Kernelized Normalizing Constant Estimation: Bridging Bayesian Quadrature and Bayesian Optimization
AAAI 2024(2024)
摘要
In this paper, we study the problem of estimating the normalizing constant through queries to the black-box function f, which is the integration of the exponential function of f scaled by a problem parameter lambda. We assume f belongs to a reproducing kernel Hilbert space (RKHS), and show that to estimate the normalizing constant within a small relative error, the level of difficulty depends on the value of lambda: When lambda approaches zero, the problem is similar to Bayesian quadrature (BQ), while when lambda approaches infinity, the problem is similar to Bayesian optimization (BO). More generally, the problem varies between BQ and BO. We find that this pattern holds true even when the function evaluations are noisy, bringing new aspects to this topic. Our findings are supported by both algorithm-independent lower bounds and algorithmic upper bounds, as well as simulation studies conducted on a variety of benchmark functions.
更多查看译文
关键词
ML: Bayesian Learning,ML: Information Theory,ML: Kernel Methods,ML: Online Learning & Bandits
AI 理解论文
溯源树
样例
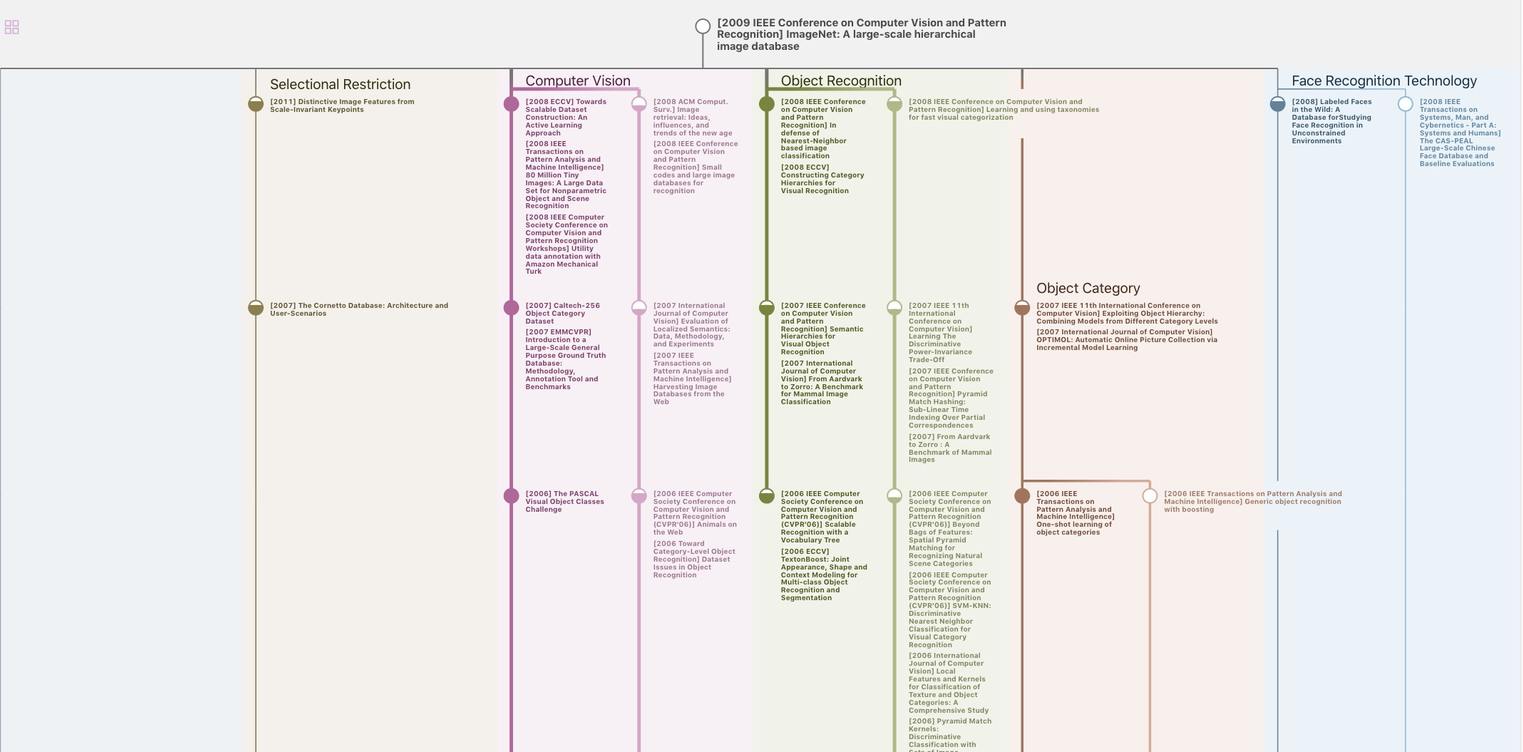
生成溯源树,研究论文发展脉络
Chat Paper
正在生成论文摘要