Pre-trained Language Model-based Retrieval and Ranking forWeb Search
ACM TRANSACTIONS ON THE WEB(2023)
摘要
Pre-trained language representation models (PLMs) such as BERT and Enhanced Representation through kNowledge IntEgration (ERNIE) have been integral to achieving recent improvements on various downstream tasks, including information retrieval. However, it is nontrivial to directly utilize these models for the large-scale web search due to the following challenging issues: (1) the prohibitively expensive computations ofmassive neural PLMs, especially for long texts in the web document, prohibit their deployments in the web search system that demands extremely low latency; (2) the discrepancy between existing task-agnostic pre-training objectives and the ad hoc retrieval scenarios that demand comprehensive relevance modeling is another main barrier for improving the online retrieval and ranking effectiveness; and (3) to create a significant impact on real-world applications, it also calls for practical solutions to seamlessly interweave the resultant PLM and other components into a cooperative system to serve web-scale data. Accordingly, we contribute a series of successfully applied techniques in tackling these exposed issues in this work when deploying the state-of-the-art Chinese pre-trained language model, i.e., ERNIE, in the online search engine system. We first present novel practices to perform expressive PLM-based semantic retrieval with a flexible poly-interaction scheme and cost-efficiently contextualize and rank web documents with a cheap yet powerful Pyramid-ERNIE architecture. We then endow innovative pre-training and fine-tuning paradigms to explicitly incentivize the query-document relevance modeling in PLM-based retrieval and ranking with the large-scale noisy and biased post-click behavioral data. We also introduce a series of effective strategies to seamlessly interwoven the designed PLM-based models with other conventional components into a cooperative system. Extensive offline and online experimental results show that our proposed techniques are crucial to achieving more effective search performance. We also provide a thorough analysis of our methodology and experimental results.
更多查看译文
关键词
Pre-trained language model,web retrieval,ranking
AI 理解论文
溯源树
样例
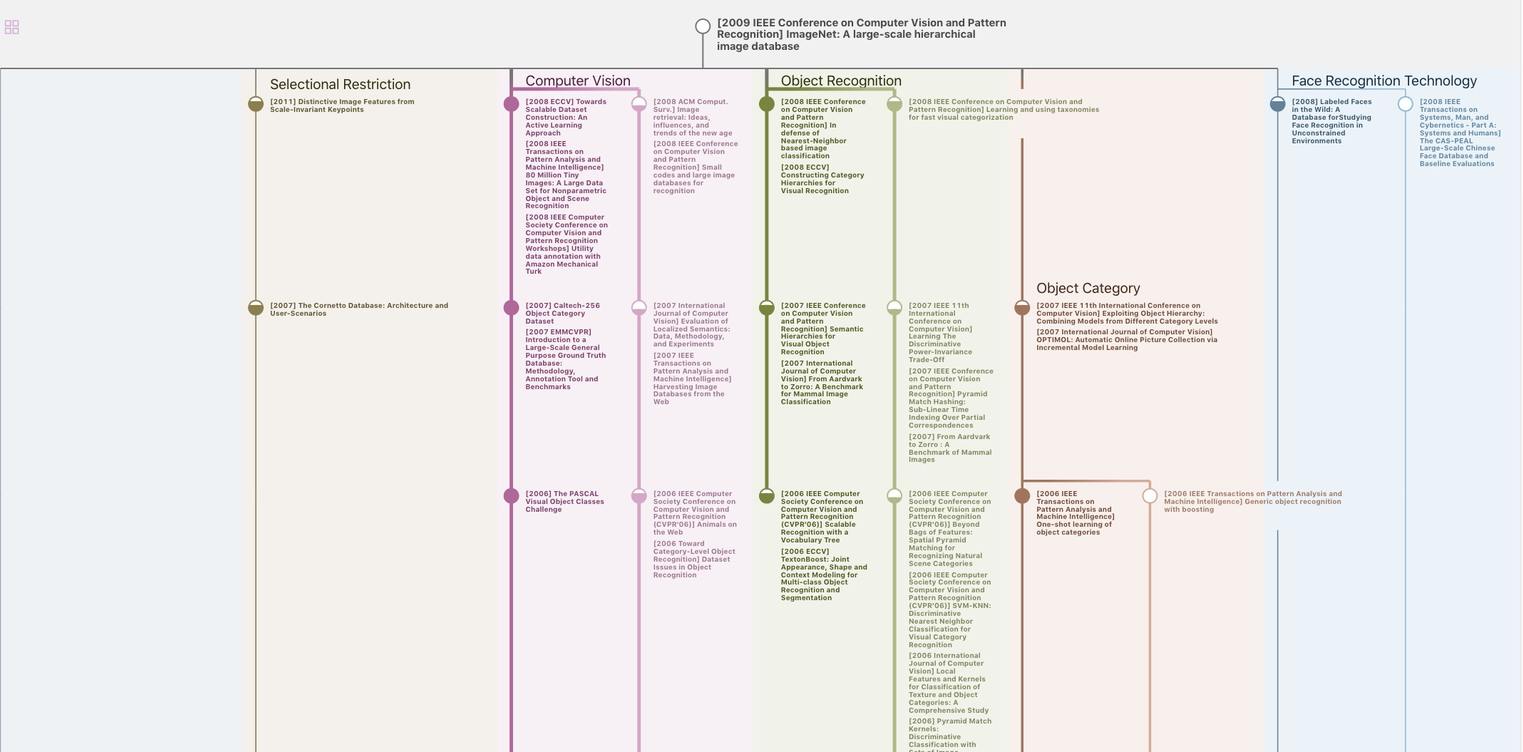
生成溯源树,研究论文发展脉络
Chat Paper
正在生成论文摘要