DC-DC circuit fault diagnosis based on GWO optimization of 1DCNN-GRU network hyperparameters
ENERGY REPORTS(2023)
摘要
Aiming at the problem that traditional machine learning methods rely on manual feature extraction in DC-DC circuit soft faults, it is important to effectively obtain the fault characteristics of DC-DC circuit soft faults. In this study, combining the advantages of one-dimensional convolutional neural network (1DCNN) and gated logic unit (GRU), a deep learning model for fault identification of time series signals is proposed to realize soft fault diagnosis of DC-DC circuits. In this study, a 1DCNN-GRU network model is constructed, and 1DCNN can directly perform automatic feature extraction on the data, while the GRU makes up for the shortcomings of CNN in processing time series data, thereby ensuring the comprehensiveness of the extracted features. For the hyperparameter problem in the network model, the powerful optimization ability of the gray wolf optimization algorithm is used to automatically search for the best hyperparameters in the 1DCNN-GRU network, and then the optimized 1DCNN-GRU network model is used for comprehensive feature learning. In order to meet the needs of deep learning for large data samples, the overlapping sampling method is used to enrich the data sample set. Experimental results show that the proposed method achieves 99.62% accuracy in DC-DC circuit fault diagnosis, and still maintains good robustness in noisy environment. (c) 2023 The Authors. Published by Elsevier Ltd. This is an open access article under the CC BY-NC-ND license (http://creativecommons.org/licenses/by-nc-nd/4.0/).
更多查看译文
关键词
Network hyperparameters,Gray wolf optimization algorithm,1DCNN-GRU,Circuit fault diagnosis
AI 理解论文
溯源树
样例
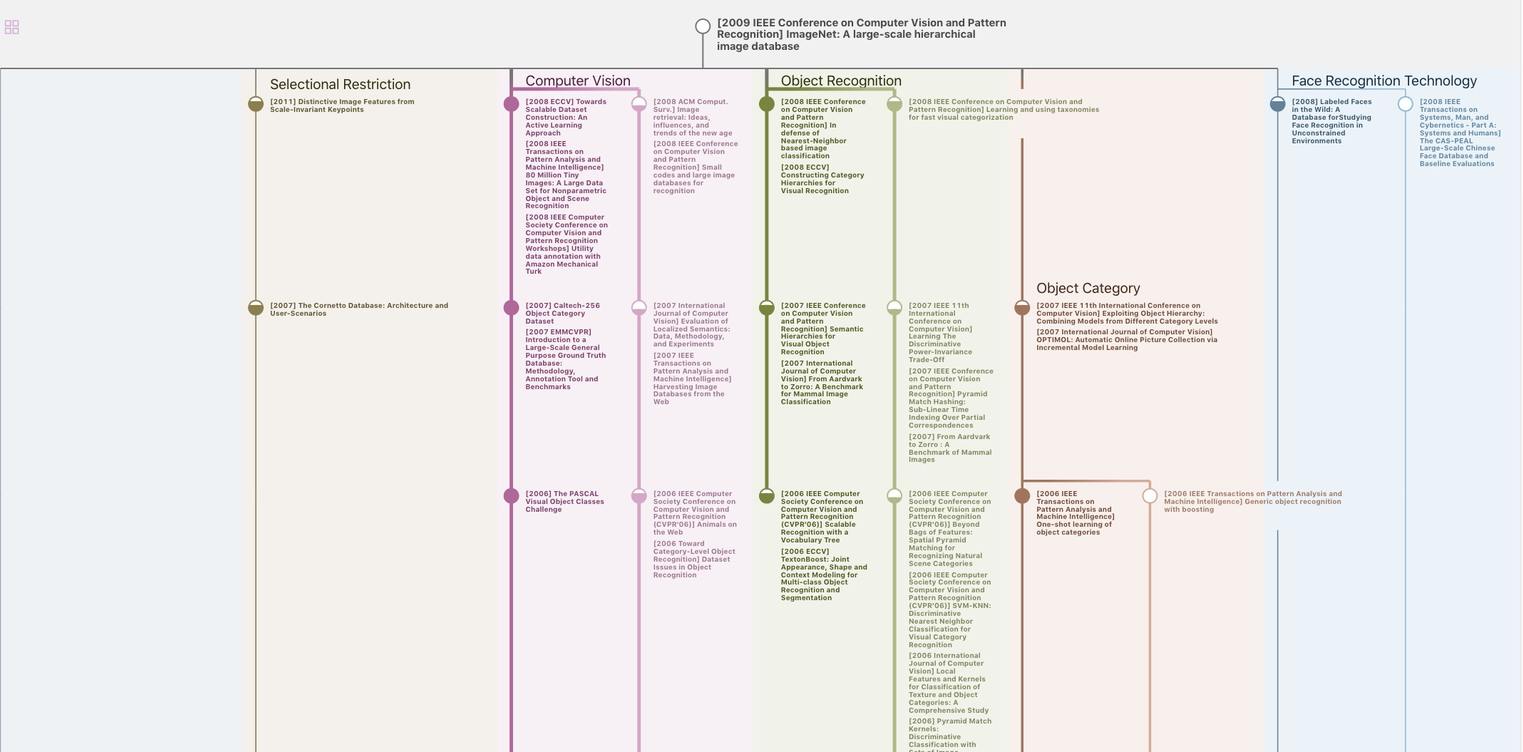
生成溯源树,研究论文发展脉络
Chat Paper
正在生成论文摘要