The adaptive constant false alarm rate for sonar target detection based on back propagation neural network access
IET SIGNAL PROCESSING(2023)
摘要
With oceanic reverberation and a large amount of data being the main sources of interference for underwater acoustic target detection, it is difficult to obtain a more robust detection performance by relying on the traditional constant false alarm rate (CFAR) detection method. An adaptive sonar CFAR detection method based on a back propagation (BP) neural network is proposed. The method combines the artificial intelligence algorithm and the traditional detection algorithm, and uses the classification ability of the algorithm to select the detection algorithm, which can effectively improve the adaptation ability of the algorithm and the environment and the false alarm control ability. The method combines the artificial intelligence algorithm and the traditional detection algorithm, and uses the classification ability of the algorithm to select the detection algorithm, which can effectively improve the adaptation ability of the algorithm and the environment and the false alarm control ability. This method uses a BP neural network to train the target echo signal to complete the clutter background classification and establish the clutter background recognition classification set. According to the output result of each classification, the best CFAR detector is selected from four CA/SO/GO/OS-CFAR detectors to detect the target. The simulation results show the detection performance of the proposed method in a uniform environment, a multi-target environment, and a clutter edge environment. The results show that the environment adaptability is strong for different clutter backgrounds, which further improves the control ability of false alarms under a non-uniform background.
更多查看译文
关键词
ocean waves, signal detection, signal processing
AI 理解论文
溯源树
样例
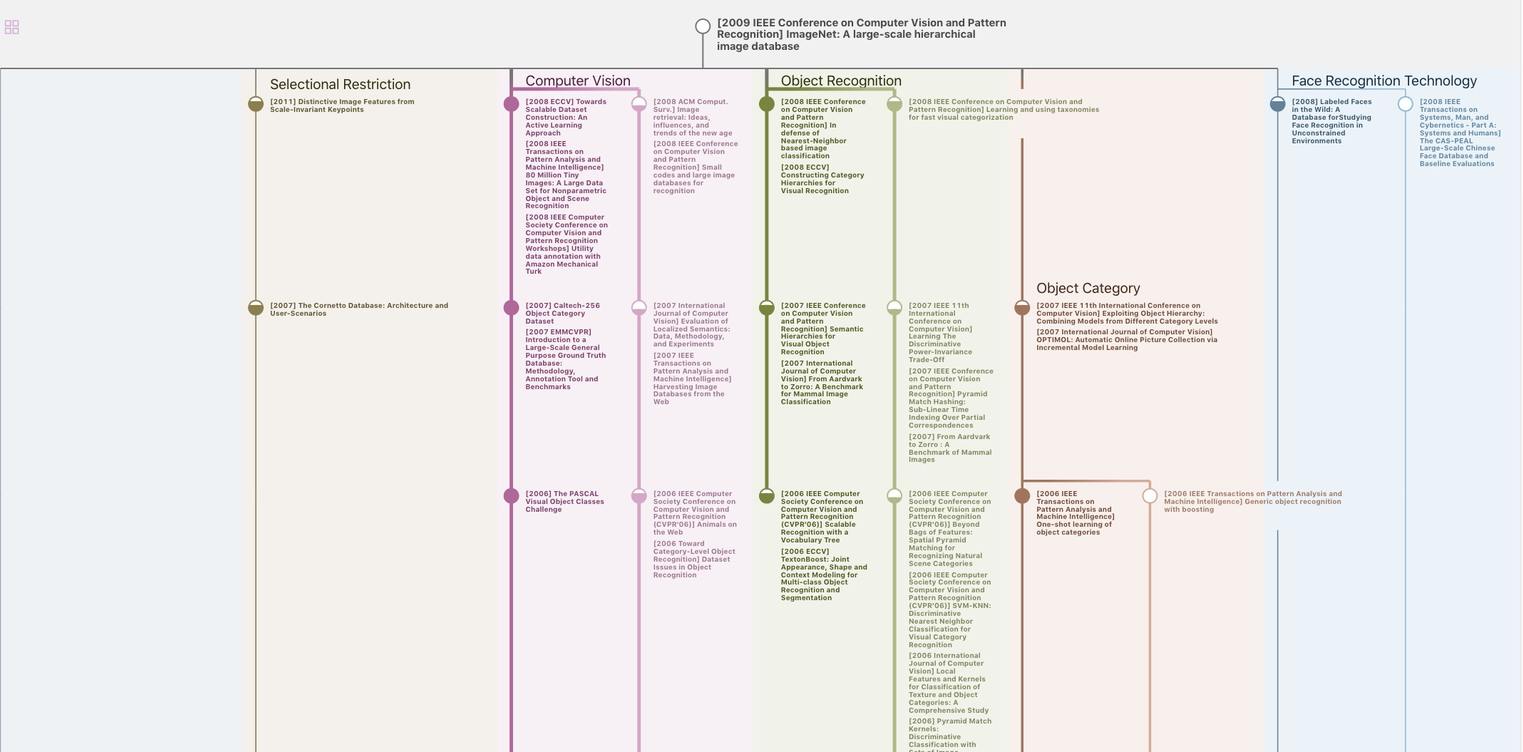
生成溯源树,研究论文发展脉络
Chat Paper
正在生成论文摘要