Trapezoid-structured LSTM with segregated gates and bridge joints for video frame inpainting
VISUAL COMPUTER(2023)
摘要
This work considers the video frame inpainting problem, where several former and latter frames are given, and the goal is to predict the middle frames. The state-of-the-art solution has applied bidirectional long short-term memory (LSTM) networks, which has a spatial-temporal mismatch problem. In this paper, we propose a trapezoid-structured LSTM architecture called T-LSTM-sbm for video frame inpainting with three designs: (i) segregated spatial-temporal gates, (ii) bridge joints, and (iii) multi-kernel LSTM. To prevent the spatial-temporal mismatch problem, while features are being passed through multi-layered LSTM nodes, the trapezoid structure reduces its number of LSTM nodes by two after each layer. This makes the model converge to the inpainted results more effectively. The separated temporal and spatial gates design can learn better spatial and temporal features by using individual gates. To relieve the information loss problem during the convergence of the trapezoidal layers, we use bridge joints among layers to better preserve useful information. The multiple kernels in LSTM are to enable extracting multi-scale information flows. T-LSTM-sbm is proved to outperform the state-of-the-art solutions in peak signal-to-noise ratio (PSNR) and structural similarity (SSIM) on three common datasets, KTH Action, HMDB-51, and UCF-101.
更多查看译文
关键词
Bridge joints,Multi-kernel LSTM,Segregated spatial-temporal gates,Trapezoid-structured LSTM,Video frame inpainting
AI 理解论文
溯源树
样例
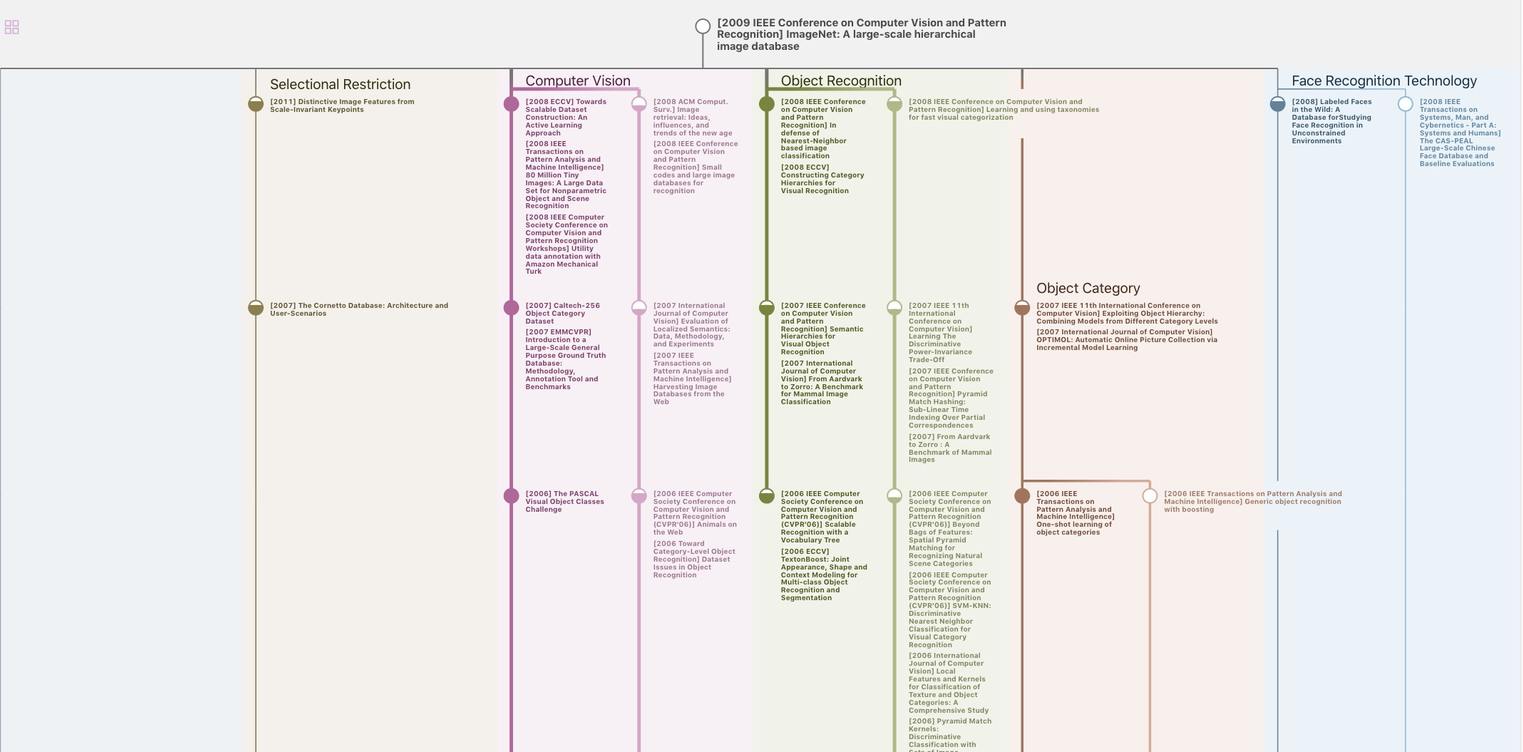
生成溯源树,研究论文发展脉络
Chat Paper
正在生成论文摘要