Scalable federated learning for emergency care using low cost microcomputing: Real-world, privacy preserving development and evaluation of a COVID-19 screening test in UK hospitals
medRxiv (Cold Spring Harbor Laboratory)(2023)
摘要
Background Tackling biases in medical artificial intelligence requires multi-centre collaboration, however, ethical, legal and entrustment considerations may restrict providers' ability to participate. Federated learning (FL) may eliminate the need for data sharing by allowing algorithm development across multiple hospitals without data transfer. Previously, we have shown an AI-driven screening solution for COVID-19 in emergency departments using clinical data routinely available within 1h of arrival to hospital (vital signs & blood tests; CURIAL-Lab). Here, we aimed to extend and federate our COVID-19 screening test, demonstrating development and evaluation of a rapidly scalable and user-friendly FL solution across 4 UK hospital groups. Methods We supplied a Raspberry Pi 4 Model B device, preloaded with our end-to-end FL pipeline, to 4 NHS hospital groups or their locally-linked research university (Oxford University Hospitals/University of Oxford (OUH), University Hospitals Birmingham/University of Birmingham (UHB), Bedfordshire Hospitals (BH) and Portsmouth Hospitals University (PUH) NHS trusts). OUH, PUH and UHB participated in federated training and calibration, training a deep neural network (DNN) and logistic regressor to predict COVID-19 status using clinical data for pre- pandemic (COVID-19-negative) admissions and COVID-19-positive cases from the first wave. We performed federated prospective evaluation at PUH & OUH, and external evaluation at BH, evaluating the resultant global and site-tuned models for admissions to the respective sites during the second pandemic wave. Removable microSD storage was destroyed on study completion. Findings Routinely collected clinical data from a total 130,941 patients (1,772 COVID-19 positive) across three hospital groups were included in federated training. OUH, PUH and BH participated in prospective federated evaluation, with sets comprising 32,986 patient admissions (3,549 positive) during the second pandemic wave. Federated training improved DNN performance by a mean of 27.6% in terms of AUROC when compared to models trained locally, from AUROC of 0.574 & 0.622 at OUH & PUH to 0.872 & 0.876 for the federated global model. Performance improvement was more modest for a logistic regressor with a mean AUROC increase of 13.9%. During federated external evaluation at BH, the global DNN model achieved an AUROC of 0.917 (0.893-0.942), with 89.7% sensitivity (83.6-93.6) and 76.7% specificity (73.9- 79.1). Site-personalisation of the global model did not give a significant improvement in overall performance (AUROC improvement <0.01), suggesting high generalisability. Interpretations We present a rapidly scalable hardware and software FL solution, developing a COVID-19 screening test across four UK hospital groups using inexpensive micro- computing hardware. Federation improved model performance and generalisability, and shows promise as an enabling technology for deep learning in healthcare. Funding University of Oxford Medical & Life Sciences Translational Fund/Wellcome
### Competing Interest Statement
Declarations: DWE reports personal fees from Gilead, outside the submitted work; DAC reports personal fees from Oxford University Innovation, personal fees from BioBeats, personal fees from Sensyne Health, outside the submitted work. No other authors report any conflicts of interest.
### Funding Statement
Funding: This study was supported by the Wellcome Trust/University of Oxford Medical & Life Sciences Translational Fund (Award: 0009350) and the Oxford National Institute of Research (NIHR) Biomedical Research Campus (BRC). The funders of the study had no role in study design, data collection, data analysis, data interpretation, or writing of the manuscript. AS is an NIHR Academic Clinical Fellow (Award: ACF-2020-13-015). DWE is a Robertson Foundation Fellow and an NIHR Oxford Biomedical Research Centre Senior Fellow. The views expressed are those of the authors and not necessarily those of the NHS, NIHR, or the Wellcome Trust.
### Author Declarations
I confirm all relevant ethical guidelines have been followed, and any necessary IRB and/or ethics committee approvals have been obtained.
Yes
The details of the IRB/oversight body that provided approval or exemption for the research described are given below:
NHS Health Research Authority approval was granted for the use of routine clinical and microbiology data from electronic health records (EHRs) for development and validation of artificial intelligence models to detect COVID-19 (CURIAL; IRAS ID 281832).
I confirm that all necessary patient/participant consent has been obtained and the appropriate institutional forms have been archived, and that any patient/participant/sample identifiers included were not known to anyone (e.g., hospital staff, patients or participants themselves) outside the research group so cannot be used to identify individuals.
Yes
I understand that all clinical trials and any other prospective interventional studies must be registered with an ICMJE-approved registry, such as ClinicalTrials.gov. I confirm that any such study reported in the manuscript has been registered and the trial registration ID is provided (note: if posting a prospective study registered retrospectively, please provide a statement in the trial ID field explaining why the study was not registered in advance).
