ADHD classification combining biomarker detection with attention auto-encoding neural network
BIOMEDICAL SIGNAL PROCESSING AND CONTROL(2023)
摘要
Attention Deficit Hyperactivity Disorder (ADHD) is one of most prevalent neurodevelopmental disorders in children. In decades, various neurobiological diagnosis methods have been well developed, yielding ADHD classification accuracy significantly improved. To our knowledge, deep-learning-based approaches attract more interest nowadays, since they effectively learn the potential features indicating ADHD disease. However, these learned features cannot directly project to certain biomarkers that have neurobiological meanings, which greatly hinders the neurobiological findings on ADHD. To ameliorate this problem, we proposed an attention auto-encoding network (Att-AENet) under an existing binary hypothesis testing (BHT) framework. By using brain functional connectivities (FCs) as input materials, an attention mechanism was employed to calculate the weights of FCs and measure the FC significance on ADHD. Therefore, the FC biomarkers of ADHD were conveniently identified from these weights. In detail, we built two attention subnetworks with dense and two-dimension convolution structures, respectively, and then evaluated the effect of these subnetworks from the viewpoint of ADHD classification. Sequentially, we took the biomarker detection analysis on the proposed dense-based attention subnetwork for its higher classification accuracy. On the test of ADHD-200 database, it shows the Att-AENet achieves a remarkable classification result with the average accuracy of 98.9%, especially 99.9% on some large-size datasets. More importantly, the FC biomarkers, corresponding to the discriminative weights learned by our attention subnetwork, are in accord with existing reported results. It demonstrates the reliability of these biomarkers. As a result, the proposed Att-AENet well fulfills the task of ADHD biomarker detection.
更多查看译文
关键词
ADHD classification, Attention mechanism, Auto-encoding neural network, Binary hypothesis testing, Functional connectivity
AI 理解论文
溯源树
样例
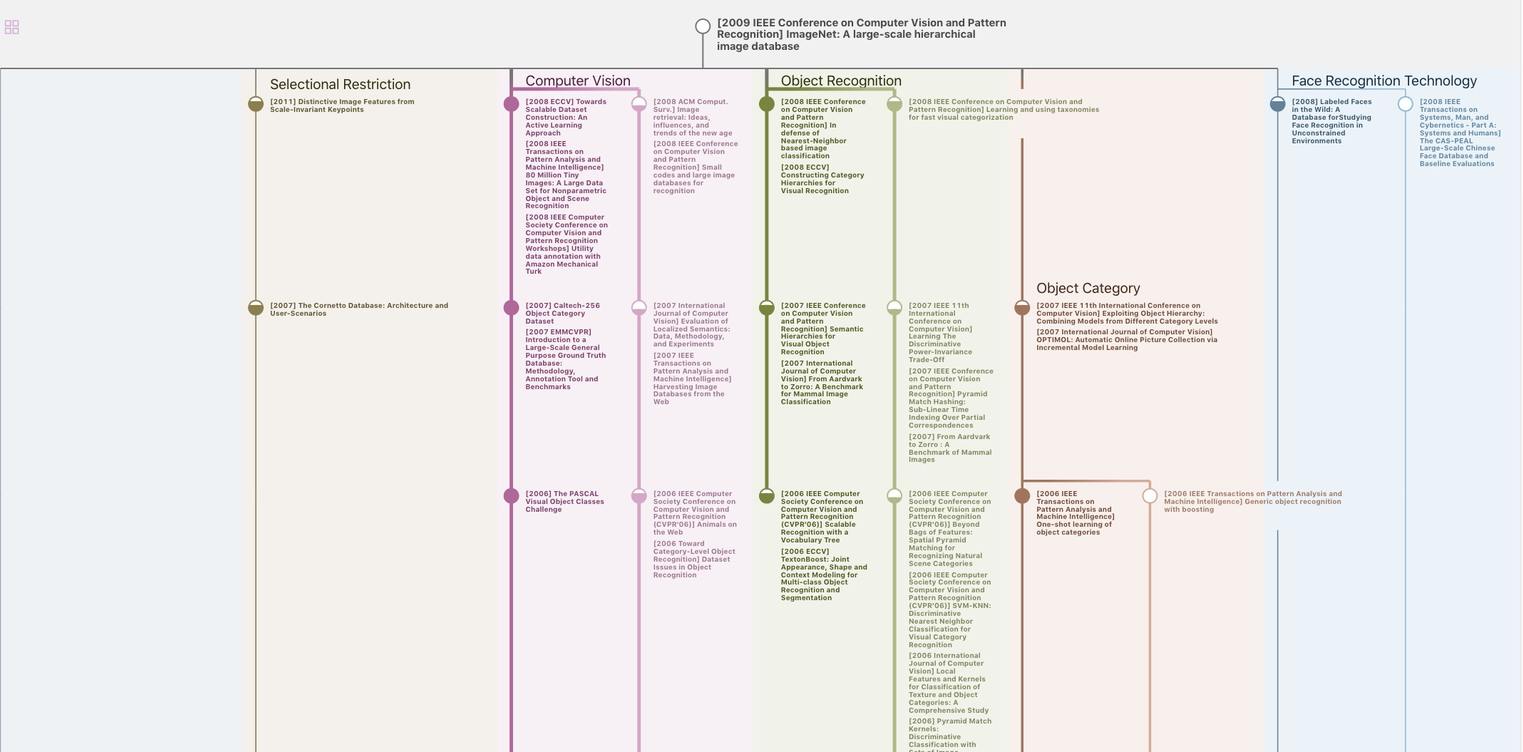
生成溯源树,研究论文发展脉络
Chat Paper
正在生成论文摘要