A few theoretical results for Laplace and arctan penalized ordinary least squares linear regression estimators
COMMUNICATIONS IN STATISTICS-THEORY AND METHODS(2023)
摘要
Two new non convex penalty functions - Laplace and arctan - were recently introduced in the literature to obtain sparse models for high-dimensional statistical problems. In this article, we study the theoretical properties of Laplace and arctan penalized ordinary least squares linear regression models. We first illustrate the near-unbiasedness of the non zero regression weights obtained by the new penalty functions, in the orthonormal design case. In the general design case, we present theoretical results in two asymptotic settings: (a) the number of features, p fixed, but the sample size, n ->infinity, and (b) both n and p tend to infinity. The theoretical results shed light onto the differences between the solutions based on the new penalty functions and those based on existing convex and non convex Bridge penalty functions. Our theory also shows that both Laplace and arctan penalties satisfy the oracle property. Finally, we also present results from a brief simulations study illustrating the performance of Laplace and arctan penalties based on the gradient descent optimization algorithm.
更多查看译文
关键词
linear regression estimators,laplace,few theoretical results
AI 理解论文
溯源树
样例
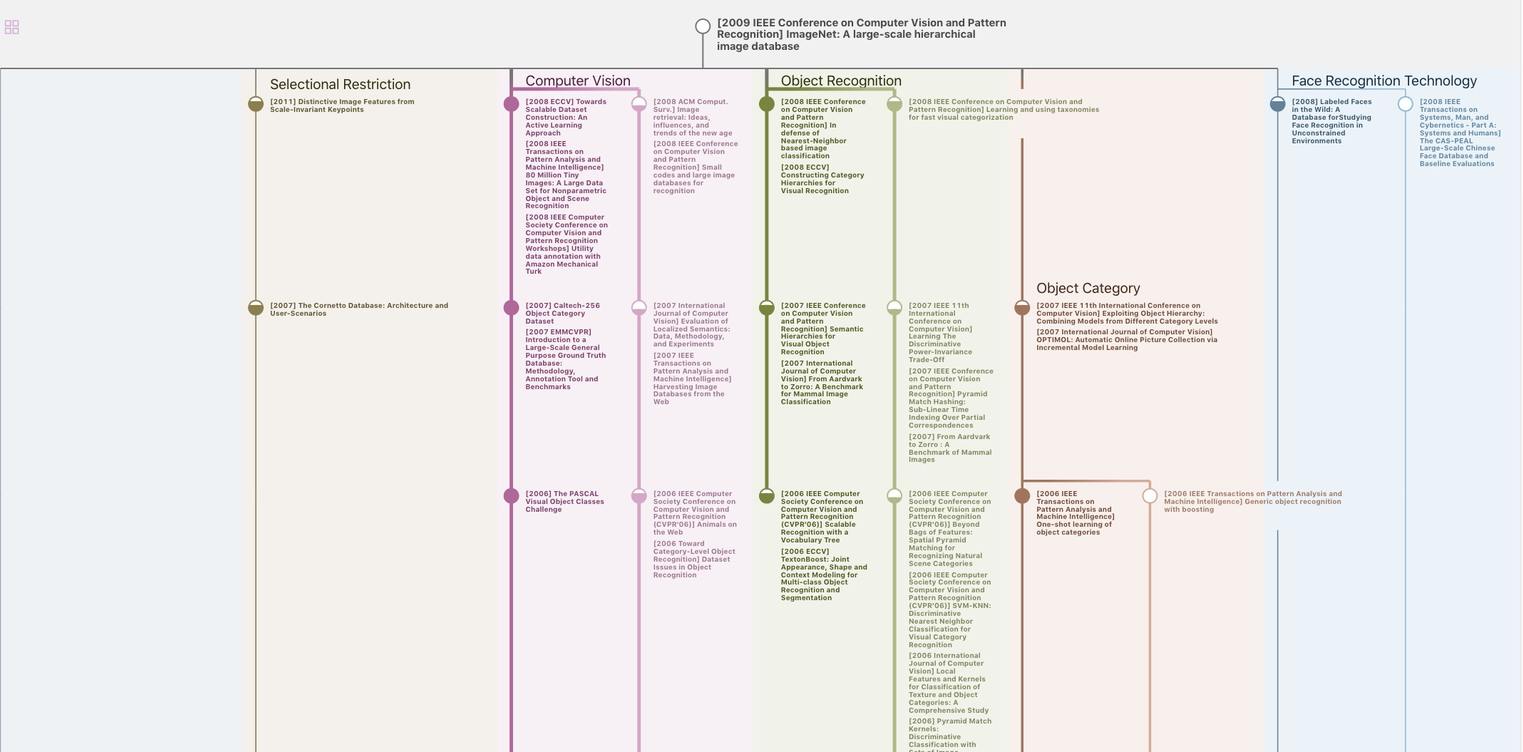
生成溯源树,研究论文发展脉络
Chat Paper
正在生成论文摘要