Importance-aware contrastive learning via semantically augmented instances for unsupervised sentence embeddings
INTERNATIONAL JOURNAL OF MACHINE LEARNING AND CYBERNETICS(2023)
摘要
Attaining better sentence embeddings benefits a wide range of natural language processing tasks. SimCSE applied a simple contrastive learning framework to train BERT models and achieved excellent sentence embeddings. Based on SimCSE, this paper proposes Importance-aware contrastive learning via semantically augmented instances for Unsupervised Sentence Embeddings (IconUSE), further improving the performance of sentence embeddings. IconUSE includes three optimizations. Firstly IconUSE applies a snippet affixation operation to modify the original sentence as its augmented version and then passes them into the pre-trained model respectively to get the positive pair. Then since hard negative instances that are similar to the anchor instance are more helpful for contrastive learning, IconUSE mixes the embeddings of the anchor instance and its negative instances to generate its virtual hard negative instances. In addition, classic contrastive learning treats all anchor instances with the same importance though some are already well represented, then IconUSE uses a modulating factor to apply different weights to different anchor instances. Experimental results suggest that our proposed IconUSE outperforms unsupervised SimCSE by + 1.35% Spearman’s correlation scores on semantic textual similarity tasks.
更多查看译文
关键词
Unsupervised sentence embeddings,Contrastive learning,Data augmentation,Importance-aware
AI 理解论文
溯源树
样例
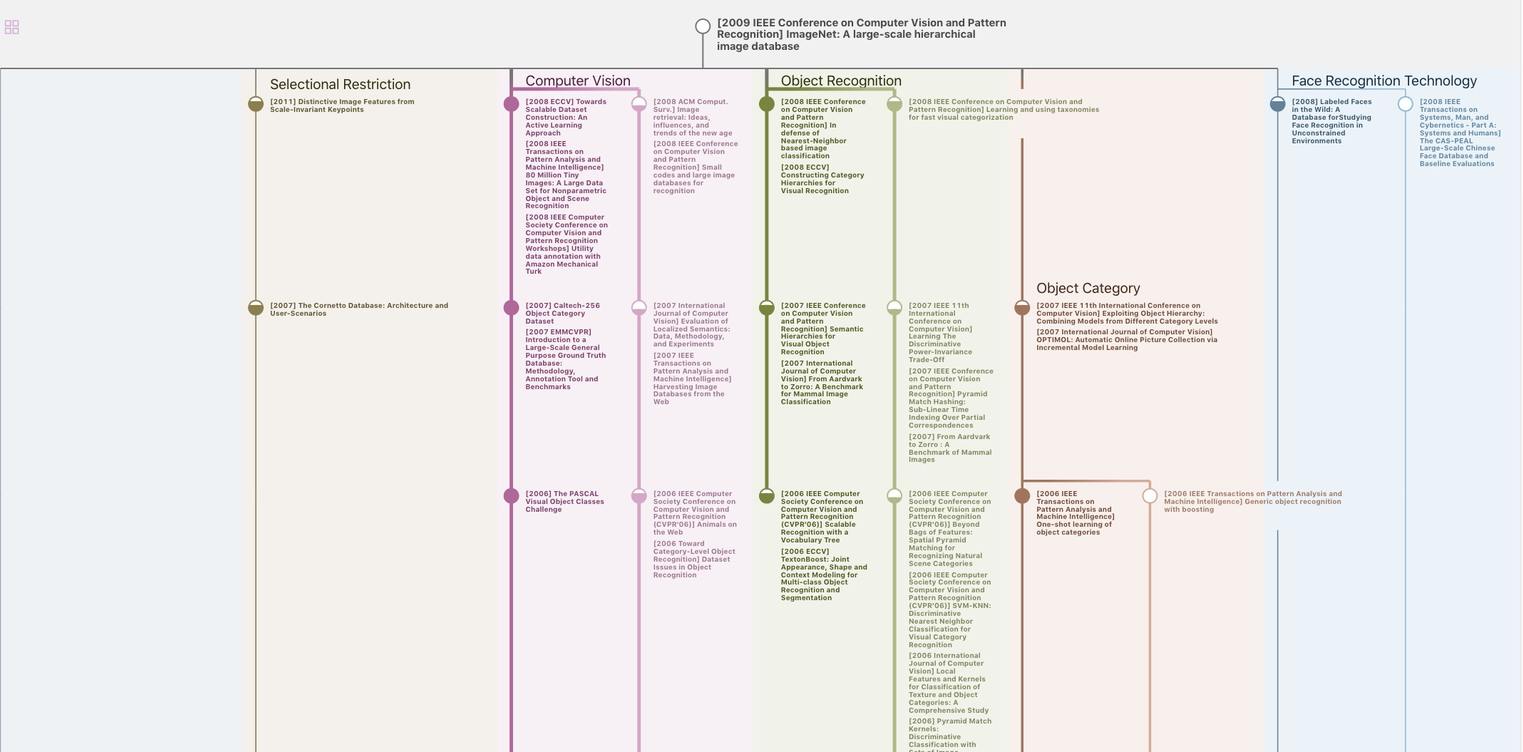
生成溯源树,研究论文发展脉络
Chat Paper
正在生成论文摘要