Deep learning based registration for head motion correction in positron emission tomography as a strategy for improved image quantification
FRONTIERS IN PHYSICS(2023)
摘要
Objectives: Positron emission tomography (PET) is affected by various kinds of patient movement during a scan. Frame-by-frame image registration is one of the most practiced motion correction techniques. In recent years, deep learning has shown a remarkable ability to quickly and accurately register images once trained. This paper studies the feasibility of using a deep learning framework to correct 3D positron emission tomography image volumes for head motion in routine positron emission tomography imaging to improve quantification in motion impacted data. Materials and Methods: A neural network was trained with 3D positron emission tomography image volumes in an unsupervised manner to predict transformation parameters required to perform image registration. A multi-step convolutional neural network (CNN) was combined with a spatial transform layer. Pairs of target and source images were used as input to the network. To prepare the training dataset, a previously published TOF-PEPT algorithm was applied to automatically detect static frames where the patient remained in a relatively steady position and transitional frames where they underwent abrupt motion. A single image volume was reconstructed for each static frame. The image reconstructed from the first static frame served as the target image with images from subsequent static frames being used as source images. The trained neural network predicted transformation parameters that could be used to perform frame-by-frame image-based motion correction but also enabled raw listmode positron emission tomography data correction where individual line-of-responses were repositioned. Line profiles and ROIs were drawn across the reconstructed image volumes to compare performance and quantitative results between standard registration tools and the deep learning technique. Corrected volumes were further compared to motion free images quantitatively using Dice indices. Results: In total, one hundred 3D positron emission tomography image volumes were used to train the network. Cross-validation was carried out using a 4:1 ratio for the training and test data. A conventional algorithm for affine registration from the Advanced Normalization Tools (ANTs) software package served as a baseline. To evaluate the correction performance, the mean Dice index and standardized uptake value (SUV) were used. Application of the algorithm to clinical data showed good performance with respect to registration accuracy as well as processing time. The neural network yielded a mean Dice index of similar to 0.87 which was similar to the advanced Normalization Tools algorithm and did so similar to 3x faster using a multi-core CPU and similar to 20x faster with a GPU. Standardized uptake value analysis showed that quantitative results were 30%-60% higher in the motion-corrected images, and the neural network performed better than or close to the advanced Normalization Tools. Conclusion: The aim of this work was to study the quantitative impact of using a data-driven deep learning motion correction technique for positron emission tomography data and assess its performance. The results showed the technique is capable of producing high quality registrations that compensate for patient motion that occurs during a scan and improve quantitative accuracy.
更多查看译文
关键词
positron emission tomography (PET),head motion correction,positron emission particle tracking (PEPT),time-of-flight (TOF),deep learning,image registration
AI 理解论文
溯源树
样例
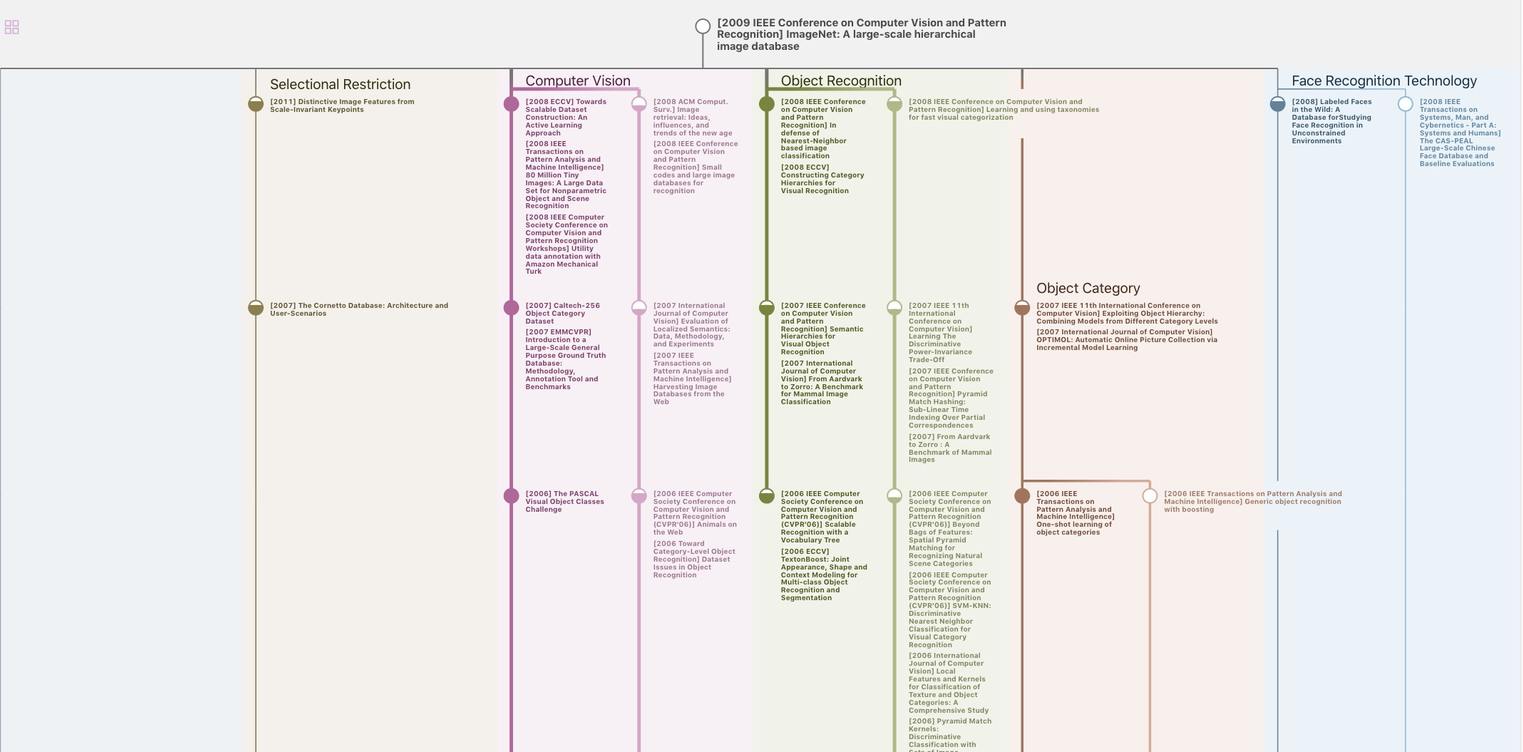
生成溯源树,研究论文发展脉络
Chat Paper
正在生成论文摘要