Prediction of methane adsorption isotherms in metal-organic frameworks by neural network synergistic with classical density functional theory
CHEMICAL ENGINEERING JOURNAL(2023)
摘要
A rapid method for predicting the unary gas adsorption isotherms of metal-organic frameworks (MOFs) is of great significance for the engineering design of both gas storage and gas separation. Here, we proposed a framework based on neural network (NN) synergistic with classical density functional theory (cDFT) that can be used to accurately predict methane adsorption isotherms of MOFs on a large scale. First, 17,644 candidate MOFs with orthorhombic unit cells were chosen from 324,426 hypothetical structures, and their methane adsorption isotherms were calculated by cDFT. Furthermore, we obtained the isotherm parameters for each MOF by fitting the isotherms with Langmuir equation. Second, the geometrical and chemical properties of all candidates were then calculated to construct the fingerprint descriptors of MOFs. These descriptors and isotherm parameters were used as inputs and targets, respectively, to train a deep neural network model. The trained neural network model can rapidly predict the isotherm parameters and further calculate the methane adsorption isotherms of the MOFs. During the model training, we proposed a physical constraint method embedded in the loss function to make the model better approximate the target isotherm parameters. Finally, we discussed several strategies to improve the prediction accuracy of the neural network.
更多查看译文
关键词
Metal–organic framework,Methane storage,Adsorption isotherm,Neural network,Classical density functional theory
AI 理解论文
溯源树
样例
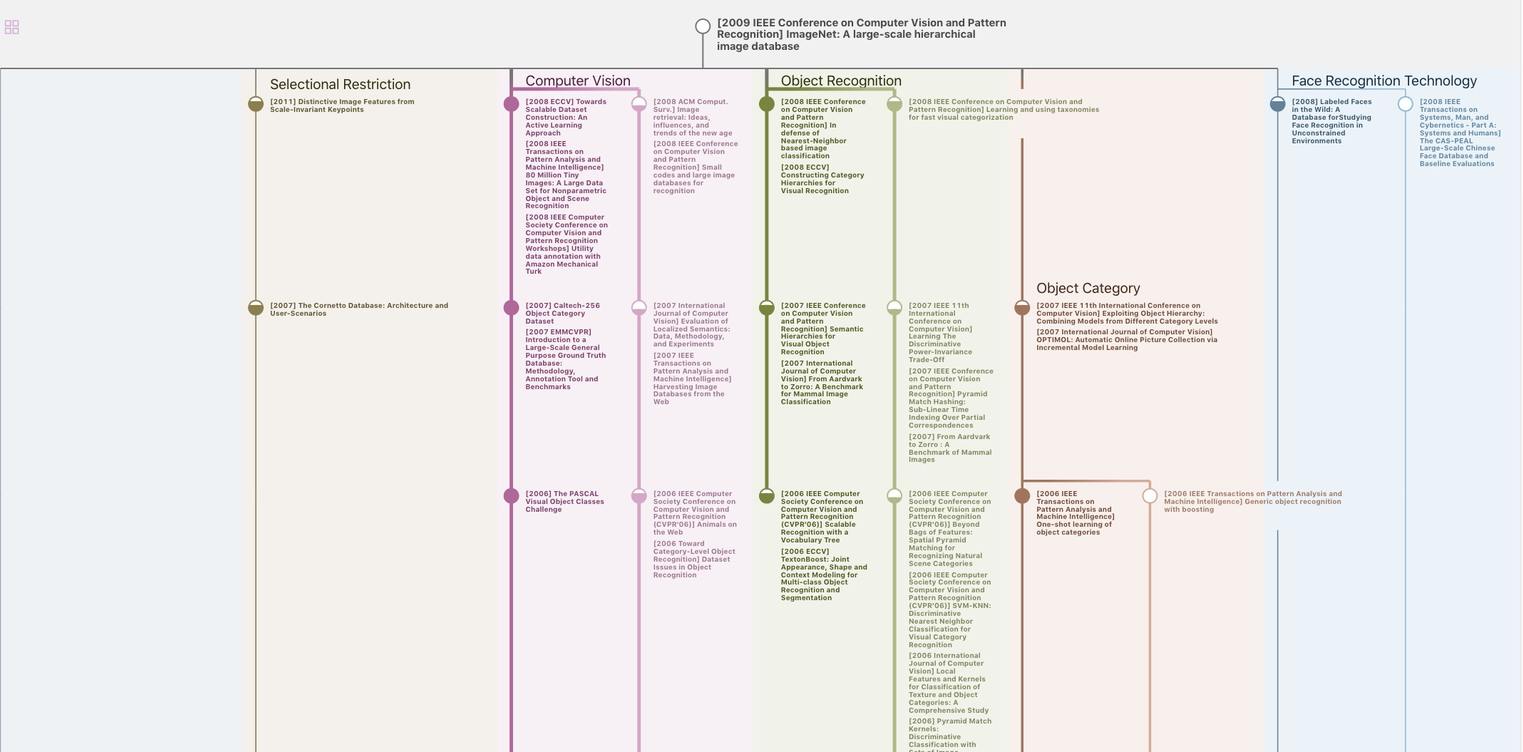
生成溯源树,研究论文发展脉络
Chat Paper
正在生成论文摘要