Deep learning-based data registration of melt-pool-monitoring images for laser powder bed fusion additive manufacturing
JOURNAL OF MANUFACTURING SYSTEMS(2023)
摘要
Melt-pool monitoring (MPM) has been widely used in the laser powder bed fusion (LPBF) additive manufac-turing process for process control and part quality prediction. Achieving this requires appropriate MPM-data alignments to the process parameters, part inspection, and testing data. This task is challenging when laser positioning is not synchronized with MPM-data acquisition. To address this challenge, this paper proposes a novel deep learning-based methodology to directly estimate melt pool positions in the machine-build coordinate system from the melt-pool images. First, we present two image-preprocessing methods, including image transformation and spatter reduction, using a stacked convolutional autoencoder to reduce noises from raw MPM images. Second, a convolutional neural network (CNN) based on self-supervised learning (SSL) is developed to identify the scan direction associated with an individual MPM image. Finally, Kalman Filtering is applied to estimate melt-pool positions given their scan directions. A case study based on an experimental build shows that the SSL-based CNN predicts the associated scan directions from the MPM images with an average error of about 1.37 degrees, and the Kalman filter effectively estimates the positions of melt pools with an average error of about 24.86 ism. The proposed methodology can benefit AM research and practice, enhancing data correlation and fusion, real-time defect prediction, and process quality control.
更多查看译文
关键词
Additive manufacturing, Data registration, Deep learning, Laser powder bed fusion, Melt pool monitoring
AI 理解论文
溯源树
样例
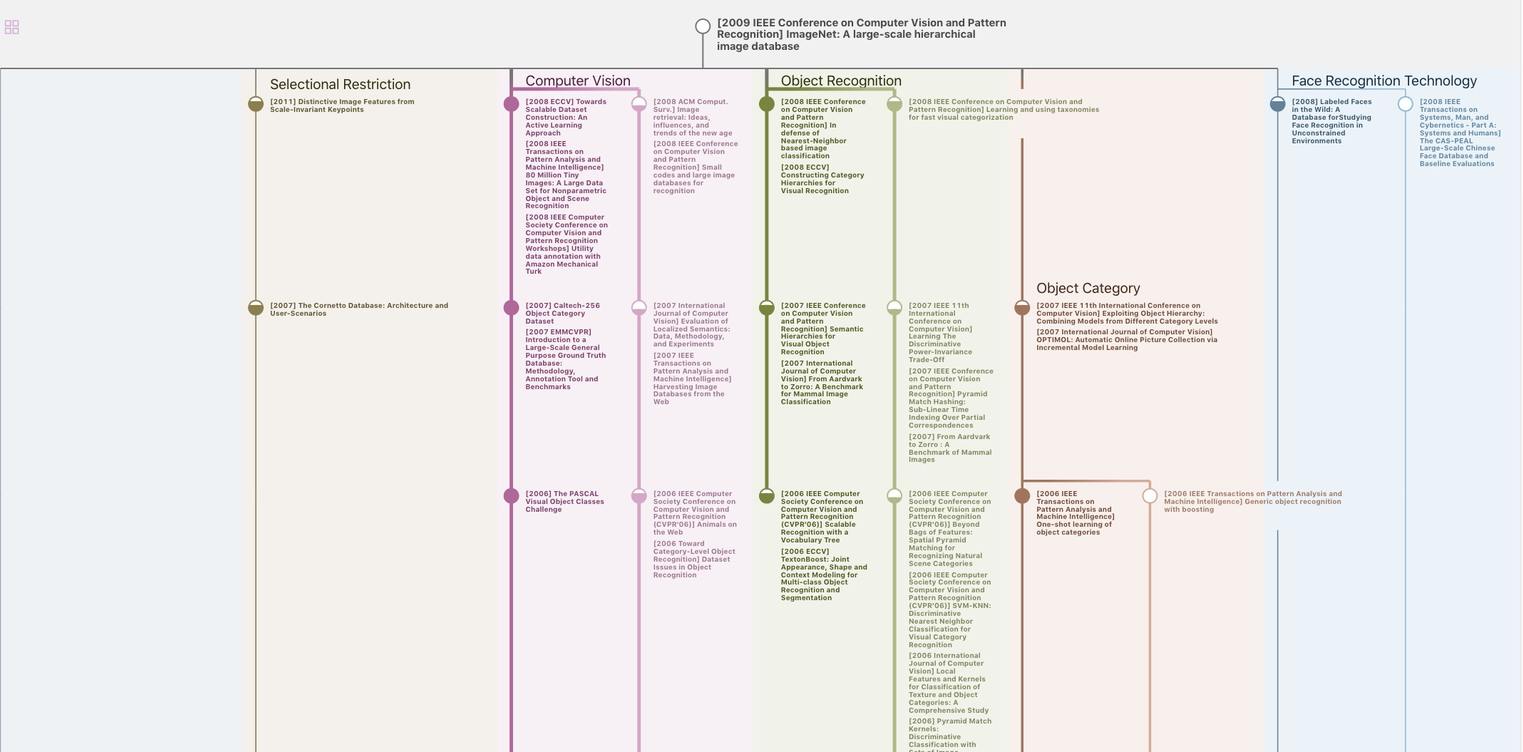
生成溯源树,研究论文发展脉络
Chat Paper
正在生成论文摘要