Quantifying the Safe Operating Space for Land-System SDG Achievement via Machine Learning and Scenario Discovery
EARTHS FUTURE(2023)
摘要
We developed a machine learning based surrogate model to identify sustainability pathways through rapid scenario generation and defined the safe operating space for achieving them via scenario discovery. We trained a surrogate model to replicate the Land-Use Trade-Offs integrated model of the Australian land system. Latin hypercube sampling was used to create many scenarios exploring the impact of uncertainties in key drivers including future socio-economic development, climate change mitigation, and agricultural productivity at a granular level. Economic and environmental impacts were evaluated against nationally downscaled SDG targets. Scenario discovery revealed new pathways to achieving five SDG targets for 2050 which required crop yield increases above 1.78 times, a carbon price above 100 AU$ tCO(2)(-1), a > 9% biodiversity levy on carbon plantings, and carefully regulated land-use policy. Machine learning based surrogate modeling teamed with scenario discovery revealed the policy and scenario settings required for a more sustainable future for the Australian land sector.Plain Language Summary Sustainable pathways are elusive in land systems due to their complexity, trade-offs, and uncertainties. We used a machine learning-based surrogate modeling approach to explore future uncertainties through rapid scenario generation and identify policy settings defining the safe operating space for future land sector sustainability.
更多查看译文
关键词
sustainable development goals,pathways,land‐use,uncertainty,machine learning,scenario discovery
AI 理解论文
溯源树
样例
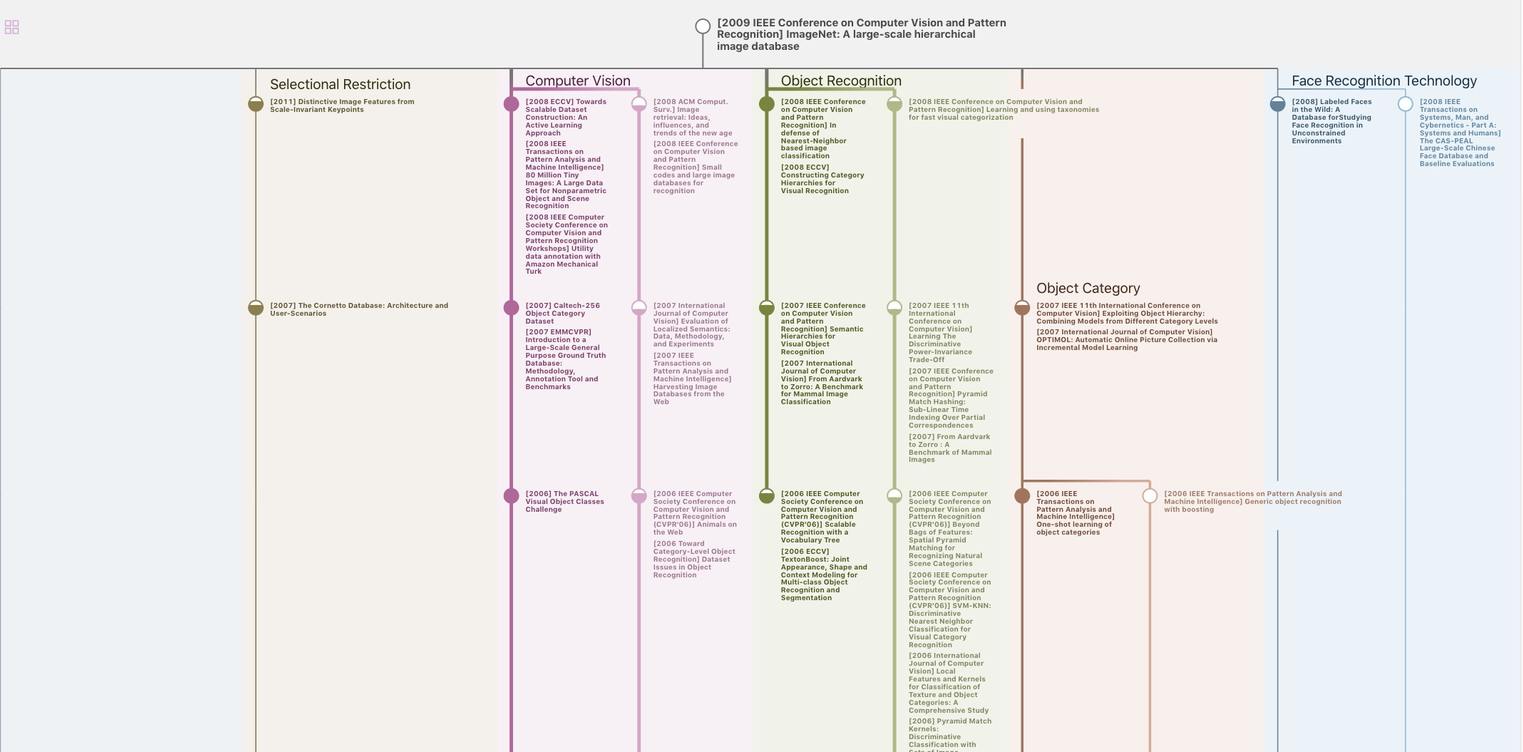
生成溯源树,研究论文发展脉络
Chat Paper
正在生成论文摘要