Two-Directional Simultaneous Inference for High-Dimensional Models
JOURNAL OF BUSINESS & ECONOMIC STATISTICS(2024)
摘要
This article proposes a general two-directional simultaneous inference (TOSI) framework for high-dimensional models with a manifest variable or latent variable structure, for example, high-dimensional mean models, high-dimensional sparse regression models, and high-dimensional latent factors models. TOSI performs simultaneous inference on a set of parameters from two directions, one to test whether the assumed zero parameters indeed are zeros and one to test whether exist zeros in the parameter set of nonzeros. As a result, we can better identify whether the parameters are zeros, thereby keeping the data structure fully and parsimoniously expressed. We theoretically prove that the single-split TOSI is asymptotically unbiased and the multi-split version of TOSI can control the Type I error below the prespecified significance level. Simulations are conducted to examine the performance of the proposed method in finite sample situations and two real datasets are analyzed. The results show that the TOSI method can provide more predictive and more interpretable estimators than existing methods.
更多查看译文
关键词
High-dimensional models,Interpretable factor model,Sparsity,Two-directional simultaneous inference
AI 理解论文
溯源树
样例
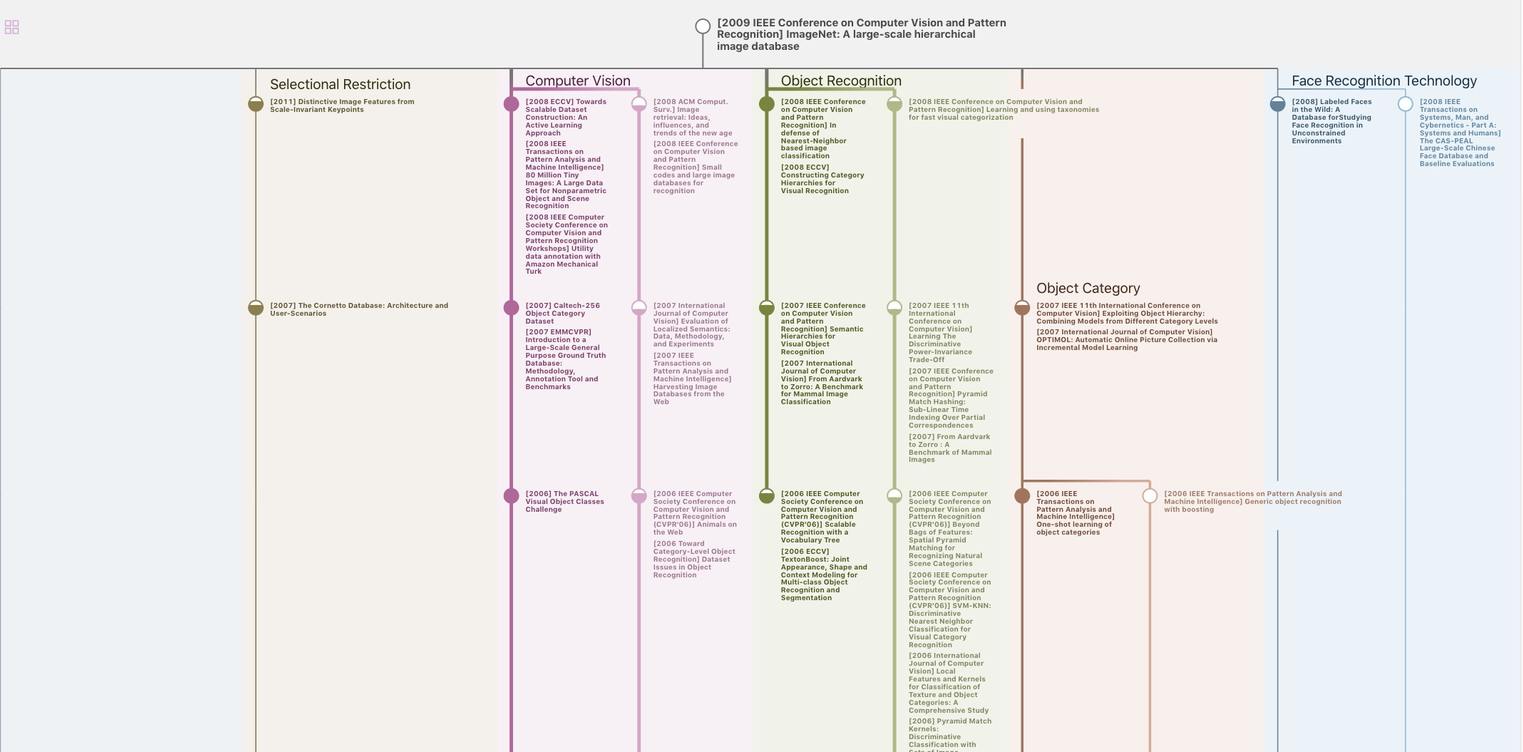
生成溯源树,研究论文发展脉络
Chat Paper
正在生成论文摘要