A 608nW Near-Microphone Keyword-Spotting Chip Using Real-Point Serial FFT-Based MFCC and Temporal Depthwise Separable CNN in 28nm CMOS
2023 IEEE Custom Integrated Circuits Conference (CICC)(2023)
摘要
In wearable and mobile devices, speech interfaces are increasingly equipped with keyword-spotting (KWS) functions. The always-on characteristic requires KWS to achieve ultra-low power while keeping good accuracy, which is a major concern for KWS ASICs. For the frontend, most commercial MEMS microphones consume power up to $\gt 100 \mu \mathrm{W}$, which breaks the low-power effort by the state-of-the-art (SoTA) works [1, 2] that lack a fully-integrated near-microphone single-chip solution. For the feature extractor (FEx), analog FExs have achieved the low power of $9.3 \mu \mathrm{W}$ [3] and 109nW [4], but weaken the detection accuracy due to low-quality features. Scaling-friendly digital FExs [1, 5] have the advantage of extracting high-quality features, but the computation complexity and memory optimization are still key issues. For the classifier, convolutional neural networks (CNNs) are commonly applied to KWS, achieving superior accuracy results. However, their complex networks cause redundant computation and hardware cost at the edge.
更多查看译文
AI 理解论文
溯源树
样例
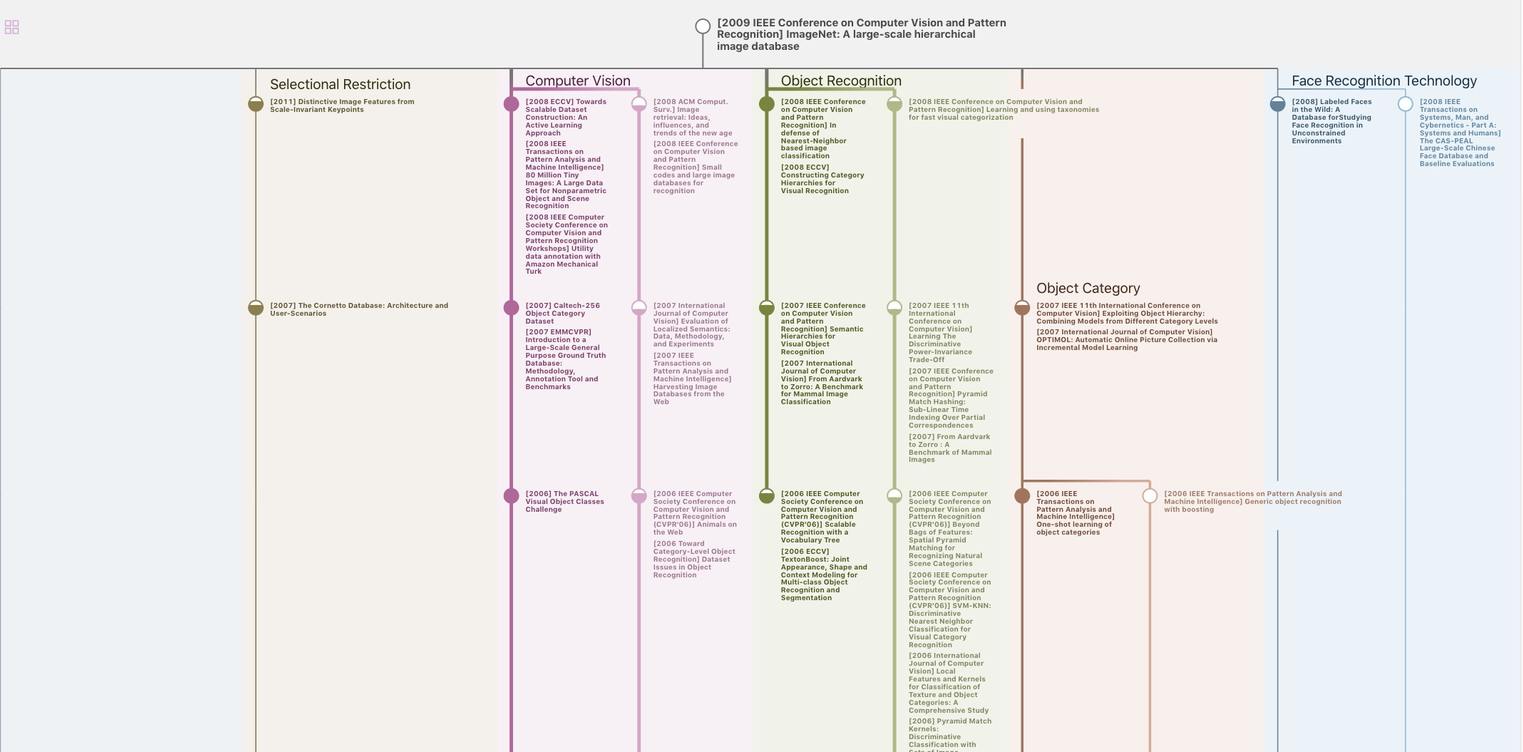
生成溯源树,研究论文发展脉络
Chat Paper
正在生成论文摘要