On the convergence of the MLE as an estimator of the learning rate in the Exp3 algorithm
CoRR(2023)
摘要
When fitting the learning data of an individual to algorithm-like learning models, the observations are so dependent and non-stationary that one may wonder what the classical Maximum Likelihood Estimator (MLE) could do, even if it is the usual tool applied to experimental cognition. Our objective in this work is to show that the estimation of the learning rate cannot be efficient if the learning rate is constant in the classical Exp3 (Exponential weights for Exploration and Exploitation) algorithm. Secondly, we show that if the learning rate decreases polynomially with the sample size, then the prediction error and in some cases the estimation error of the MLE satisfy bounds in probability that decrease at a polynomial rate.
更多查看译文
关键词
exp3 algorithm,learning rate,mle,estimator
AI 理解论文
溯源树
样例
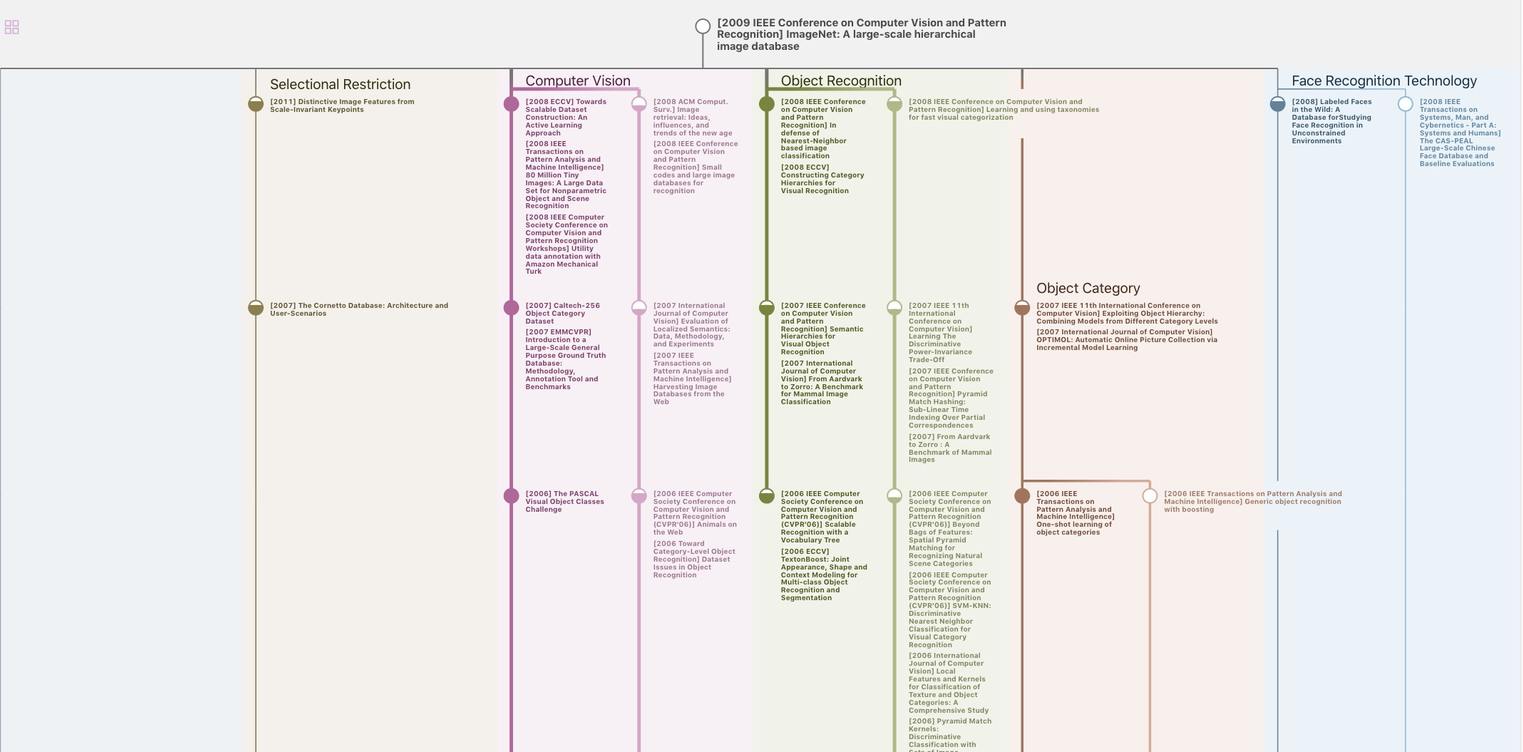
生成溯源树,研究论文发展脉络
Chat Paper
正在生成论文摘要