Enhancement of E-Learning Student's Performance Based on Ensemble Techniques
ELECTRONICS(2023)
摘要
Educational institutions have dramatically increased in recent years, producing many graduates and postgraduates each year. One of the critical concerns of decision-makers is student performance. Educational data mining techniques are beneficial to explore uncovered data in data itself, creating a pattern to analyze student performance. In this study, we investigate the student E-learning data that has increased significantly in the era of COVID-19. Thus, this study aims to analyze and predict student performance using information gathered from online systems. Evaluating the student E-learning data through the data mining model proposed in this study will help the decision-makers make informed and suitable decisions for their institution. The proposed model includes three traditional data mining methods, decision tree, Naive Bays, and random forest, which are further enhanced by the use of three ensemble techniques: bagging, boosting, and voting. The results demonstrated that the proposed model improved the accuracy from 0.75 to 0.77 when we used the DT method with boosting. Furthermore, the precision and recall results both improved from 0.76 to 0.78.
更多查看译文
关键词
educational data mining,student performance,classification techniques,ensemble methods
AI 理解论文
溯源树
样例
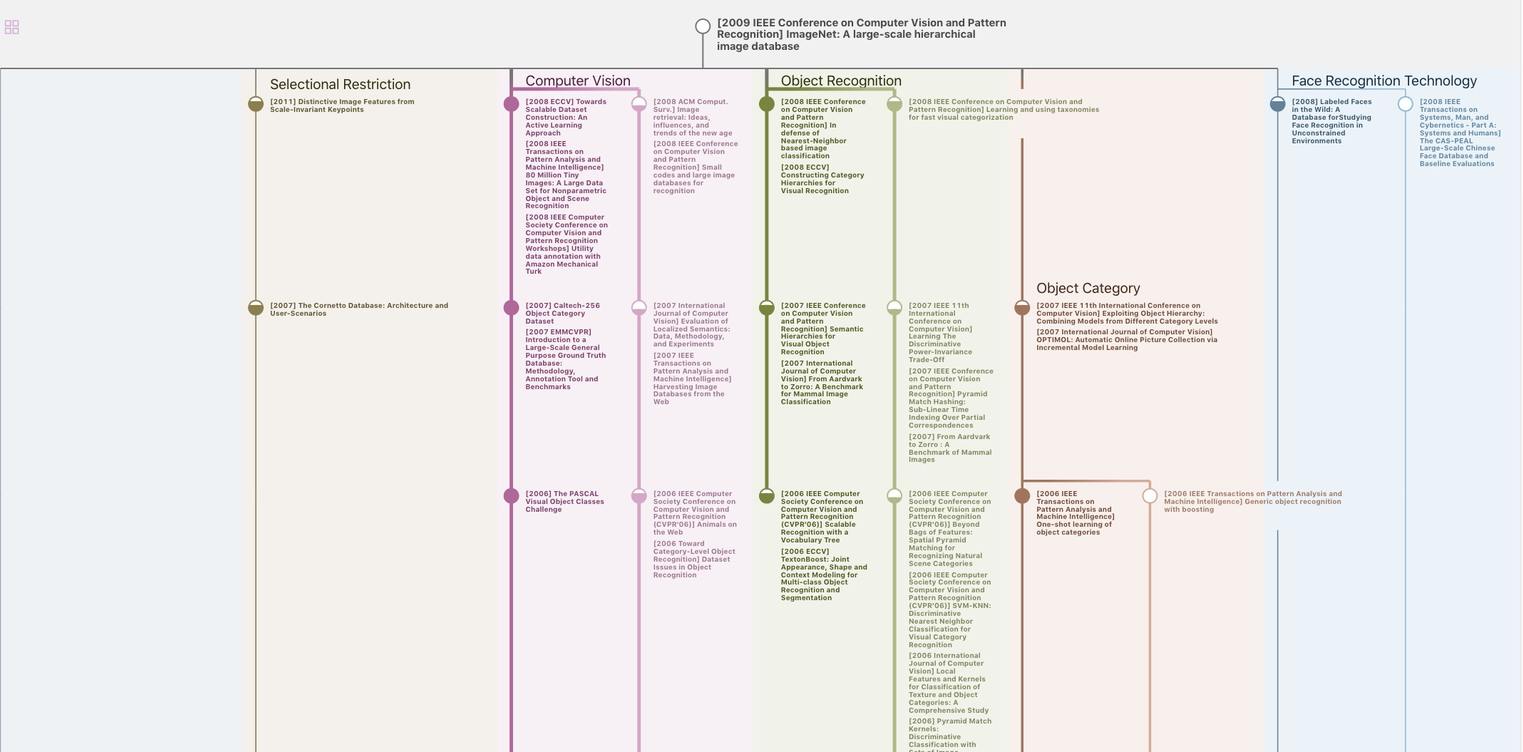
生成溯源树,研究论文发展脉络
Chat Paper
正在生成论文摘要