CIFair: Constructing continuous domains of invariant features for image fair classifications
KNOWLEDGE-BASED SYSTEMS(2023)
摘要
With learning models widely deployed in daily life, researchers are discovering that many of them generate discriminatory predictions towards sensitive attributes such as gender or race. A common way of tackling this problem is to learn fair features without sensitive information by removing features. This method requires extra heads for attribute predictions and eliminates the information via adversarial settings. Although the processes can impose fairness, they reduce the accuracy of the models relative to the originals. In this research, we generate continuous domains containing information from different subgroups with mixup operations. We then learn domain invariant features with similarity constraints. Different from previous methods, the proposed method, CIFair, can learn fair features without feature removal operations or task-irrelevant learning objectives. Finally, we evaluated our approach on the CelebA dataset with different sensitive attributes under multiple settings. All experimental results demonstrate that CIFair is able to impose enforce better fair prediction results than previous methods while maintaining model accuracy performance. (c) 2023 Elsevier B.V. All rights reserved.
更多查看译文
关键词
Fairness,Deep learning,Fair representation learning,Domain adaptation
AI 理解论文
溯源树
样例
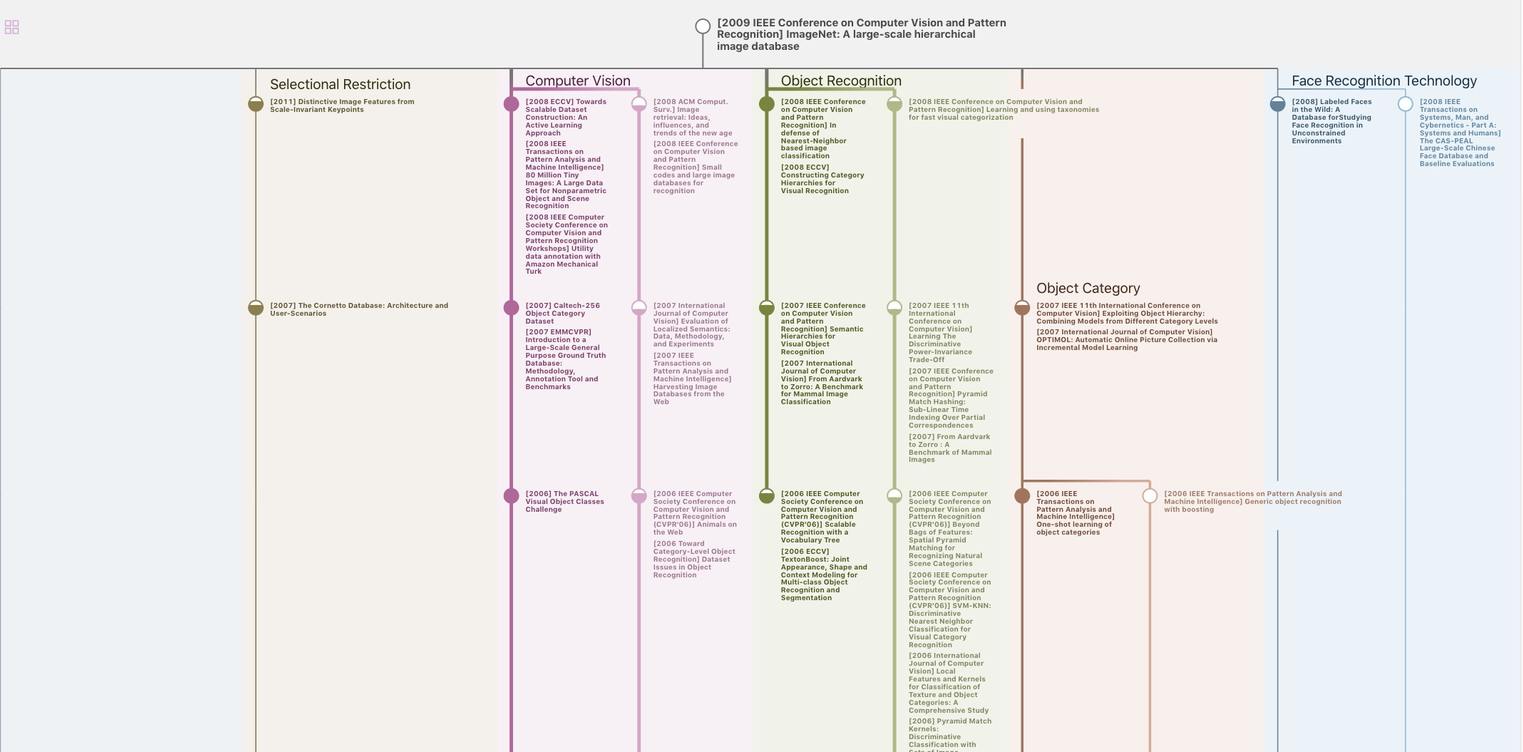
生成溯源树,研究论文发展脉络
Chat Paper
正在生成论文摘要