A new intelligent fault diagnosis framework for rotating machinery based on deep transfer reinforcement learning
CONTROL ENGINEERING PRACTICE(2023)
摘要
The advancement of artificial intelligence algorithms has gained growing interest in identifying the fault types in rotary machines, which is a high-efficiency but not a human-like module. Hence, in order to build a human-like fault identification module that could learn knowledge from the environment, in this paper, a deep reinforcement learning framework is proposed to provide an end-to-end training mode and a human-like learning process based on an improved Double Deep Q Network. In addition, to improve the convergence properties of the Deep Reinforcement Learning algorithm, the parameters of the former layers of the convolutional neural networks are transferred from a convolutional auto-encoder under an unsupervised learning process. The experiment results show that the proposed framework could efficiently extract the fault features from raw time-domain data and have higher accuracy than other deep learning models with balanced samples and better performance with imbalanced samples.
更多查看译文
关键词
Deep reinforcement learning, Convolutional auto-encoder, Fault diagnosis, Double deep Q network, Transfer learning
AI 理解论文
溯源树
样例
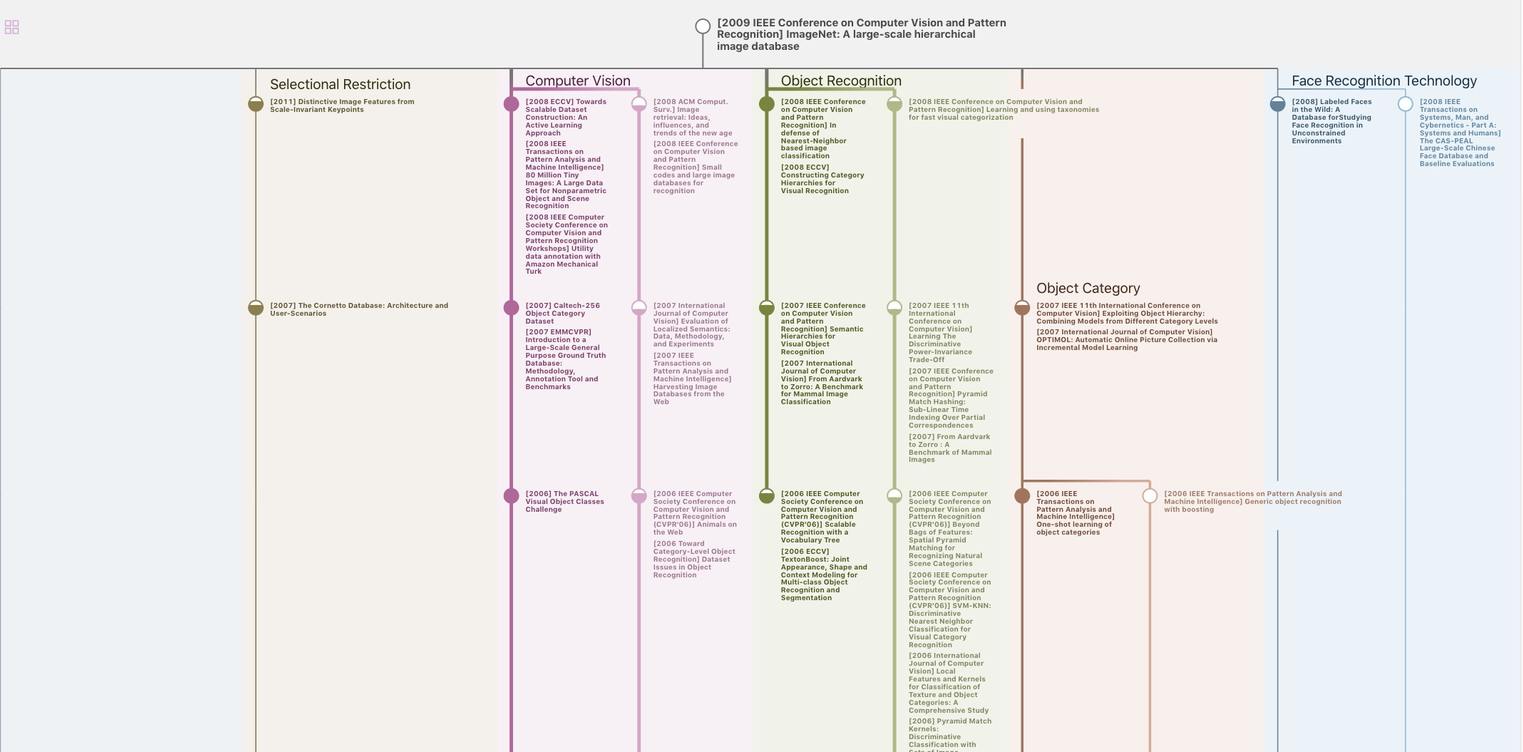
生成溯源树,研究论文发展脉络
Chat Paper
正在生成论文摘要