Modeling Based on Ensemble Learning Methods for Detection of Diagnostic Biomarkers from LncRNA Data in Rats Treated with Cis-Platinum-Induced Hepatotoxicity
Diagnostics(2023)
摘要
Background: The first aim of this study is to perform bioinformatic analysis of lncRNAs obtained from liver tissue samples from rats treated with cisplatin hepatotoxicity and without pathology. Another aim is to identify possible biomarkers for the diagnosis/early diagnosis of hepatotoxicity by modeling the data obtained from bioinformatics analysis with ensemble learning methods. Methods: In the study, 20 female Sprague-Dawley rats were divided into a control group and a hepatotoxicity group. Liver samples were taken from rats, and transcriptomic and histopathological analyses were performed. The dataset achieved from the transcriptomic analysis was modeled with ensemble learning methods (stacking, bagging, and boosting). Modeling results were evaluated with accuracy (Acc), balanced accuracy (B-Acc), sensitivity (Se), specificity (Sp), positive predictive value (Ppv), negative predictive value (Npv), and F1 score performance metrics. As a result of the modeling, lncRNAs that could be biomarkers were evaluated with variable importance values. Results: According to histopathological and immunohistochemical analyses, a significant increase was observed in the sinusoidal dilatation and Hsp60 immunoreactivity values in the hepatotoxicity group compared to the control group (p < 0.0001). According to the results of the bioinformatics analysis, 589 lncRNAs showed different expressions in the groups. The stacking model had the best classification performance among the applied ensemble learning models. The Acc, B-Acc, Se, Sp, Ppv, Npv, and F1-score values obtained from this model were 90%, 90%, 80%, 100%, 100%, 83.3%, and 88.9%, respectively. lncRNAs with id rna-XR_005492522.1, rna-XR_005492536.1, and rna-XR_005505831.1 with the highest three values according to the variable importance obtained as a result of stacking modeling can be used as predictive biomarker candidates for hepatotoxicity. Conclusions: Among the ensemble algorithms, the stacking technique yielded higher performance results as compared to the bagging and boosting methods on the transcriptomic data. More comprehensive studies can support the possible biomarkers determined due to the research and the decisive results for the diagnosis of drug-induced hepatotoxicity.
更多查看译文
关键词
genomic,hepatotoxicity,machine learning,ensemble learning,biomarker
AI 理解论文
溯源树
样例
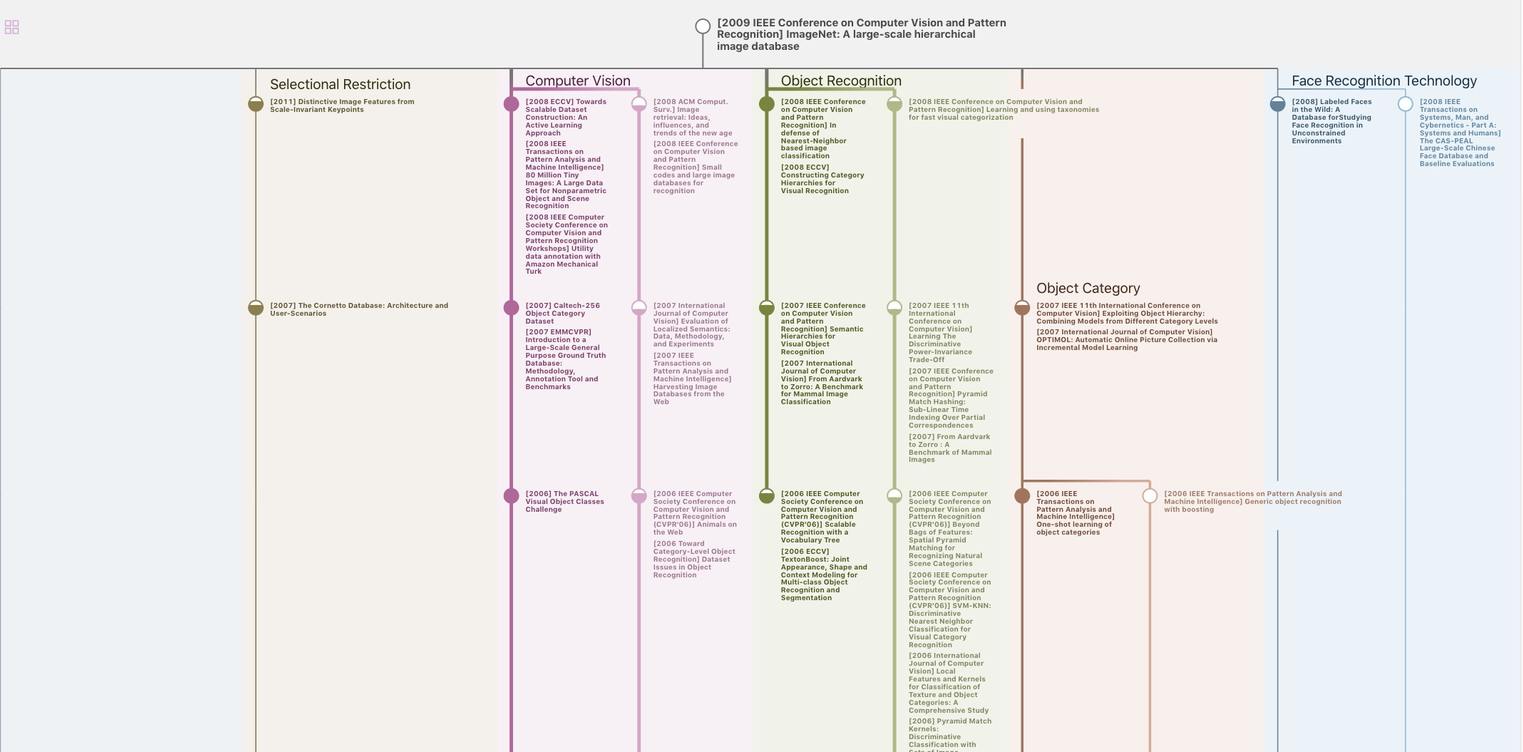
生成溯源树,研究论文发展脉络
Chat Paper
正在生成论文摘要