A distribution information sharing federated learning approach for medical image data
COMPLEX & INTELLIGENT SYSTEMS(2023)
摘要
In recent years, federated learning has been believed to play a considerable role in cross-silo scenarios (e.g., medical institutions) due to its privacy-preserving properties. However, the non-IID problem in federated learning between medical institutions is common, which degrades the performance of traditional federated learning algorithms. To overcome the performance degradation problem, a novelty distribution information sharing federated learning approach (FedDIS) to medical image classification is proposed that reduce non-IIDness across clients by generating data locally at each client with shared medical image data distribution from others while protecting patient privacy. First, a variational autoencoder (VAE) is federally trained, of which the encoder is uesd to map the local original medical images into a hidden space, and the distribution information of the mapped data in the hidden space is estimated and then shared among the clients. Second, the clients augment a new set of image data based on the received distribution information with the decoder of VAE. Finally, the clients use the local dataset along with the augmented dataset to train the final classification model in a federated learning manner. Experiments on the diagnosis task of Alzheimer’s disease MRI dataset and the MNIST data classification task show that the proposed method can significantly improve the performance of federated learning under non-IID cases.
更多查看译文
关键词
Federated learning,Distribution information sharing,Image classification,Non-IID
AI 理解论文
溯源树
样例
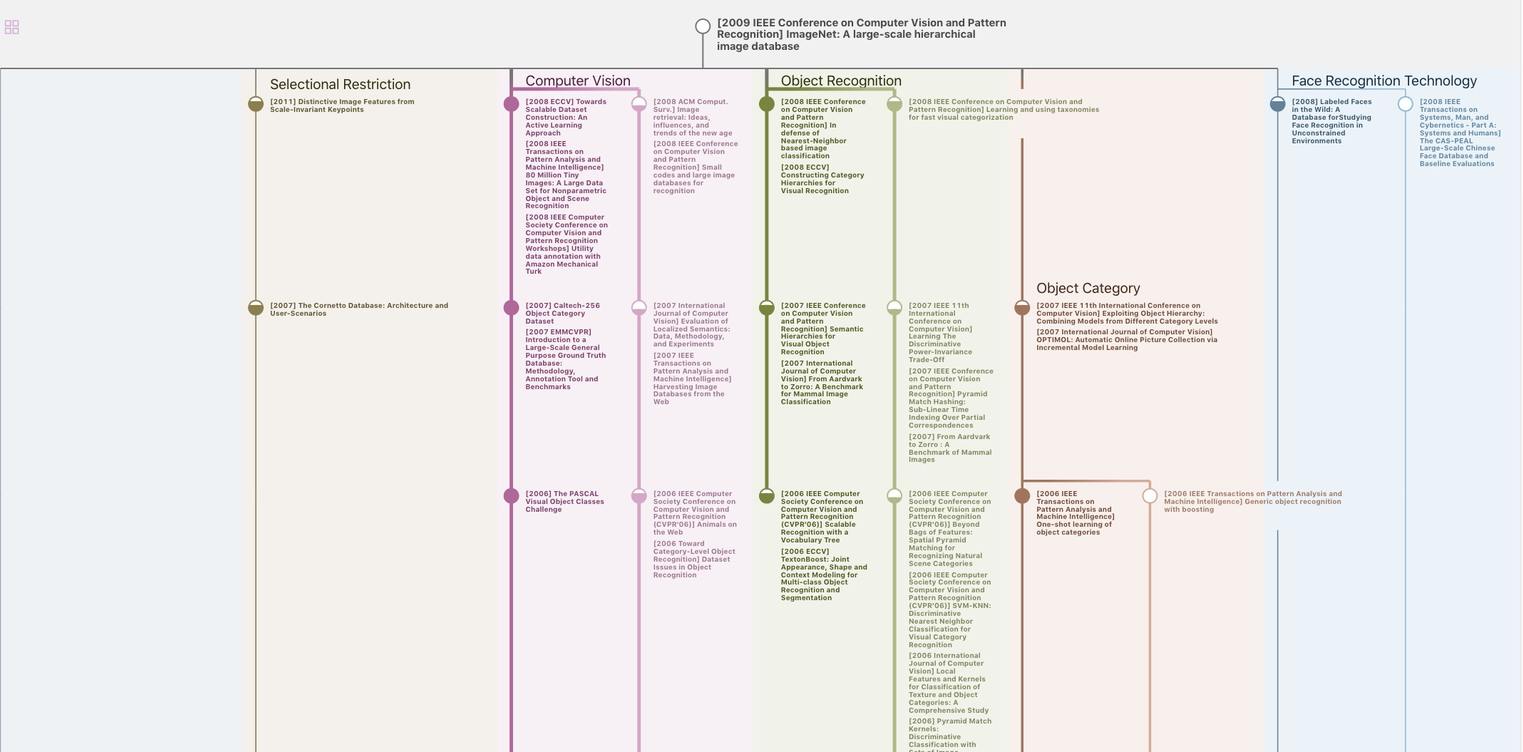
生成溯源树,研究论文发展脉络
Chat Paper
正在生成论文摘要