Improving explainability results of convolutional neural networks in microscopy images
NEURAL COMPUTING & APPLICATIONS(2023)
摘要
Explaining the predictions of neural networks to comprehend which region of an image influences the most its decision has become an imperative prerequisite when classifying medical images. In the case of convolutional neural networks, gradient-weighted class activation mapping is an explainability scheme that is more than often utilized for the unveiling of connections between stimuli and predictions especially in classification tasks that address the determination of the class between distinct objects in an image. However, certain categories of medical imaging such as confocal and histopathology images contain rich and dense information that differs from the cat versus dog paradigm. To further improve the performance of the gradient-weighted class activation mapping technique and the generated visualizations, we propose a segmentation-based explainability scheme that focuses on the common visual characteristics of each segment in an image to provide enhanced visualizations instead of highlighting rectangular regions. The explainability performance was quantified by applying random noise perturbations on microscopy images. The area over perturbation curve is utilized to demonstrate the improvement of the proposed methodology when utilizing the Slic superpixel algorithm against the Grad-CAM technique by an average of 4% for the confocal dataset and 9% for histopathology dataset. The results show that the generated visualizations are more comprehensible to humans than the initial heatmaps and demonstrate improved performance against the original Grad-CAM technique.
更多查看译文
关键词
convolutional neural networks,explainability results,neural networks,images
AI 理解论文
溯源树
样例
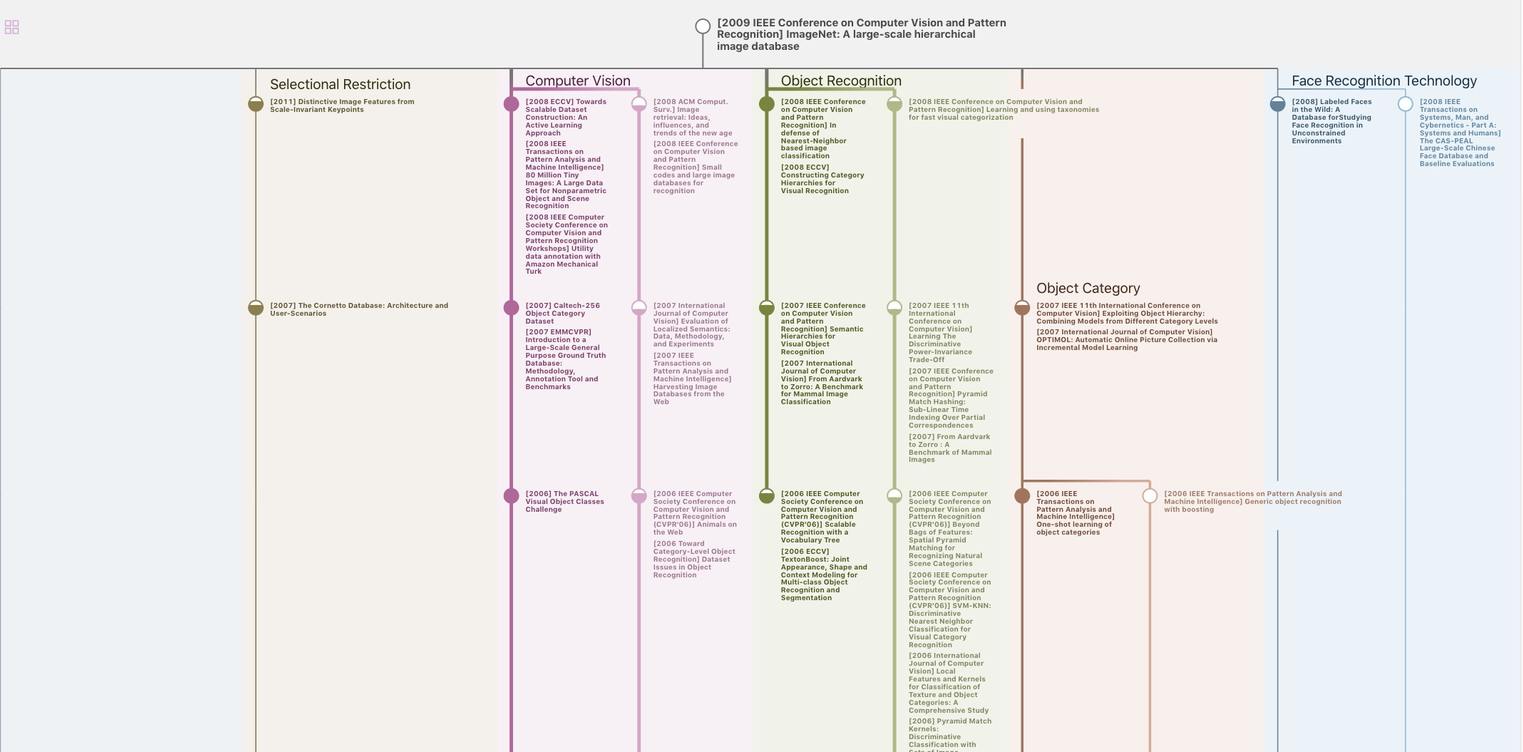
生成溯源树,研究论文发展脉络
Chat Paper
正在生成论文摘要