A joint local-global search mechanism for long-term tracking with dynamic memory network
EXPERT SYSTEMS WITH APPLICATIONS(2023)
摘要
Long-term tracking is a very popular tracking framework recently. Previous trackers mostly adopted a sliding window approach for global search, which can lead to significant time consumption. In addition, frequent appearance variations can make the tracking model lack long term adaptability. To solve the above issues, this paper proposes a joint local-global search mechanism for long-term tracking with dynamic memory network (LGST), which uses a global detector to generate "high-quality"region proposals and a dynamic memory network to store reliable template samples. Specifically, we first propose a novel global re-detection module that employs learning target channel-aware representation (TCA-R) to guide the region proposal network (RPN) to perform global search and cover the region of the target of interest to cope with the target disappearance and reappearance. Then, for the local tracker, a dynamic memory network is designed to collect the updated templates at different time periods and store reliable template samples. More importantly, we connect and feed the collected template features into the transformer encoder to generate the attention -enhanced features. Finally, we adopt a verifier to determine whether the tracked object exists or not, and dynamically switch between local search and global search mechanisms in the next image frame to form a long-term tracking framework. Experimental results on VOT-LT2018, VOT-LT2019, LaSOT, TLP, OTB2015, and UAV123 benchmarks show that our proposed tracker achieves comparable performance to state-of-the-art tracking algorithms.
更多查看译文
关键词
local–global search mechanism,dynamic,memory,long-term
AI 理解论文
溯源树
样例
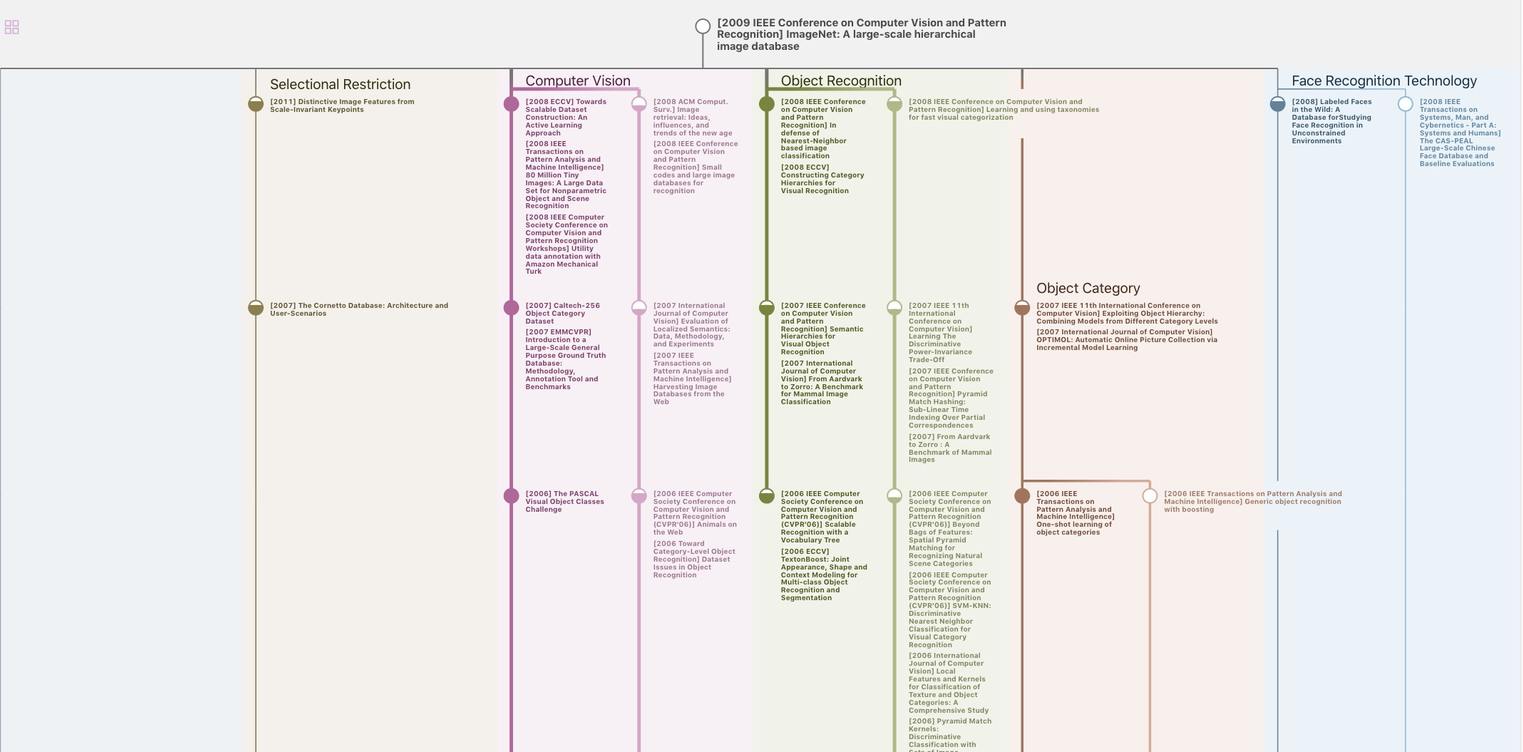
生成溯源树,研究论文发展脉络
Chat Paper
正在生成论文摘要