Leveraging relevant summarized information and multi-layer classification to generalize the detection of misleading headlines
DATA & KNOWLEDGE ENGINEERING(2023)
摘要
Disinformation is an important problem facing society nowadays. Given the rapid and easy access to information, news stories quickly go viral, the vast majority of which are misleading and with no prospect of verification. Specifically, the headline of a correctly designed news item must correspond to a summary of the main information of that news item and it should be neutral. However, many headlines circulating on the Internet use false or distorted information, seeking to confuse or mislead the reader. Misleading headlines indicate a dissonance between the headline and the content of the news story. From a computational perspective, this problem is being tackled as a Stance Detection problem between the headline and the body text of the news item. This paper contributes to the fight against the spread of misleading information by presenting a generic and flexible multi-level hierarchical classification. The approach is based on two stages that enable the detection of the stance between the news headline and the body text. The proposed architecture, called HeadlineStanceChecker+ uses the headline and only the essential information of the news item (not the full body text) as inputs. To extract this essential information, different summarization approaches (extractive and abstractive) are analyzed in order to determine the most relevant information for the task. The experimentation has been carried out using the Fake News Challenge (FNC-1) dataset. A 94.49% accuracy was obtained using extractive summaries, which were more helpful than abstractive ones. HeadlineStanceChecker+ improves the accuracy results of existing state-of -the-art systems. In conclusion, using automatic extractive summaries together with the two-stage generic architecture is an effective solution to the problem.
更多查看译文
关键词
Natural language processing,Fake news,Misleading headlines,Stance detection
AI 理解论文
溯源树
样例
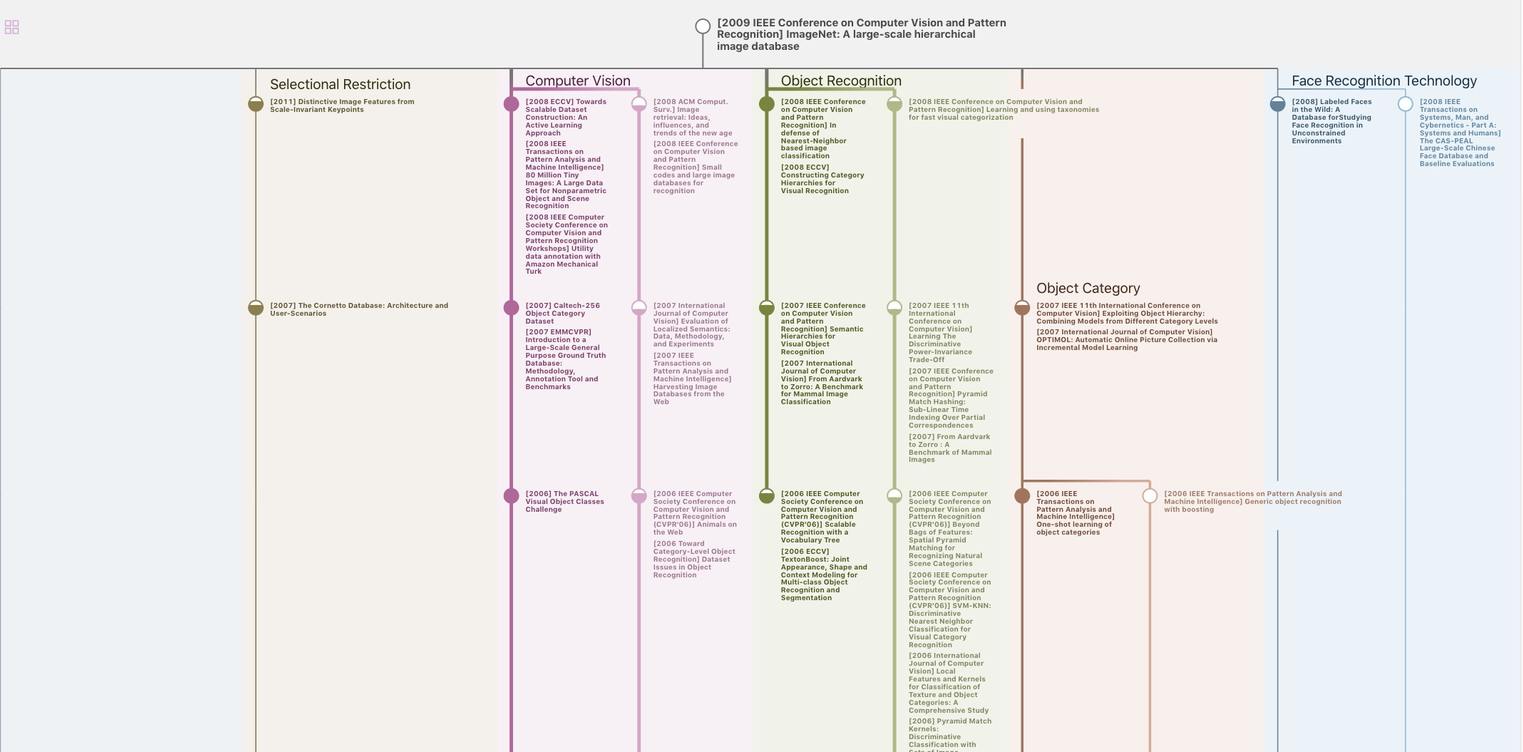
生成溯源树,研究论文发展脉络
Chat Paper
正在生成论文摘要