A deep learning model developed for sleep apnea detection: A multi-center study
BIOMEDICAL SIGNAL PROCESSING AND CONTROL(2023)
摘要
Objective. Manual detection of obstructive sleep apnea (OSA) is a challenging task for many reasons such as time-consuming and subjective. This study aimed to develop a deep learning model for automatically detecting abnormal sleep respiratory episodes in patients with OSA and to explore the performance differences between different sleep centers and different kind of sleep monitoring devices. Methods. The proposed model which comprised branch convolution, separable convolution, and self-attention mechanisms, took minute-to-minute raw electroencephalography (EEG) and oral-nasal airflow signals as its input. Results. The results of five-fold cross-validation in 50 clinical polysomnography recordings evidenced that the average accuracy was 0.877, with a sensitivity and specificity of 0.837, and 0.873, respectively. The proposed method achieved almost perfect agreement with manual scoring method according to the apnea-hypopnea index. In addition, experiments revealed that the combination of EEG and oral-nasal airflow signals could improve the accuracy of approximately 10% relative to oral-nasal airflow signals. Furthermore, a significant difference (P < 0.05) in performance be-tween different sleep centers and different sleep-monitoring devices was also observed. Conclusion and signif-icance. The proposed model achieved a comparably high performance in three different sleep centers, indicating its feasibility and robustness. This study suggested the reliable and simple alternative to clinical OSA screening. The model and data can be found at https://github.com/Lifanfan-dlut/OSA-detection.
更多查看译文
关键词
Electroencephalography, Multi-center, Obstructive sleep apnea, Deep learning
AI 理解论文
溯源树
样例
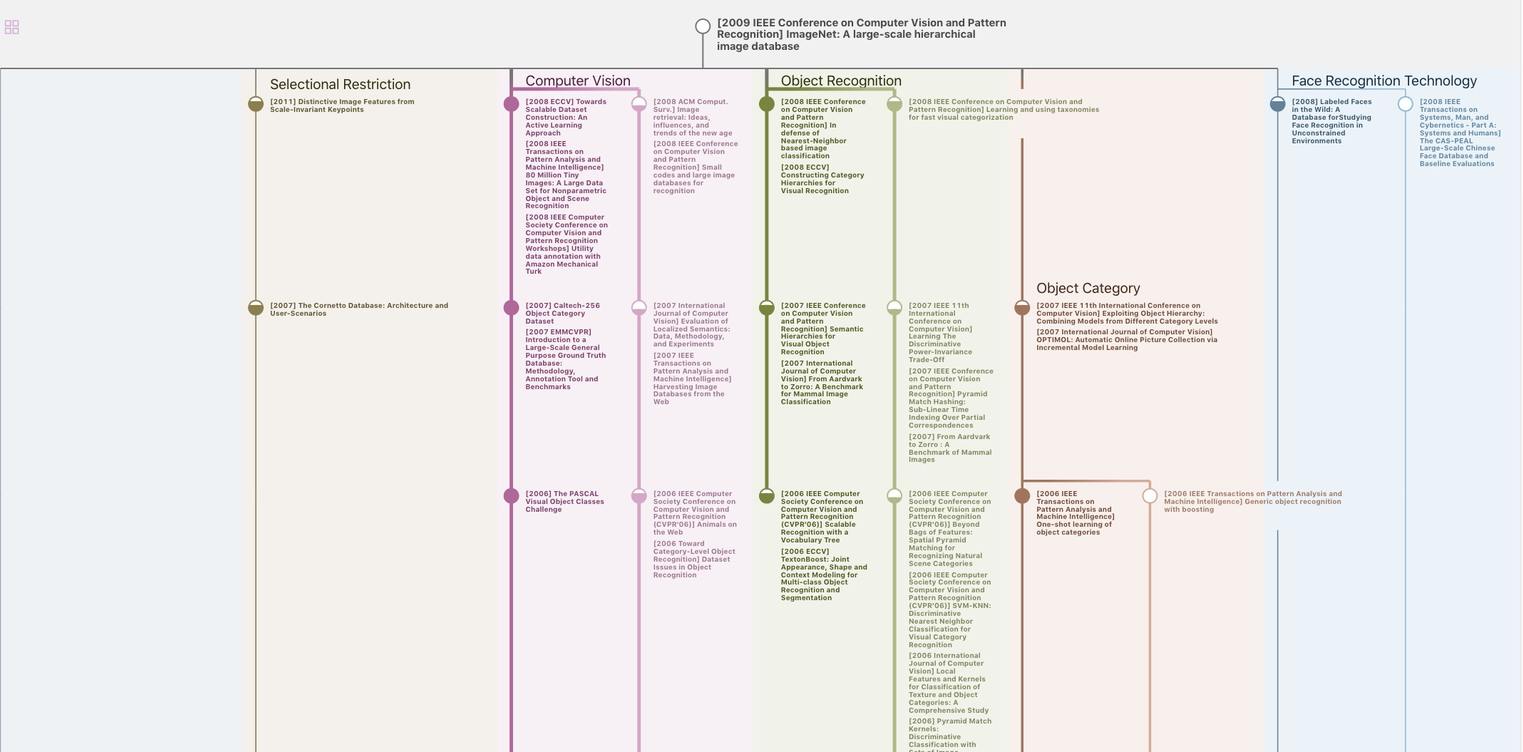
生成溯源树,研究论文发展脉络
Chat Paper
正在生成论文摘要