Bayesian sensitivity analysis for a missing data model
arxiv(2023)
摘要
In causal inference, sensitivity analysis is important to assess the robustness of study conclusions to key assumptions. We perform sensitivity analysis of the assumption that missing outcomes are missing completely at random. We follow a Bayesian approach, which is nonparametric for the outcome distribution and can be combined with an informative prior on the sensitivity parameter. We give insight in the posterior and provide theoretical guarantees in the form of Bernstein-von Mises theorems for estimating the mean outcome. We study different parametrisations of the model involving Dirichlet process priors on the distribution of the outcome and on the distribution of the outcome conditional on the subject being treated. We show that these parametrisations incorporate a prior on the sensitivity parameter in different ways and discuss the relative merits. We also present a simulation study, showing the performance of the methods in finite sample scenarios.
更多查看译文
关键词
bayesian sensitivity analysis,missing,data,model
AI 理解论文
溯源树
样例
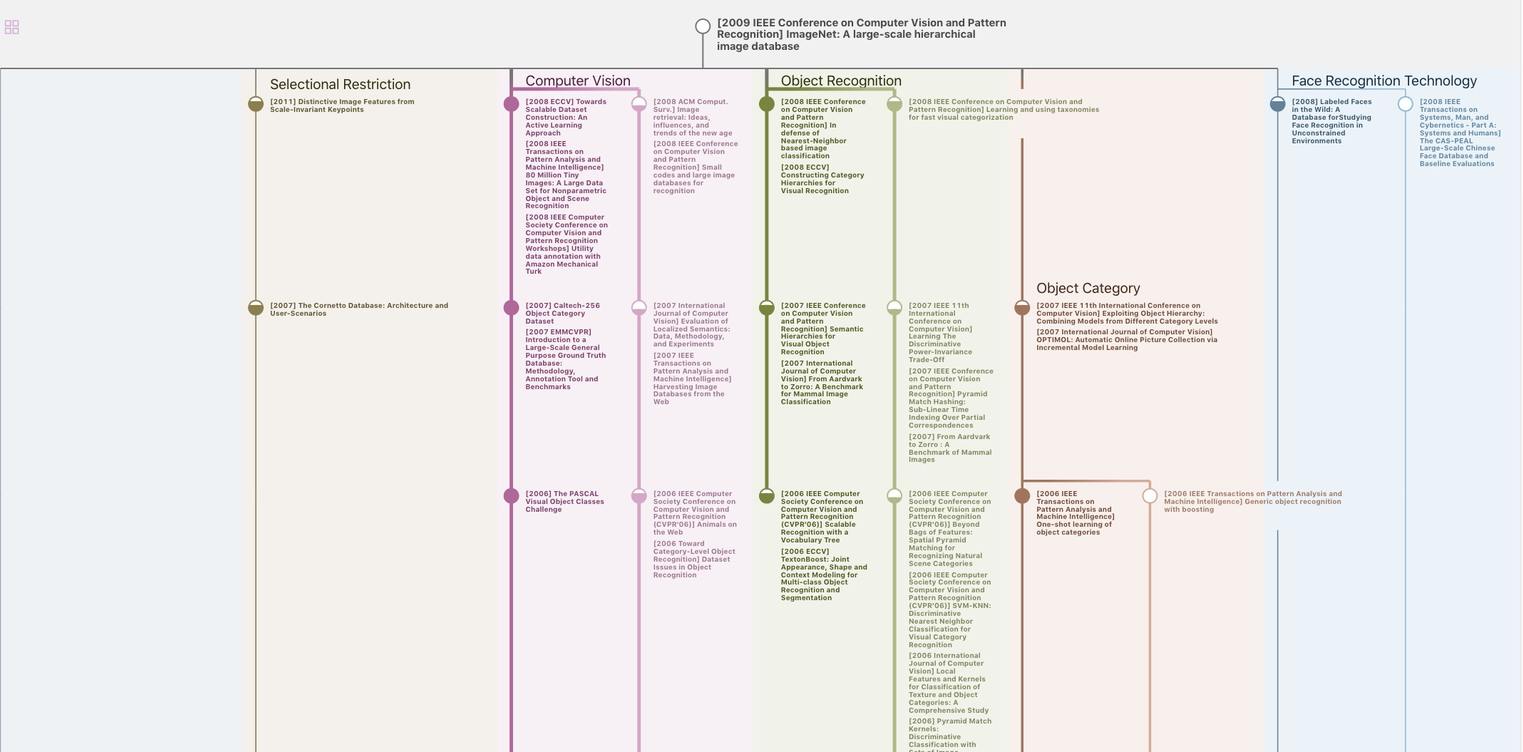
生成溯源树,研究论文发展脉络
Chat Paper
正在生成论文摘要