A Physics-Guided MFL Deformed Defect Recovery Method
IEEE TRANSACTIONS ON AUTOMATION SCIENCE AND ENGINEERING(2023)
摘要
Magnetic flux leakage (MFL) testing and analysis is an effective non-destructive testing (NDT) method of pipeline health. The health status of pipelines is evaluated by analyzing MFL signals, especially the defect analysis. However, complex operating conditions and equipment deviations may cause deformation on the defects. Availability and integrity of the MFL defects are the keys to accurate signal processing. Due to the limited interpretability of abstract networks and insufficient feature extraction, it is hard to accurately recover the deformed defects. Considering the problems above, an MFL deformed defect recovery method with the guidance of physical features is proposed. The proposed method integrates a deep feature extraction model into a sparse autoencoder, whose parameters are guided by the combination of MSE loss and physics loss. First, the deep feature extraction model fully extracts the abstract features, which contains more information on deformed defects. Second, the sparse penalty factor in the sparse autoencoder reduces the feature redundancy of deformed defects. Third, the physical features are extracted to guide the MFL deformed defect recovery relying on the MFL mechanism for the first time, which enhances the robustness and accuracy of the network. Finally, several comparison experiments have been conducted on measured and simulated MFL defects. The results show that the proposed method is effective in deformed defect recovery. Note to Practitioners-The motivation of this paper is a practical problem of MFL deformed defect recovery. The deformation on MFL defects affects defect inversion and evaluation seriously, which causes great potential harm to pipeline safety transmission. In this paper, compared with traditional neural network-based methods, physical features based on MFL mechanism are extracted to guide the parameter update. In addition, a pre-trained model replaces regular convolutional layers to extract features sufficiently, while a sparse factor is applied to reduce redundant features. The proposed method not only utilizes deep features from deep networks, but also increases the guidance of physical features, so that the algorithm is more robust and accurate. The experiment results show that our proposed method is more effective than the comparative methods on MFL simulated and measured defects. In a word, the proposed method has strong theoretical research and practical value.
更多查看译文
关键词
Deformed defect recovery,physical features,sparse autoencoder,pre-trained model,MFL
AI 理解论文
溯源树
样例
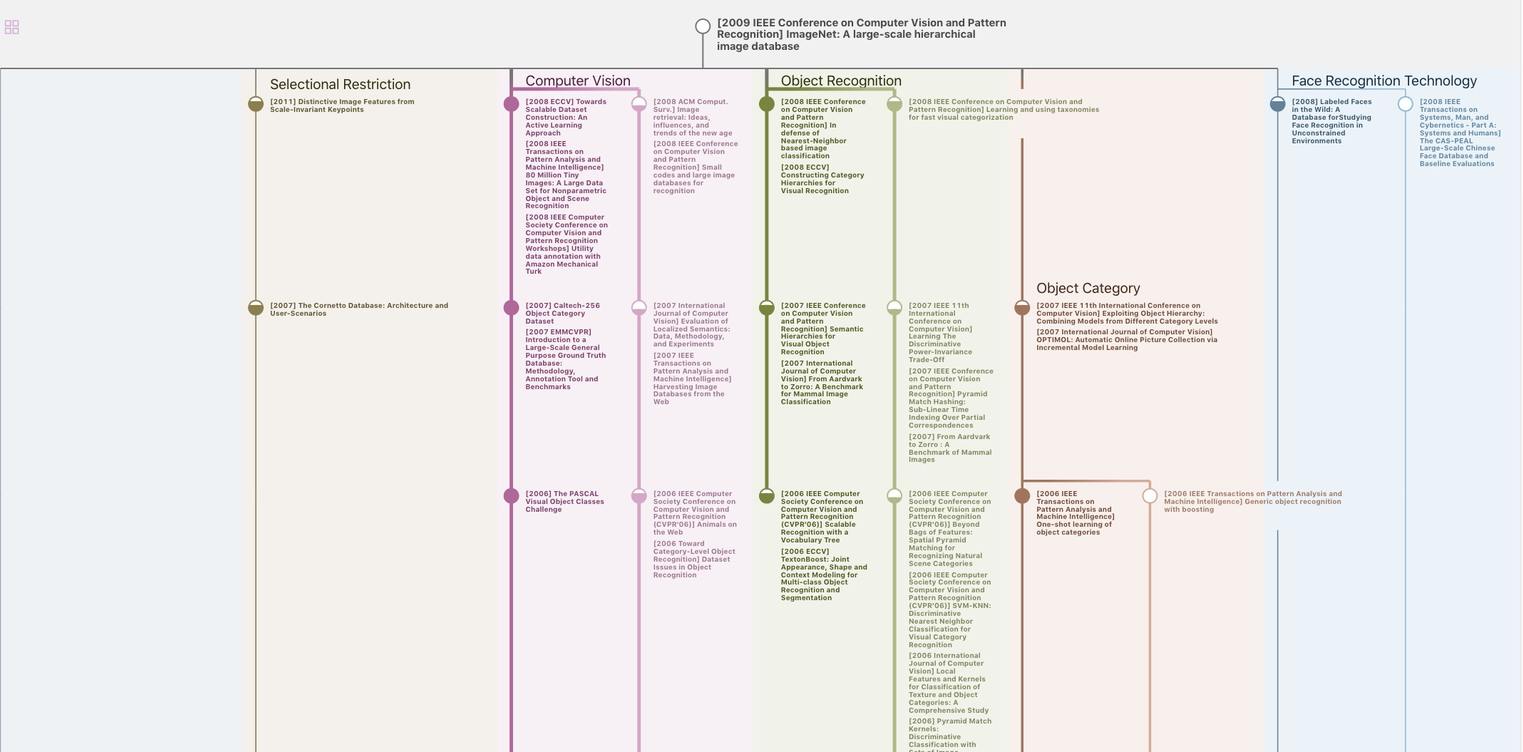
生成溯源树,研究论文发展脉络
Chat Paper
正在生成论文摘要