Bias correction for nonignorable missing counts of areal HIV new diagnosis
STAT(2023)
摘要
Public health data, such as HIV new diagnoses, are often left-censored due to confidentiality issues. Standard analysis approaches that assume censored values as missing at random often lead to biased estimates and inferior predictions. Motivated by the Philadelphia areal counts of HIV new diagnosis for which all values less than or equal to 5 are suppressed, we propose two methods to reduce the adverse influence of missingness on predictions and imputation of areal HIV new diagnoses. One is the likelihood-based method that integrates the missing mechanism into the likelihood function, and the other is a nonparametric algorithm for matrix factorization imputation. Numerical studies and the Philadelphia data analysis demonstrate that the two proposed methods can significantly improve prediction and imputation based on left-censored HIV data. We also compare the two methods on their robustness to model misspecification and find that both methods appear to be robust for prediction, while their performance for imputation depends on model specification.
更多查看译文
关键词
areal hiv,diagnosis
AI 理解论文
溯源树
样例
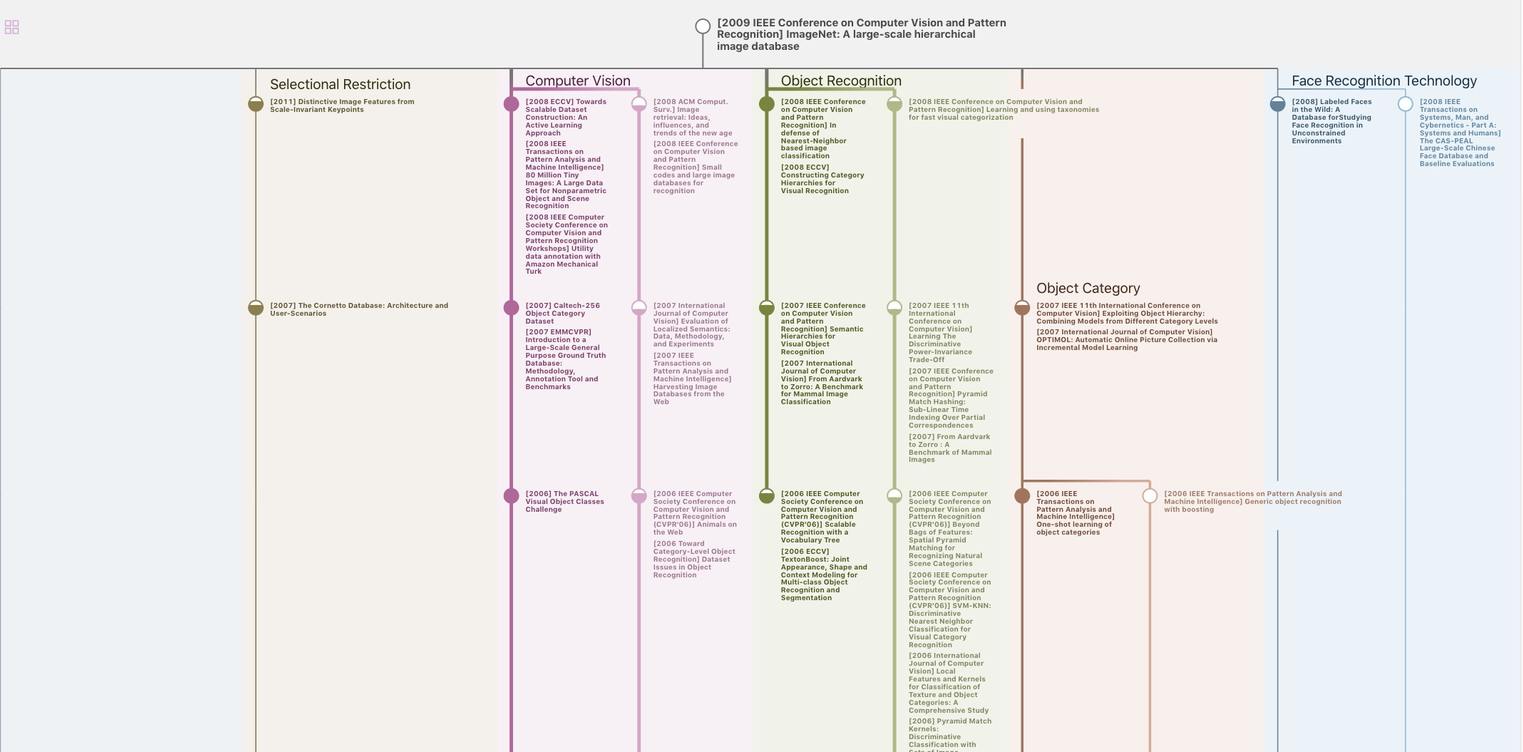
生成溯源树,研究论文发展脉络
Chat Paper
正在生成论文摘要