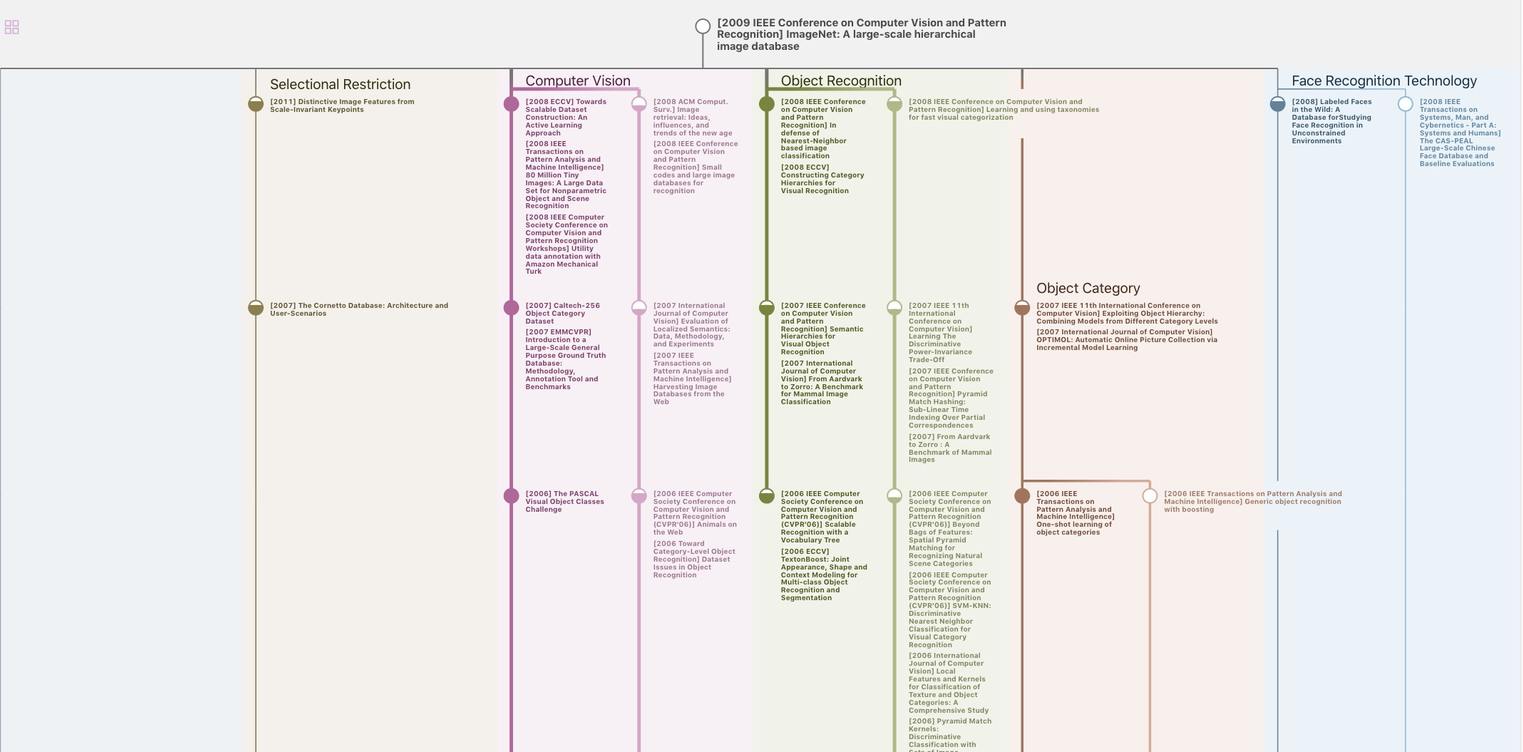
生成溯源树,研究论文发展脉络
Chat Paper
正在生成论文摘要