Data quality dimensions for fair AI
CoRR(2023)
摘要
AI systems are not intrinsically neutral and biases trickle in any type of technological tool. In particular when dealing with people, AI algorithms reflect technical errors originating with mislabeled data. As they feed wrong and discriminatory classifications, perpetuating structural racism and marginalization, these systems are not systematically guarded against bias. In this article we consider the problem of bias in AI systems from the point of view of Information Quality dimensions. We illustrate potential improvements of a bias mitigation tool in gender classification errors, referring to two typically difficult contexts: the classification of non-binary individuals and the classification of transgender individuals. The identification of data quality dimensions to implement in bias mitigation tool may help achieve more fairness. Hence, we propose to consider this issue in terms of completeness, consistency, timeliness and reliability, and offer some theoretical results.
更多查看译文
关键词
quality,data
AI 理解论文
溯源树
样例
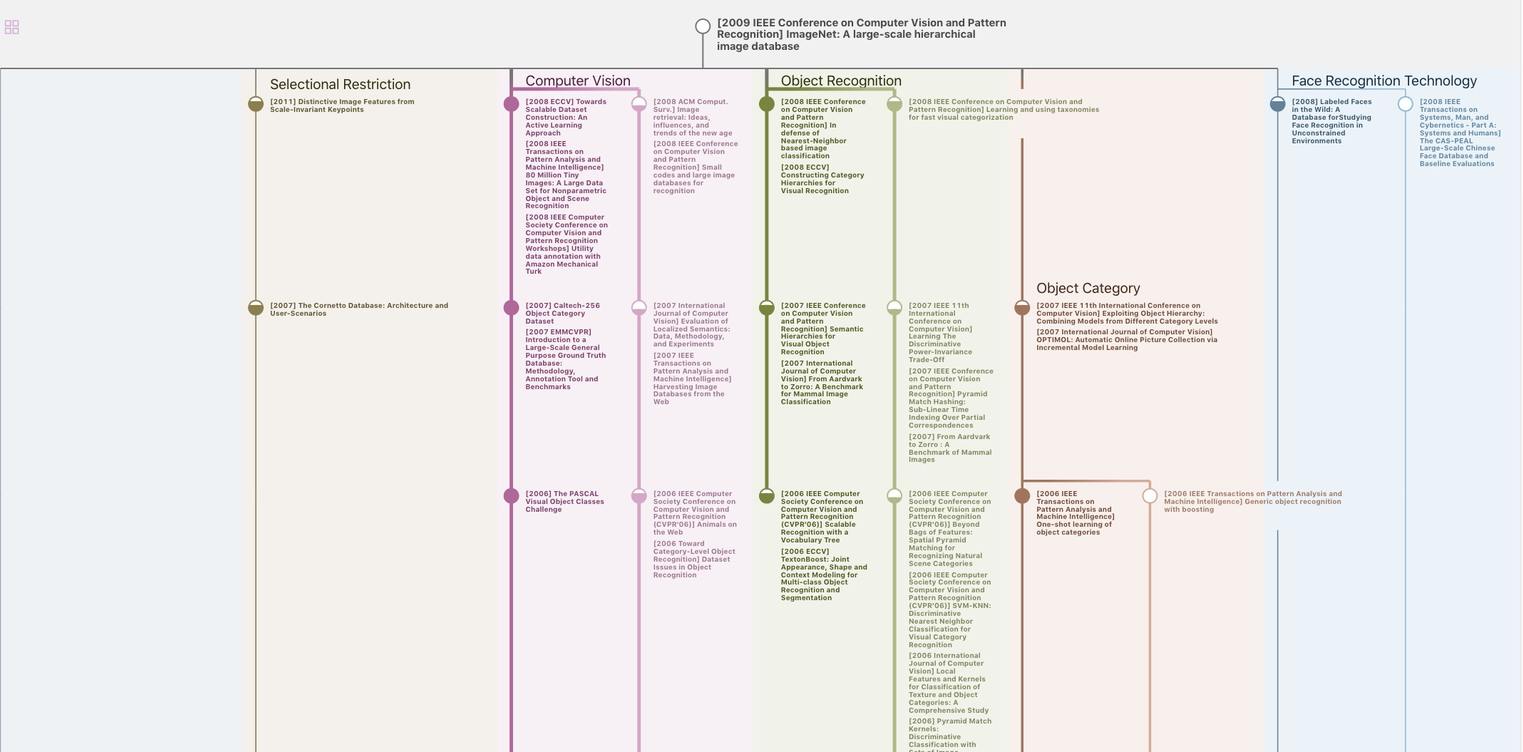
生成溯源树,研究论文发展脉络
Chat Paper
正在生成论文摘要