DA-FPN: Deformable Convolution and Feature Alignment for Object Detection
ELECTRONICS(2023)
摘要
This study sought to address the problem of the insufficient extraction of shallow object information and boundary information when using traditional FPN structures in current object detection algorithms, which degrades object detection accuracy. In this paper, a new FPN structure model, DA-FPN, is proposed. DA-FPN replaces the 1 x 1 convolution used in the conventional FPN structure for lateral connection with a 3 x 3 deformable convolution and adds a feature alignment module after the 2x downsampling operation used for lateral connection. This design allows the detection framework to extract more accurate information about the boundary of the object, particularly the boundary information of small objects. A bottom-up module was also added to incorporate the shallow information of the object more accurately into the high-level feature map, and a feature alignment module was added to the bottom-up module, thereby improving object detection accuracy. The experimental results show that DA-FPN can improve the accuracy of the single-stage object detection algorithms FoveaBox and GFL by 1.7% and 2.4%, respectively, on the MS-COCO dataset. This model was also found to improve the two-stage object detection algorithm SABL by 2.4% and offer higher small object detection accuracy and better robustness.
更多查看译文
关键词
deformable convolution, feature alignment, object detection
AI 理解论文
溯源树
样例
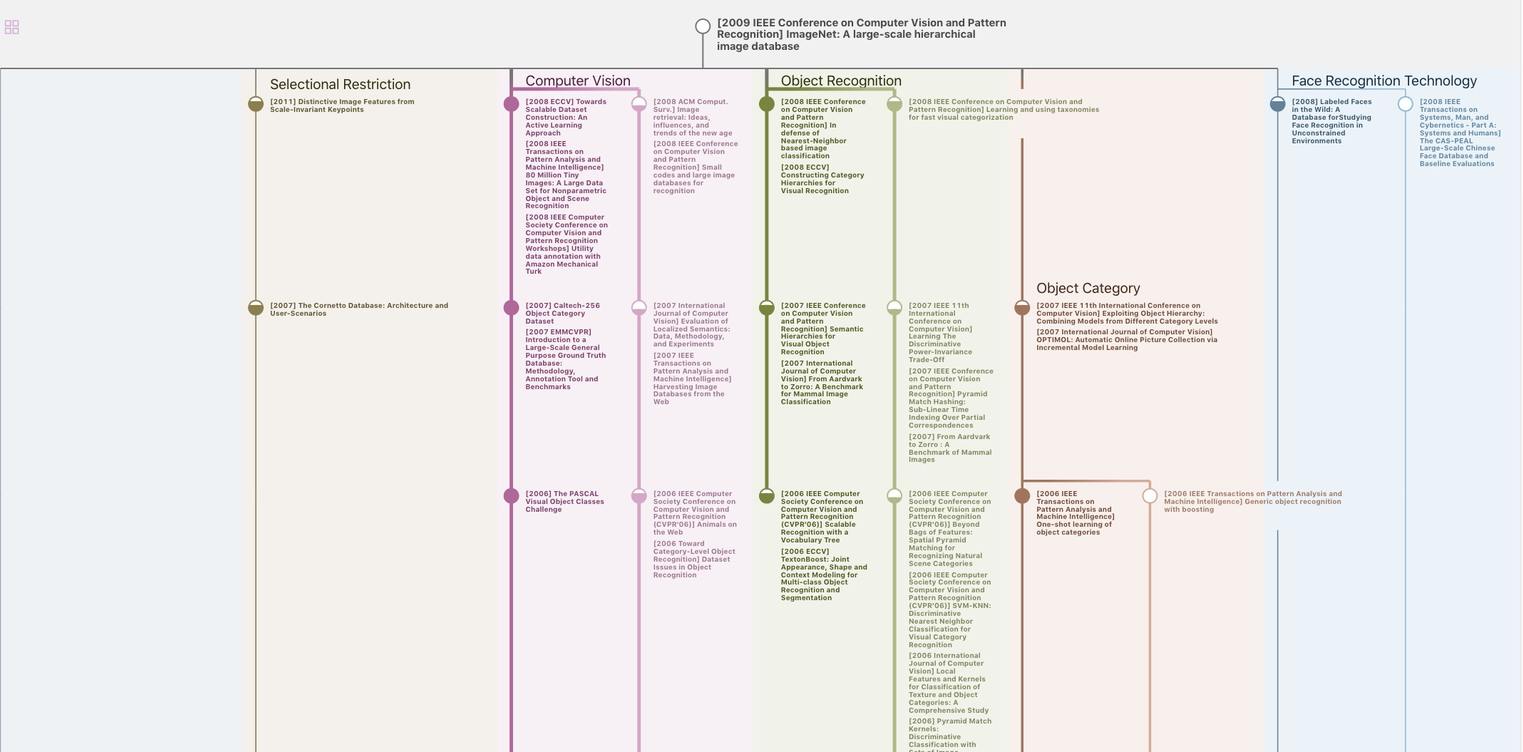
生成溯源树,研究论文发展脉络
Chat Paper
正在生成论文摘要