Machine learning-based accelerated design of fluorphlogopite glass ceramic chemistries with targeted hardness
JOURNAL OF THE AMERICAN CERAMIC SOCIETY(2023)
摘要
In this work, we develop and employ an accelerated design strategy using a machine learning algorithm to overcome the challenges for designing a new machinable glass ceramic. The trained machine learning model predicts the specific hardness value for numerous possibilities of processing conditions such as growth temperature and time. We report that the optimized growth parameters of 1200 degrees C and 5 h achieve the highest machinability of 0.4 in the glass ceramic. Furthermore, we predicted the eight most promising candidates containing specific ratios of silicon, magnesium, aluminum, lithium, boron, potassium, barium, and oxygen. Combining machine learning with experimental data enables a systemic and rapid design of a ceramic material while capturing the underlying physics represented in the experimental data.
更多查看译文
关键词
glass ceramics, hardness, modeling, model, polycrystalline materials, silica
AI 理解论文
溯源树
样例
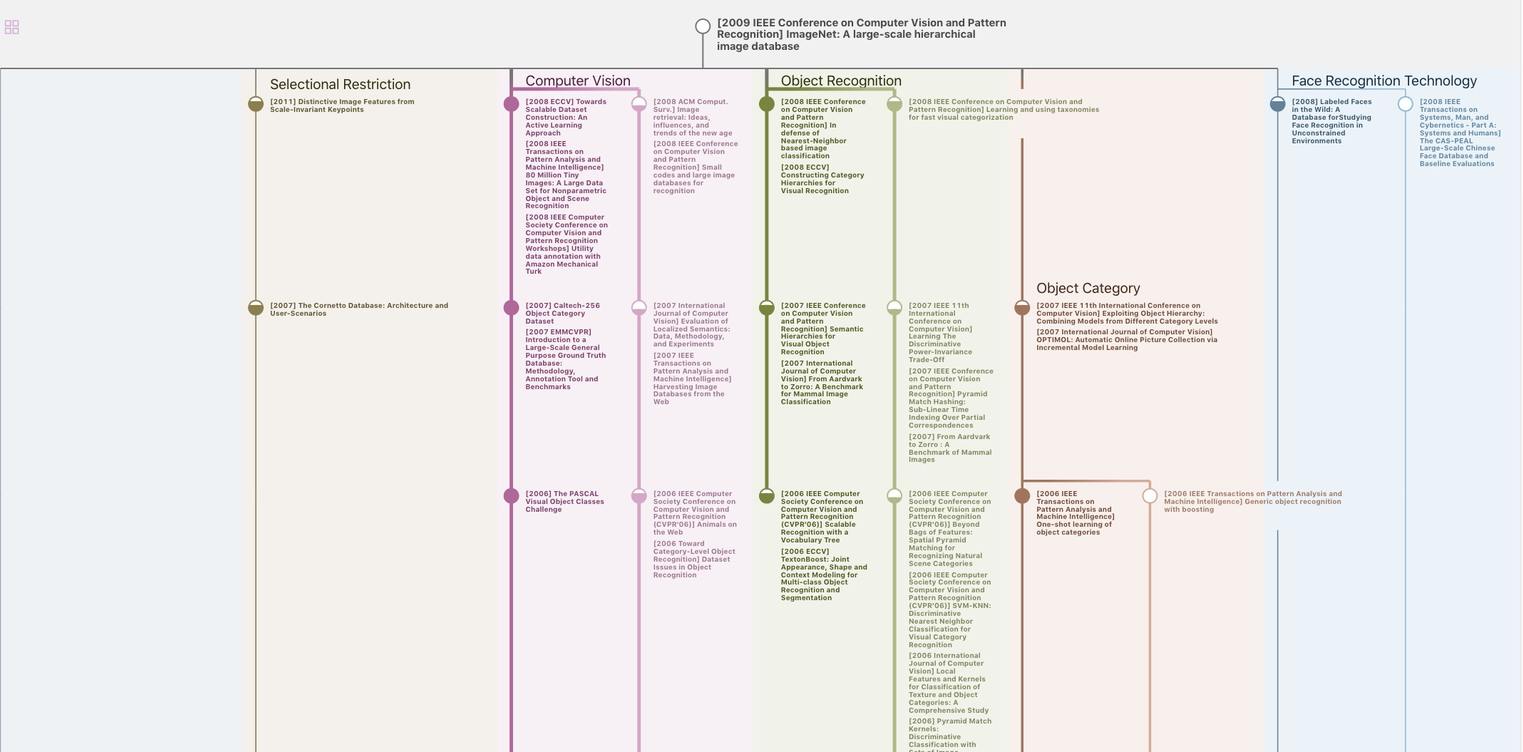
生成溯源树,研究论文发展脉络
Chat Paper
正在生成论文摘要