Electrocardiogram Analysis by Means of Empirical Mode Decomposition-Based Methods and Convolutional Neural Networks for Sudden Cardiac Death Detection
APPLIED SCIENCES-BASEL(2023)
摘要
Sudden cardiac death (SCD) is a global health problem, which represents 15-20% of global deaths. This type of death can be due to different heart conditions, where ventricular fibrillation has been reported as the main one. These cardiac alterations can be seen in an electrocardiogram (ECG) record, where the heart's electrical activity is altered. The present research uses these variations to be able to predict 30 min in advance when the SCD event will occur. In this regard, a methodology based on the complete ensemble empirical mode decomposition (CEEMD) method to decompose the cardiac signal into its intrinsic mode functions (IMFs) and a convolutional neural network (CNN) for automatic diagnosis is proposed. Results for the ensemble empirical mode decomposition (EEMD) method and the empirical mode decomposition (EMD) method are also compared. Results demonstrate that the combination of the CEEMD and the CNN is a potential solution for SCD prediction since 97.5% of accuracy is achieved up to 30 min in advance of the SCD event.
更多查看译文
关键词
electrocardiogram signal, sudden cardiac death, empirical mode decomposition, ensemble empirical mode decomposition, complete ensemble empirical mode decomposition, convolutional neural network
AI 理解论文
溯源树
样例
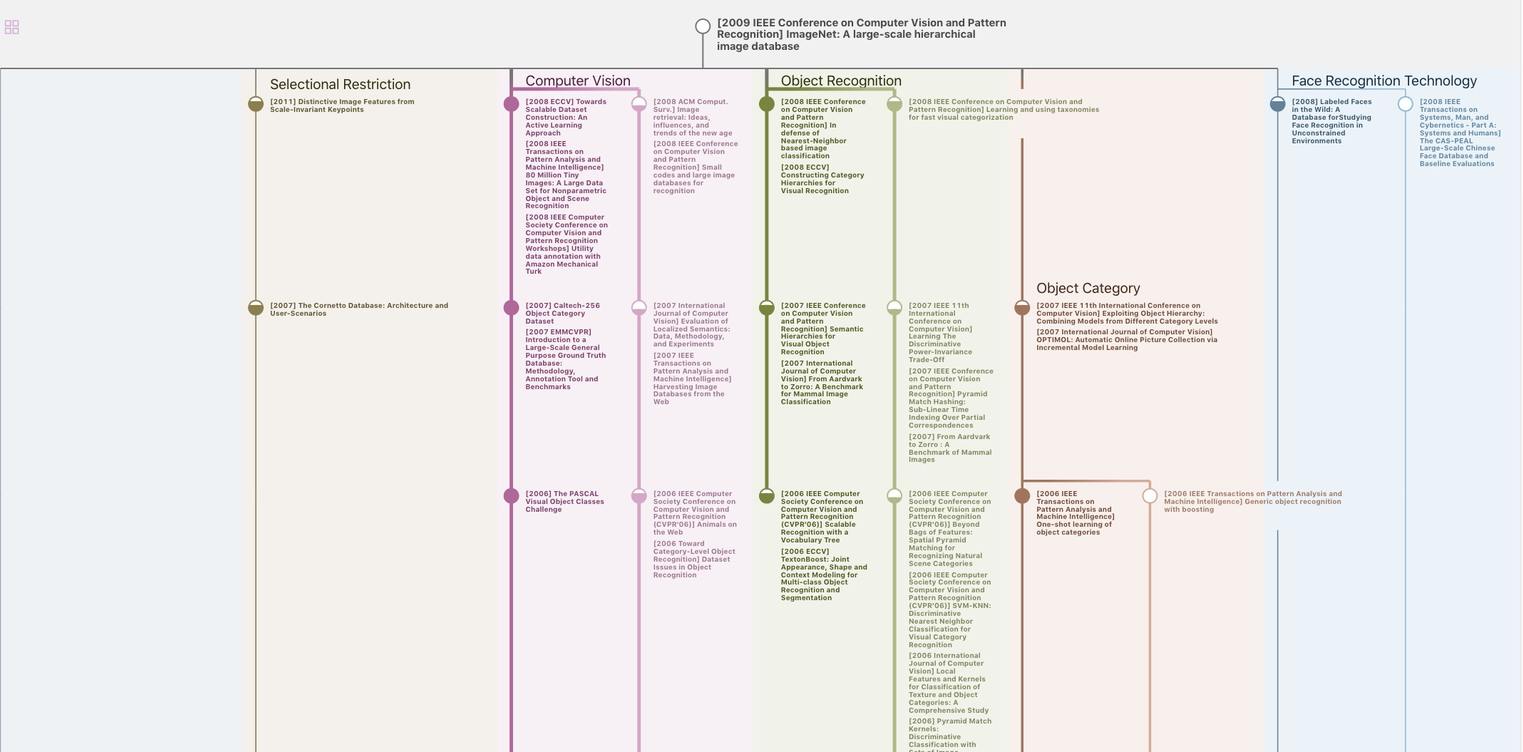
生成溯源树,研究论文发展脉络
Chat Paper
正在生成论文摘要