SepFE: Separable Fusion Enhanced Network for Retinal Vessel Segmentation
CMES-COMPUTER MODELING IN ENGINEERING & SCIENCES(2023)
摘要
The accurate and automatic segmentation of retinal vessels from fundus images is critical for the early diagnosis and prevention of many eye diseases, such as diabetic retinopathy (DR). Existing retinal vessel segmentation approaches based on convolutional neural networks (CNNs) have achieved remarkable effectiveness. Here, we extend a retinal vessel segmentation model with low complexity and high performance based on U-Net, which is one of the most popular architectures. In view of the excellent work of depth-wise separable convolution, we introduce it to replace the standard convolutional layer. The complexity of the proposed model is reduced by decreasing the number of parameters and calculations required for the model. To ensure performance while lowering redundant parameters, we integrate the pre-trained MobileNet V2 into the encoder. Then, a feature fusion residual module (FFRM) is designed to facilitate complementary strengths by enhancing the effective fusion between adjacent levels, which alleviates extraneous clutter introduced by direct fusion. Finally, we provide detailed comparisons between the proposed SepFE and U-Net in three retinal image mainstream datasets (DRIVE, STARE, and CHASEDB1). The results show that the number of SepFE parameters is only 3% of U-Net, the Flops are only 8% of U-Net, and better segmentation performance is obtained. The superiority of SepFE is further demonstrated through comparisons with other advanced methods.
更多查看译文
关键词
Retinal vessel segmentation, U-Net, depth-wise separable convolution, feature fusion
AI 理解论文
溯源树
样例
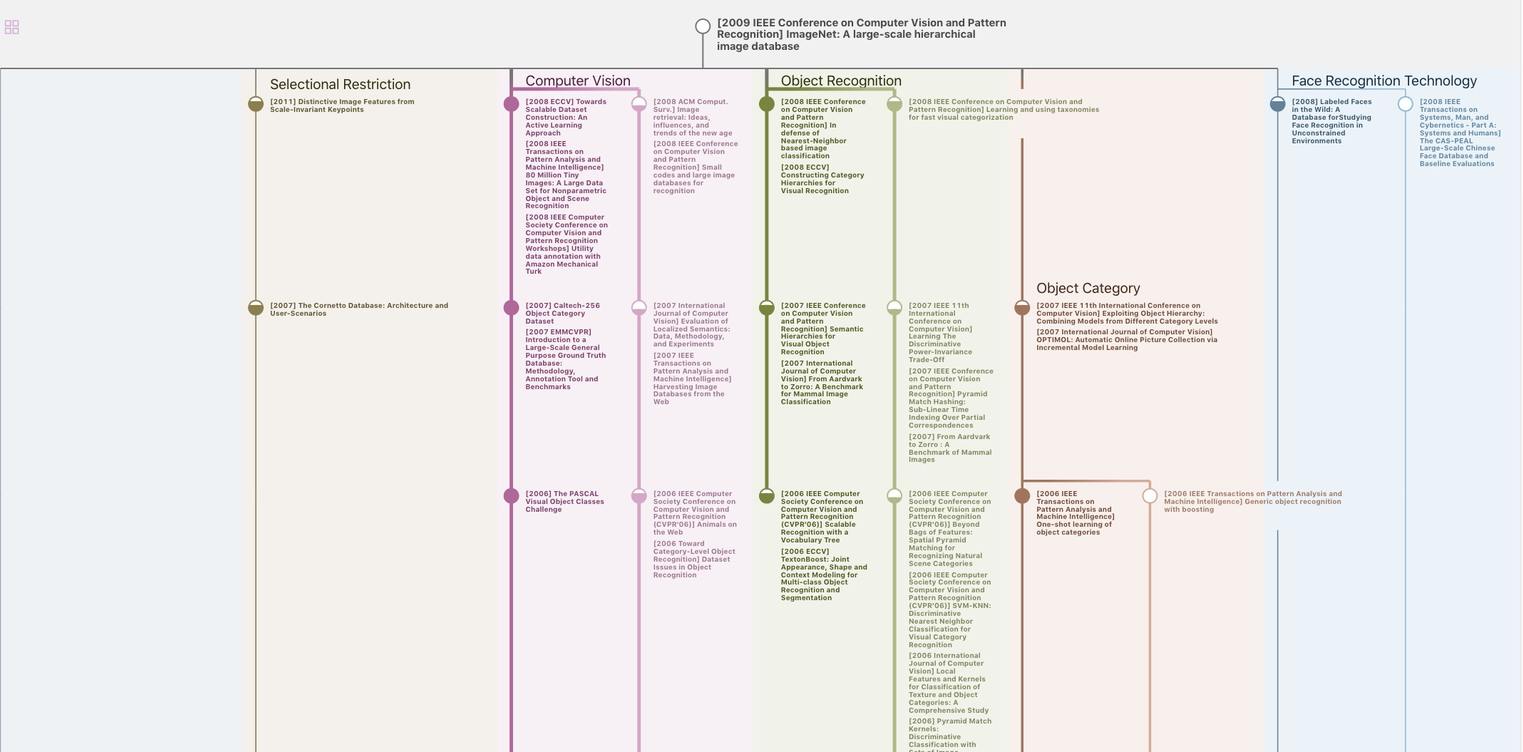
生成溯源树,研究论文发展脉络
Chat Paper
正在生成论文摘要