Yes
I have followed all appropriate research reporting guidelines, such as any relevant EQUATOR Network research reporting checklist(s) and other pertinent material, if applicable.
Yes
Data & Code Availability: The code for our federated learning pipeline is available online alongside publication, via Github. Although, by design, patient data was not transferred within the FL for this study, AS, JY, DWE and DAC have previously had access to the raw data within a prior related evaluation study (Soltan et. al 2022). PD accessed and verified the data at BH. MAS & DRT accessed and verified the data at UHB. LDC accessed and verified the data at PUH. Data from OUH studied here are available from the Infections in Oxfordshire Research Database (https://oxfordbrc.nihr.ac.uk/research-themes-overview/antimicrobial-resistance-and- modernising-microbiology/infections-in-oxfordshire-research-database-iord/), subject to an application meeting the ethical and governance requirements of the Database. Data from UHB, PUH and BH are available on reasonable request to the respective trusts subject to NHS HRA requirements.
更多查看译文
关键词
federated learning,uk hospitals,emergency care,privacy,real-world
AI 理解论文
溯源树
样例
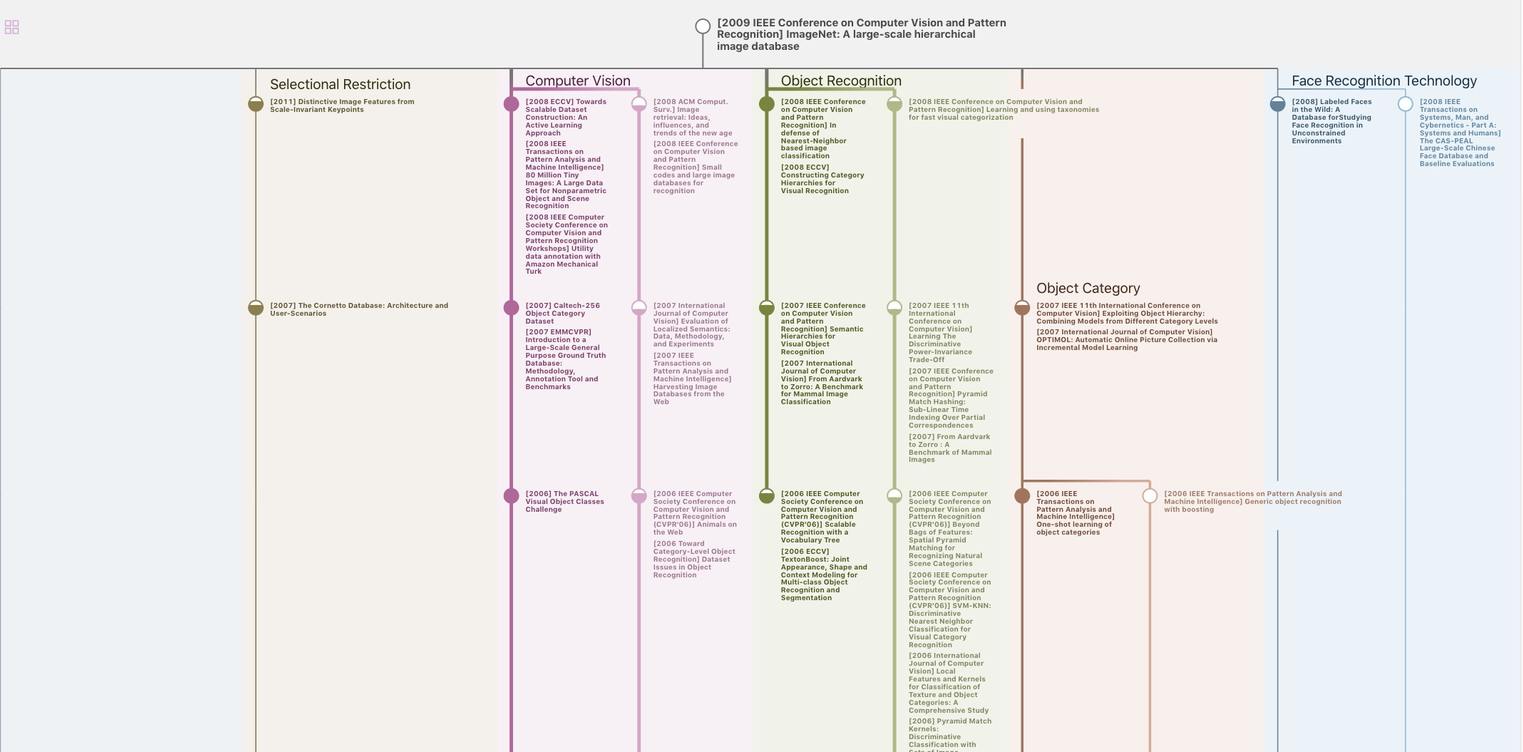
生成溯源树,研究论文发展脉络
Chat Paper
正在生成论文摘要