SDGAN: A novel spatial deformable generative adversarial network for low-dose CT image reconstruction
DISPLAYS(2023)
摘要
X-ray computed tomography (CT) is one of the most commonly used medical imaging technologies for the evaluation of many diseases. Full-dose imaging for CT ensures the image quality but usually raises concerns about the potential health risks of radiation exposure, especially for cancer patients. The conflict between reducing the radiation exposure and remaining diagnostic performance can be addressed effectively via recon-structing the low-dose CT (L-CT) to the high-quality full-dose CT (F-CT) ones. In this paper, we propose a Spatial Deformable Generative Adversarial Network (SDGAN) to achieve efficient L-CT reconstruction and analysis. SDGAN consists of three modules: the conformer-based generator, the dual-scale discriminator and the spatial deformable fusion module (SDFM). A sequence of consecutive L-CT slices is first fed into the conformer-based generator with the dual-scale discriminator to generate F-CT images. These estimated F-CT images are then fed into SDFM, which fully explores the inter-and intra-slice spatial information, to synthesize the final F-CT images with high quality. Experimental results show that the proposed SDGAN achieves the state-of-the-art performances on commonly used metrics and satisfies the reconstruction needs for clinical standards.
更多查看译文
关键词
ct,low-dose
AI 理解论文
溯源树
样例
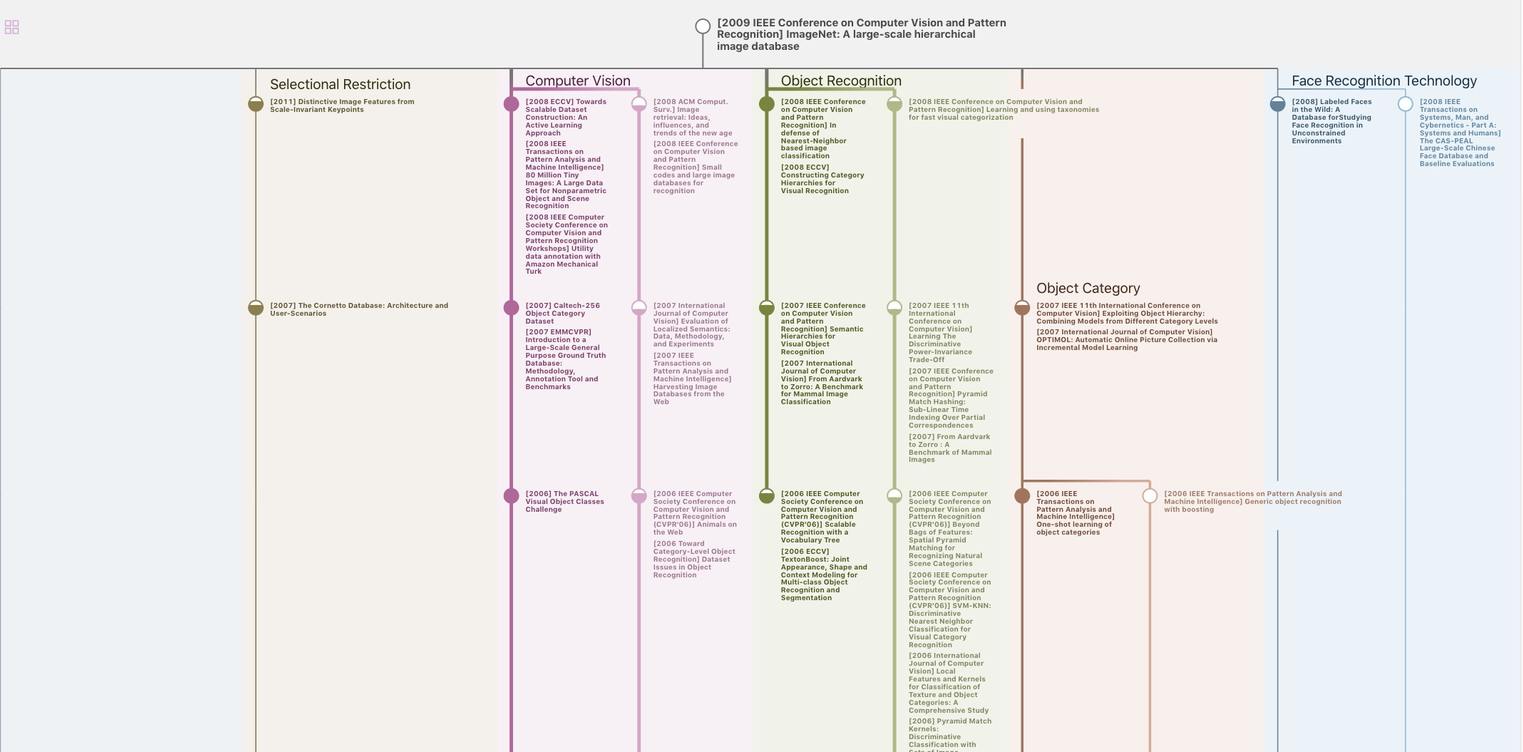
生成溯源树,研究论文发展脉络
Chat Paper
正在生成论文摘要