A Double-Mode Sparse Compute-In-Memory Macro with Reconfigurable Single and Dual Layer Computation
2023 IEEE Custom Integrated Circuits Conference (CICC)(2023)
摘要
Compute-in-memory (C1M) is an energy-efficient approach to tackle the memory-wall bottlenecks of memory-hungry Al tasks. Despite the mixed-signal C1M macros [1] –[5] showing outstanding computing efficiency, they still face various challenges such as accuracy loss from mismatch and noise, low scalability, frequent inter-layer data access with DA/AD conversion, etc. Moreover, the redundant computation in DNN limits the achievable system efficiency. Exploiting data sparsity [6] can render a high-energy efficiency, but often requires extra control logic or specific training, inducing either hardware or software overhead.
更多查看译文
AI 理解论文
溯源树
样例
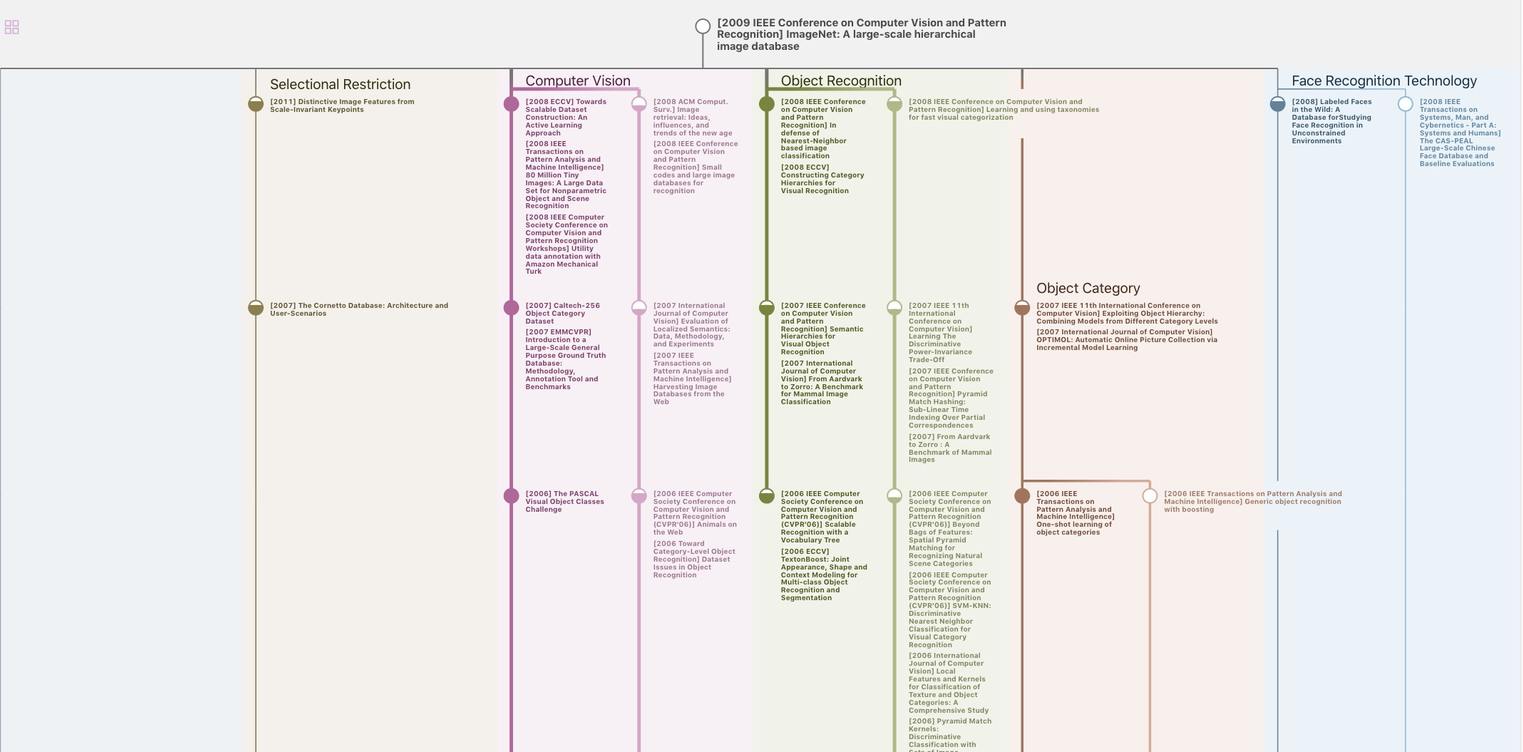
生成溯源树,研究论文发展脉络
Chat Paper
正在生成论文摘要