RePGAN: image inpainting via residual partial connection and mask discriminator
INTERNATIONAL JOURNAL OF MACHINE LEARNING AND CYBERNETICS(2023)
摘要
In recent years, image inpainting approaches have shown remarkable improvements by employing encoder-decoder-based convolutional neural networks (CNNs). An important issue is that texture information is lost during the convolution procedure. Previous works addressed this problem by employing skip connection strategies, which deliver encoder features to a symmetrical decoder, neglecting the fact that the shallow encoder layers contain both zeros and noise. In this paper, we propose a novel strategy that applies a residual partial module (RePM) to divide the feature maps into two branches (i.e., identity and residual branches) to replace skip connections. The identity branch retains the texture information, and the residual branch eliminates noise. Moreover, we propose a mask discriminator that judges the ground truth of the missing area. Experiments on benchmark datasets show the effectiveness of our method in producing coherent images, with our approach performing favourably against existing approaches.
更多查看译文
关键词
residual partial connection,mask
AI 理解论文
溯源树
样例
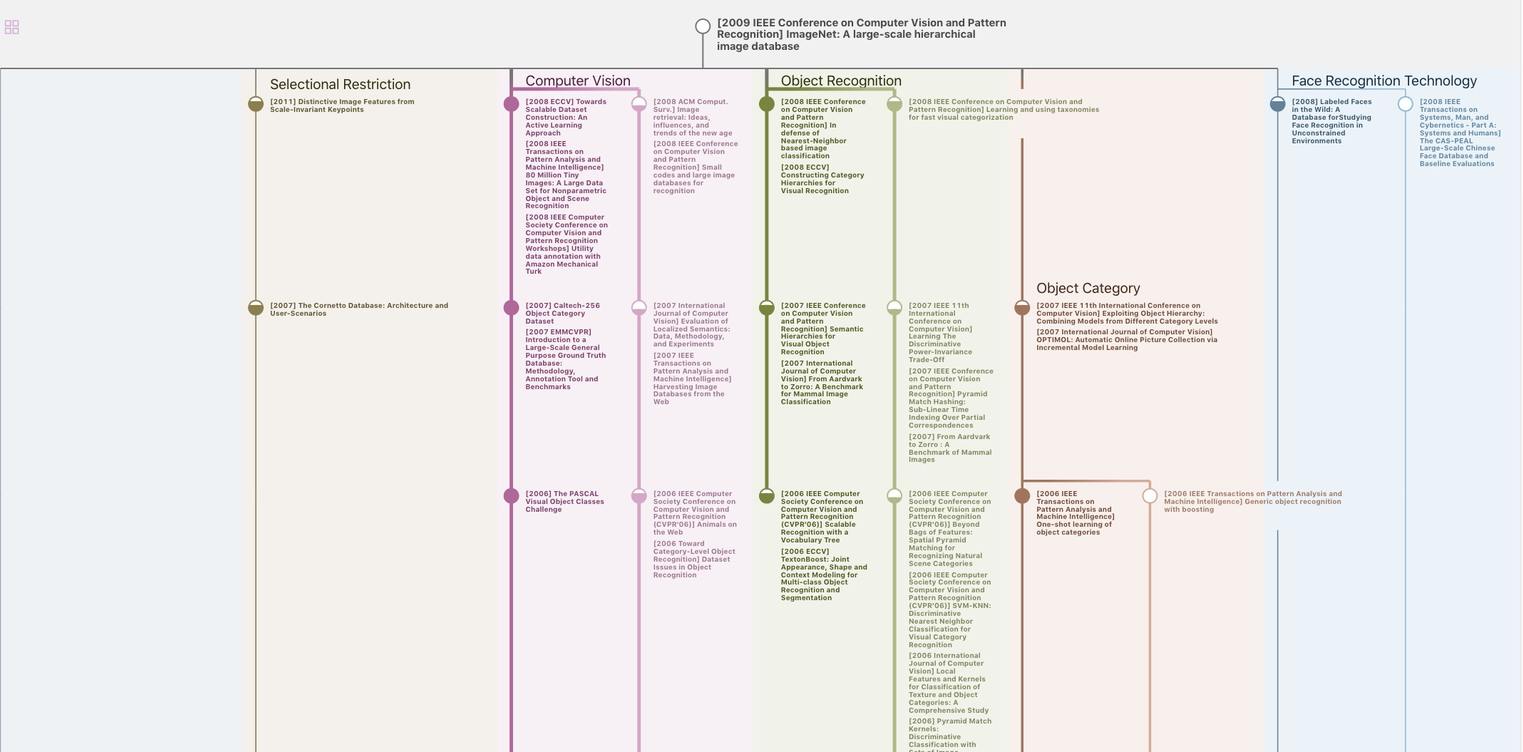
生成溯源树,研究论文发展脉络
Chat Paper
正在生成论文摘要