Predict water quality using an improved deep learning method based on spatiotemporal feature correlated: a case study of the Tanghe Reservoir in China
STOCHASTIC ENVIRONMENTAL RESEARCH AND RISK ASSESSMENT(2023)
摘要
The ecological health of water quality is an essential factor in the survival and development of human beings. By setting up multiple sensors in the water body, people observe the factual state of water quality, and collect a large number of sequence data containing time information. In order to effectively utilize water quality time series data and predict future water quality changes, this paper proposes an improved deep learning method based on spatiotemporal feature correlated, called the convolution recurrent basis expansion analysis architecture. The model improves the original model by using the ability of convolutional neural network structure to extract spatial features and the continuous memory ability of recurrent neural network structure. Then, the physical prior knowledge is integrated into the proposed network to limit the unreasonable results predicted in the feasible solution space to further improve the learning efficiency of the model. For specific research objects, the model can mine multi-dimensional features in water quality sequences at different depths, and learn the temporal features of the sequences hierarchically. In order to verify the performance of the proposed model, we apply the proposed model to the water quality dataset collected in Tanghe Reservoir for simulation. The results of the study demonstrate that our model is suitable for water quality prediction and has distinct advantages over traditional neural networks. Our model will make accurate predictions of future changes in water quality and provide technical support in the refined water quality automatic monitoring system.
更多查看译文
关键词
Time series predicting,Water quality,Deep neural network,Physics-constrained
AI 理解论文
溯源树
样例
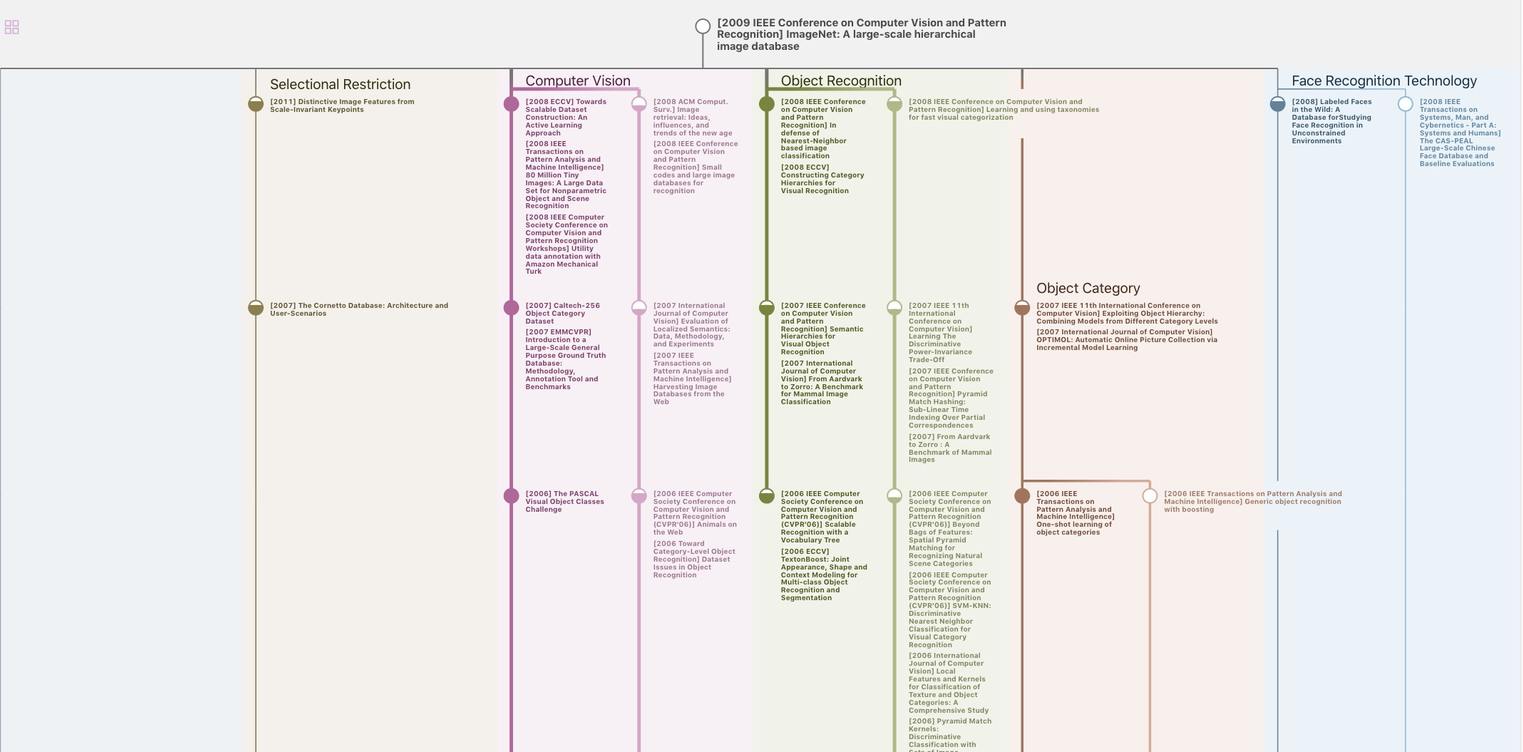
生成溯源树,研究论文发展脉络
Chat Paper
正在生成论文摘要